Joint Visibility Region Detection and Channel Estimation for XL-MIMO Systems via Alternating MAP
arxiv(2024)
摘要
We investigate a joint visibility region (VR) detection and channel
estimation problem in extremely large-scale multiple-input-multiple-output
(XL-MIMO) systems, where near-field propagation and spatial non-stationary
effects exist. In this case, each scatterer can only see a subset of antennas,
i.e., it has a certain VR over the antennas. Because of the spatial correlation
among adjacent sub-arrays, VR of scatterers exhibits a two-dimensional (2D)
clustered sparsity. We design a 2D Markov prior model to capture such a
structured sparsity. Based on this, a novel alternating maximum a posteriori
(MAP) framework is developed for high-accuracy VR detection and channel
estimation. The alternating MAP framework consists of three basic modules: a
channel estimation module, a VR detection module, and a grid update module.
Specifically, the first module is a low-complexity inverse-free variational
Bayesian inference (IF-VBI) algorithm that avoids the matrix inverse via
minimizing a relaxed Kullback-Leibler (KL) divergence. The second module is a
structured expectation propagation (EP) algorithm which has the ability to deal
with complicated prior information. And the third module refines polar-domain
grid parameters via gradient ascent. Simulations demonstrate the superiority of
the proposed algorithm in both VR detection and channel estimation.
更多查看译文
AI 理解论文
溯源树
样例
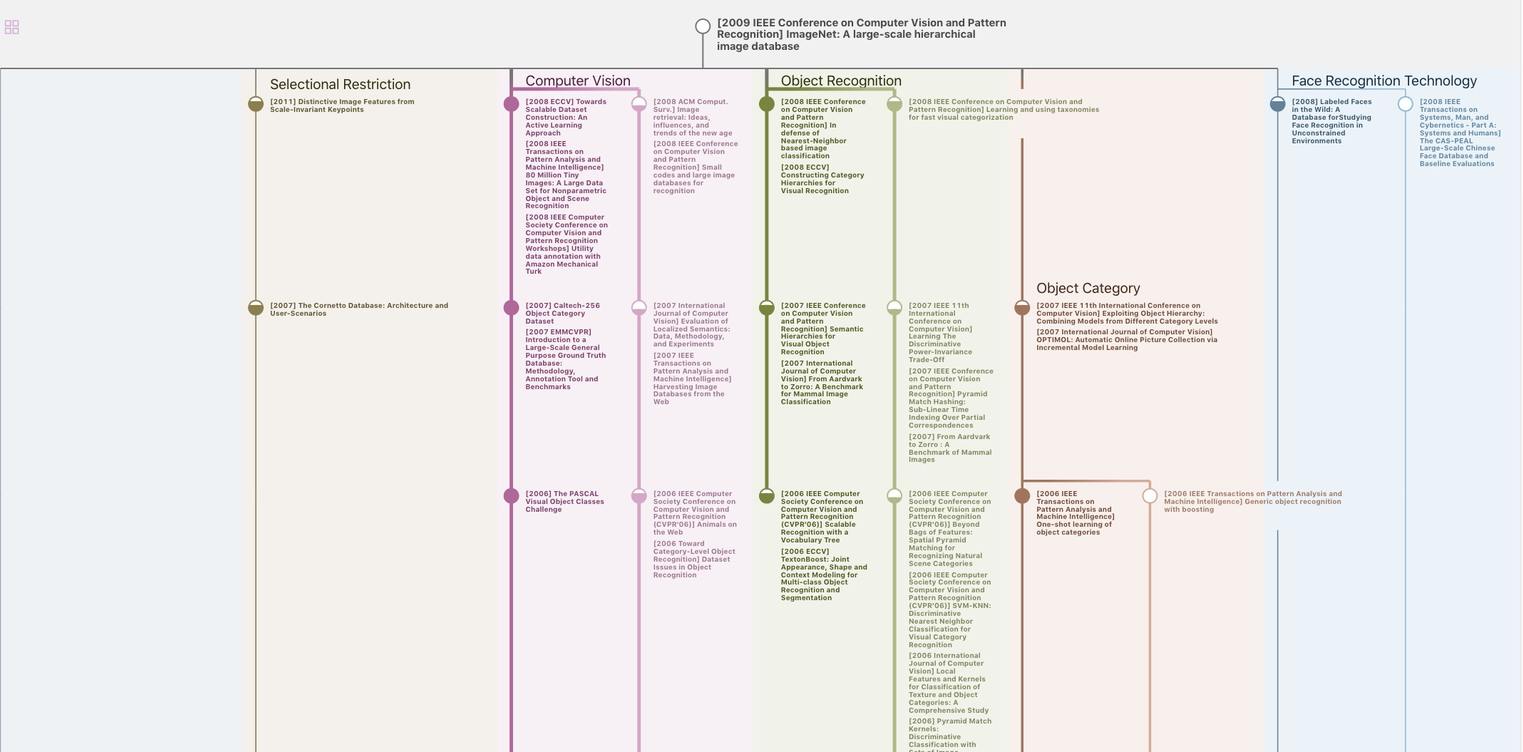
生成溯源树,研究论文发展脉络
Chat Paper
正在生成论文摘要