Traffic Performance GPT (TP-GPT): Real-Time Data Informed Intelligent ChatBot for Transportation Surveillance and Management
arxiv(2024)
摘要
The digitization of traffic sensing infrastructure has significantly
accumulated an extensive traffic data warehouse, which presents unprecedented
challenges for transportation analytics. The complexities associated with
querying large-scale multi-table databases require specialized programming
expertise and labor-intensive development. Additionally, traditional analysis
methods have focused mainly on numerical data, often neglecting the semantic
aspects that could enhance interpretability and understanding. Furthermore,
real-time traffic data access is typically limited due to privacy concerns. To
bridge this gap, the integration of Large Language Models (LLMs) into the
domain of traffic management presents a transformative approach to addressing
the complexities and challenges inherent in modern transportation systems. This
paper proposes an intelligent online chatbot, TP-GPT, for efficient customized
transportation surveillance and management empowered by a large real-time
traffic database. The innovative framework leverages contextual and generative
intelligence of language models to generate accurate SQL queries and natural
language interpretations by employing transportation-specialized prompts,
Chain-of-Thought prompting, few-shot learning, multi-agent collaboration
strategy, and chat memory. Experimental study demonstrates that our approach
outperforms state-of-the-art baselines such as GPT-4 and PaLM 2 on a
challenging traffic-analysis benchmark TransQuery. TP-GPT would aid researchers
and practitioners in real-time transportation surveillance and management in a
privacy-preserving, equitable, and customizable manner.
更多查看译文
AI 理解论文
溯源树
样例
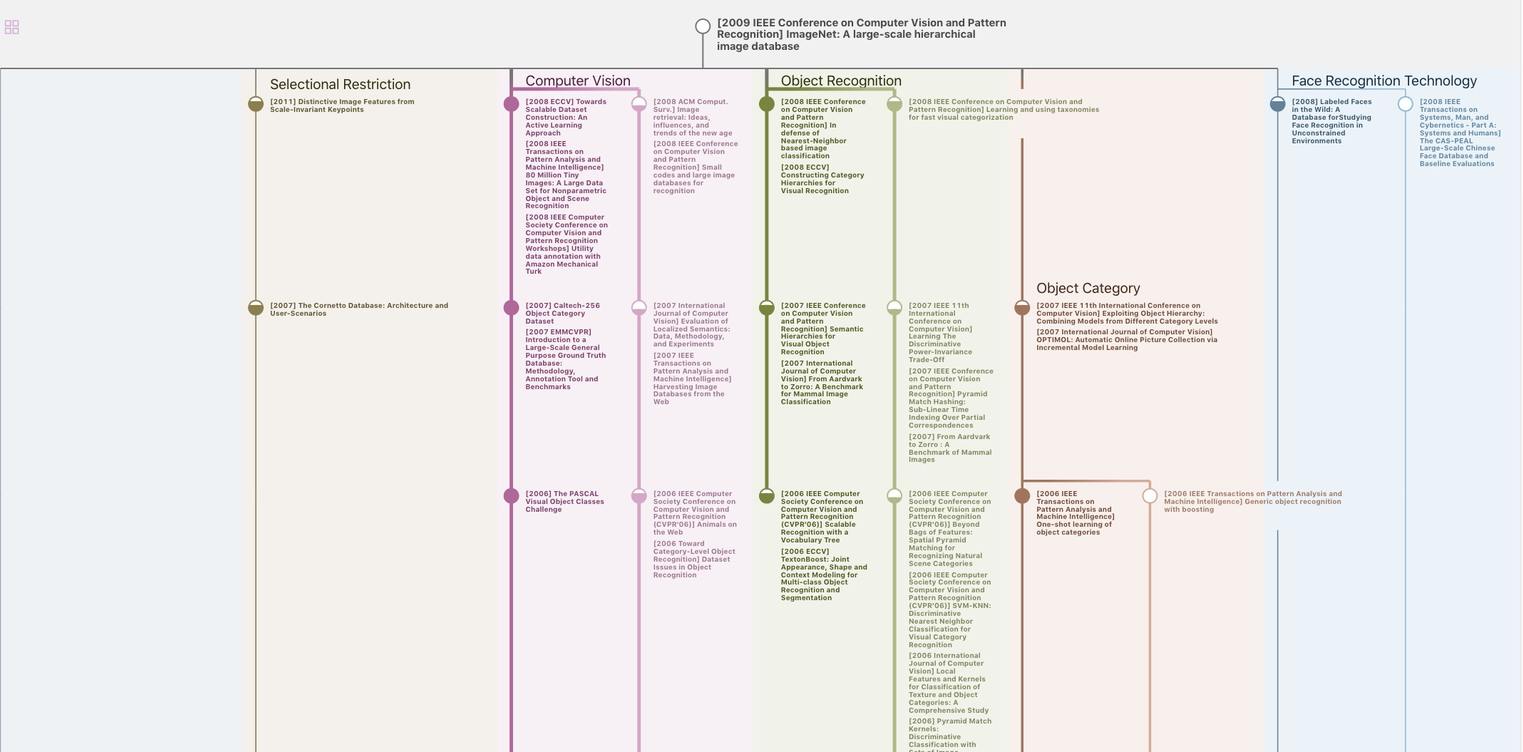
生成溯源树,研究论文发展脉络
Chat Paper
正在生成论文摘要