Enhancing Knowledge Reusability: A Distributed Multitask Machine Learning Approach
IEEE Transactions on Emerging Topics in Computing(2024)
摘要
In the era of the Internet of Things, the unprecedented growth of data surpasses current predictive analytics and processing capabilities. Due to the potential redundancy of similar data and analytics tasks, it is imperative to extract patterns from distributed data and predictive models so that existing schemes can be efficiently reused in distributed computing environments. This is expected to avoid building and maintaining reduplicative predictive models. The fundamental challenge, however, is the detection of reusable tasks and tuning models in order to improve predictive capacity while being reused. We introduce a two-phase Distributed Multi-task Machine Learning (DMtL) framework coping with this challenge. In the first phase, similar tasks are identified and efficiently grouped together according to locally trained models' performance meta-features, using Partial Learning Curves (PLC). In the subsequent phase, we leverage the PLC-driven DMtL paradigm to boost the performance of candidate reusable models per group of tasks in distributed computing environments. We provide a thorough analysis of our framework along with a comparative assessment against relevant approaches and prior work found in the respective literature. Our experimental results showcase the feasibility of the PLC-driven DMtL method in terms of adaptability and reusability of existing knowledge in distributed computing systems.
更多查看译文
关键词
Distributed Machine Learning,Knowledge Reuse,Knowledge Management,Multitask Learning
AI 理解论文
溯源树
样例
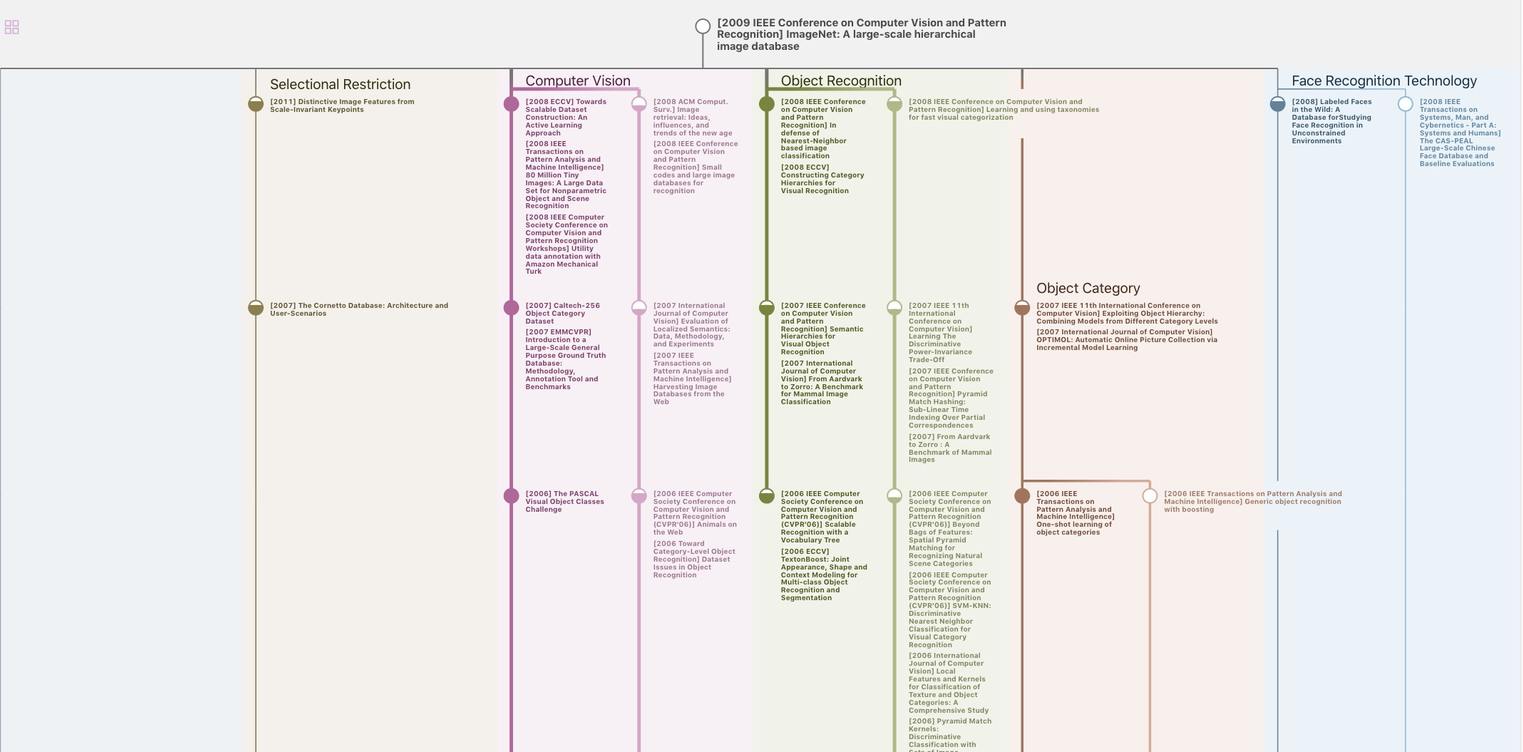
生成溯源树,研究论文发展脉络
Chat Paper
正在生成论文摘要