Few-Shot Class-Incremental Learning with Adjustable Pseudo-Incremental Sessions for Bearing Fault Diagnosis
IEEE Sensors Journal(2024)
摘要
Rotating machinery may constantly generate new classes of faults in complex operating environments, with a finite set of fault samples that are obtainable. The incremental nature of fault types and the scarcity of samples present great challenges for fault diagnosis models employing deep learning techniques, such as overfitting due to insufficient data and stability–plasticity problem when new fault samples are introduced. A few-shot class-incremental learning (FSCIL) method with adjustable pseudo-incremental sessions (APIS) is introduced in this study to overcome the abovementioned concerns. First, the method extracts fault samples from the sufficient data of the real base session, and it sets the pseudo FSCIL task according to the data format of FSCIL. The pseudo FSCIL task is employed to extract invariant features in the base and incremental session, and the feature space is trained to provide a reference model for the real fault incremental task. Second, the adjuster based on a self-attention mechanism is used to learn more discriminative features. Feature differences are observed between the old fault classifier and a fresh fault prototype. Based on the self-attention mechanism, the distinguishing features between the fault samples in the testing dataset and the old classifier are highlighted. In this way, a fault diagnosis model of the few-shot fault class increment with more generalization ability is acquired. A case study shows that APIS can effectively alleviate stability–plasticity and overfitting problems when dealing with FSCIL bearing fault diagnosis tasks.
更多查看译文
关键词
Bearing,fault diagnosis,class-incremental learning,few-shot learning,few-shot class-incremental learning
AI 理解论文
溯源树
样例
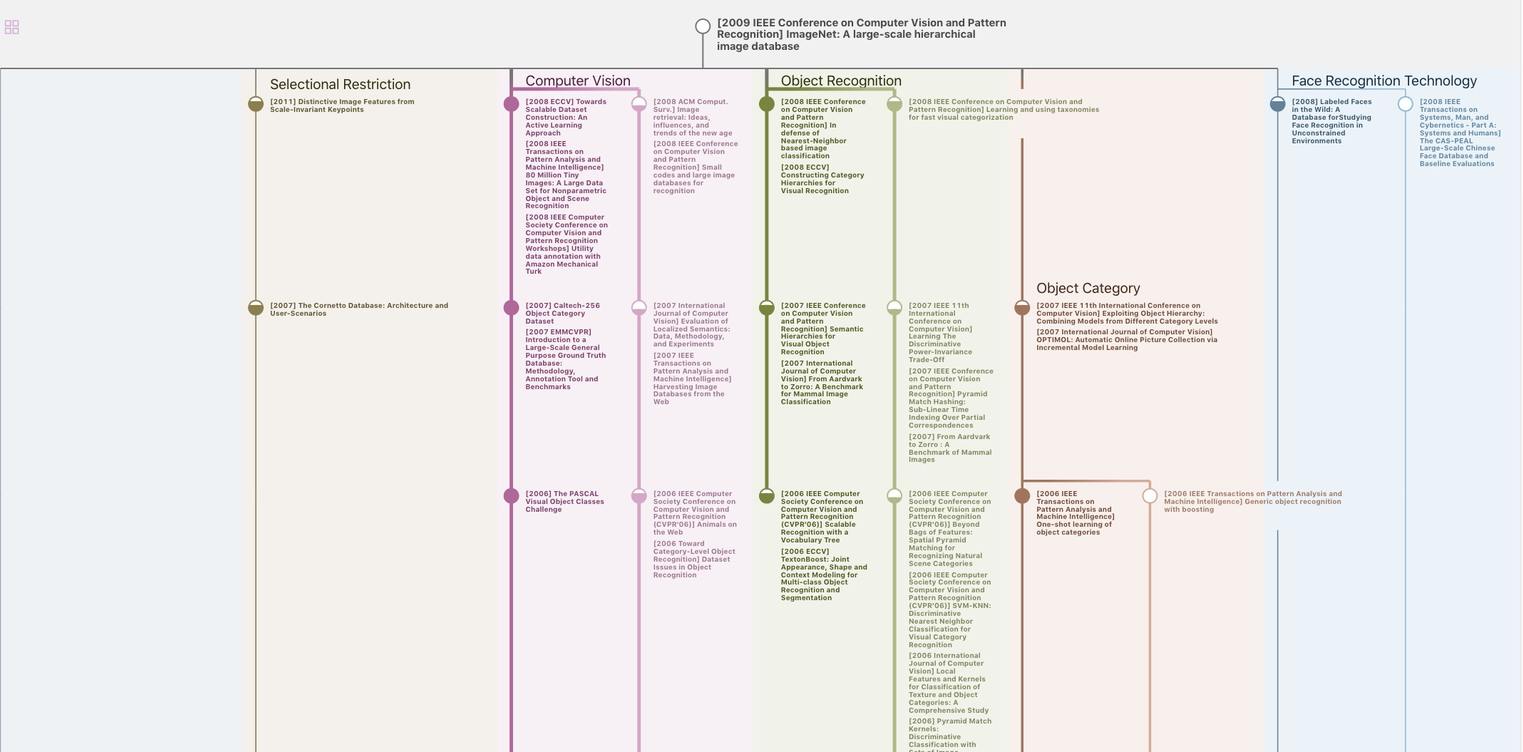
生成溯源树,研究论文发展脉络
Chat Paper
正在生成论文摘要