Enhancing automated inspection in metal industries: zero-shot segmentation of surface defects using bounding box prompts
Measurement Science and Technology(2024)
摘要
Abstract Surface defect detection poses a challenging problem that has been addressed for decades. Many of actual solutions are based on deep learning algorithms.
However, these algorithms require a large amount of data to train accurate models. This becomes especially problematic for semantic segmentation algorithms, which need labelled datasets at the pixel level, a laborious and time-consuming task. Therefore, this paper proposes a semi-supervised method in which the predictions of an object detector are combined with the segmentation of a zero-shot model, eliminating the need to label a dataset for semantic segmentation. Results are compared with relevant supervised semantic segmentation models, such as UNet and DeepLabv3+. UNet achieves an F1 score of 0.824, while DeepLabv3+ achieves 0.847. The proposed method combining YOLOv8n and Segment Anything Model (SAM) achieves an F1 score of 0.804. This slight loss of F1 is compensated by the significant time savings, unlike UNet and DeepLabv3+, where labelling of the dataset is necessary.
更多查看译文
AI 理解论文
溯源树
样例
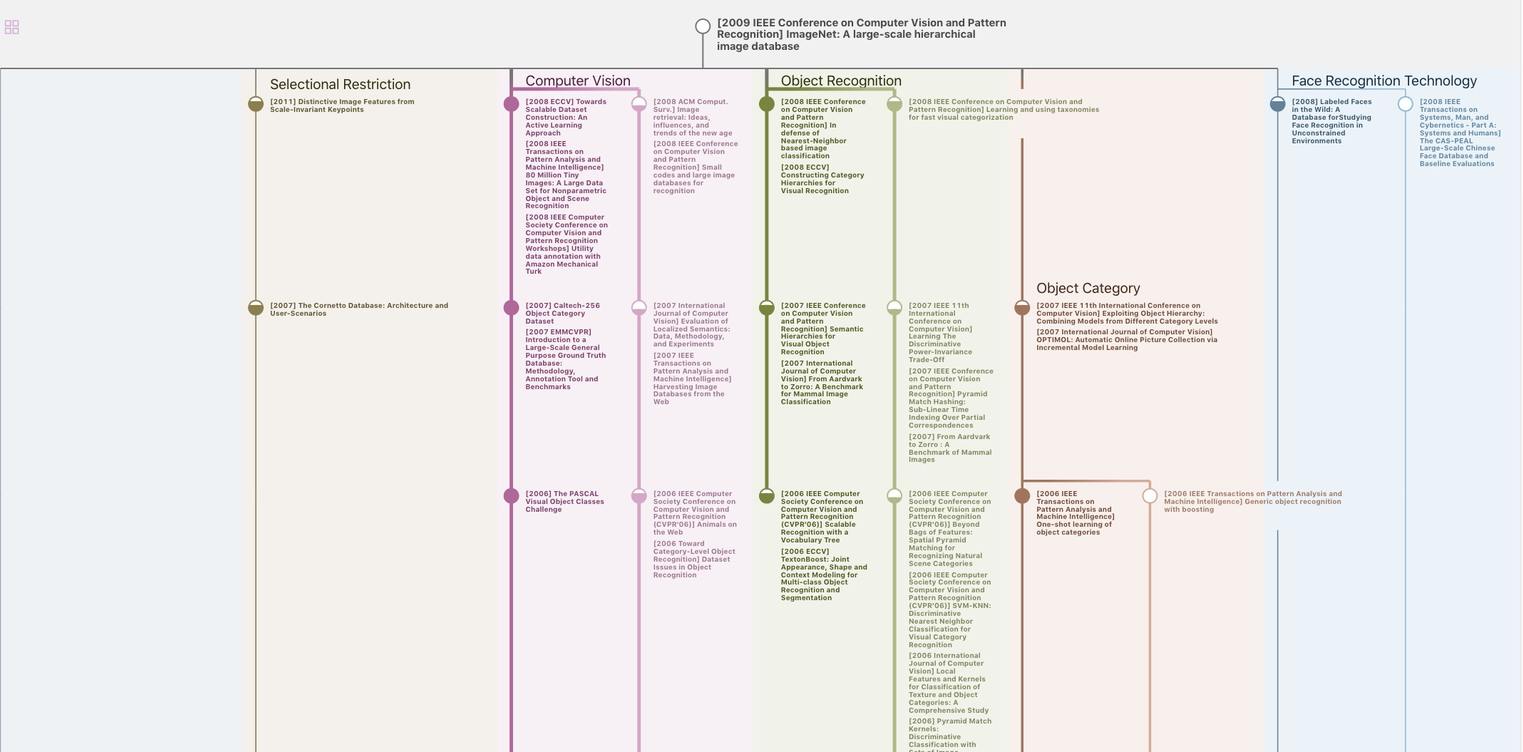
生成溯源树,研究论文发展脉络
Chat Paper
正在生成论文摘要