Validation of a Natural Language Processing Algorithm using National Reporting Data to Improve Identification of Anesthesia-related ADVerse evENTs: The “ADVENTURE” Study
Anaesthesia Critical Care & Pain Medicine(2024)
摘要
Background
Reporting and analysis of adverse events (AE) is associated with improved healthcare learning, quality outcomes, and patient safety. Manual text analysis is time-consuming, costly, and prone to human errors. We aimed to demonstrate the feasibility of machine learning and natural language processing (NLP) approaches for early predictions of adverse events and provide input to direct quality improvement and patient safety initiatives.
Methods
We used machine learning to analyze 9,559 continuously reported AE by clinicians and healthcare systems to the French National Health accreditor (HAS) between January 1, 2009, and December 31, 2020, for a total of 135,000 unique de-identified AE reports. We validated the labeling and determined the associations between different root causes and patient consequences. The model was validated by independent expert anesthesiologists.
Results
The machine learning and Artificial Intelligence (AI) model trained on 9,559 AE datasets accurately categorized 8800 (88%) of reported AE. The three most frequent AE types were “difficult orotracheal intubation” (16.9% of AE reports), “medication error” (10.5%), and “post-induction hypotension” (6.9%). The accuracy of the AI model reached 70.9% sensitivity, 96.6% specificity for “difficult intubation”, 43.2% sensitivity, and 98.9% specificity for “medication error.”
Conclusions
This unsupervised method provides an accurate, automated, AI-supported search algorithm that ranks and helps to understand complex patterns of risky patient situations and has greater speed, precision, and clarity when compared to manual human data extraction. Machine learning (ML) and natural language processing models can effectively be used to process natural language AE reports and augment expert clinician input. This model can support clinical applications and methodological standards of implementations and be used to better inform and enhance decision-making for improved risk management and patient safety.
Trial Registration
The study was approved by the ethics committee of the French Society of Anesthesiology (IRB 00010254-2020-20) and the CNIL (CNIL: 118 58 95) and the study was registered with ClinicalTrials.gov (NCT: NCT05185479).
更多查看译文
关键词
Adverse events,patient safety,Natural Language Processing,Artificial intelligence,Quality improvement
AI 理解论文
溯源树
样例
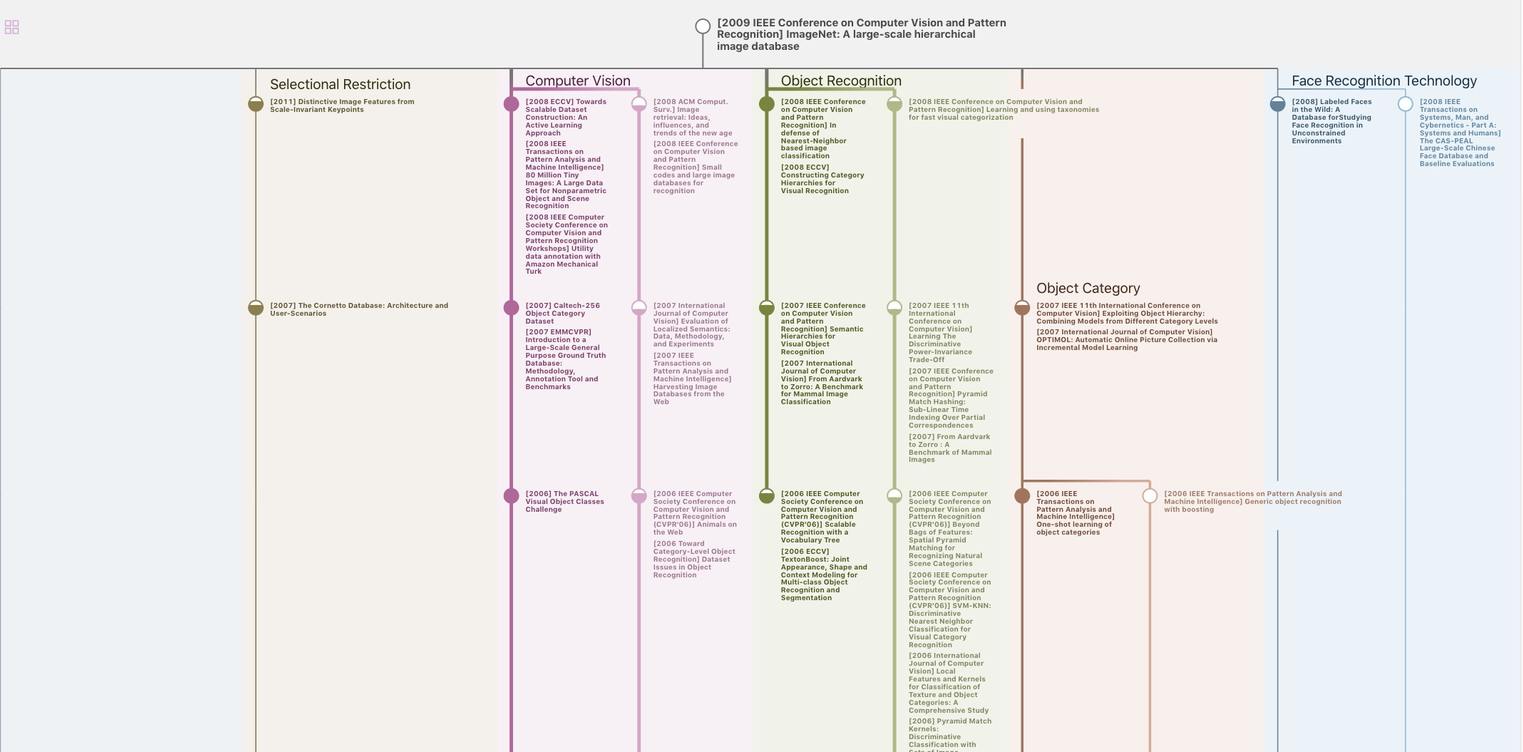
生成溯源树,研究论文发展脉络
Chat Paper
正在生成论文摘要