Intelligent Model Management: Using Reinforcement Learning to Detect Data Drift and Retrain Industrial Machine Learning Systems
2024 26th International Conference on Digital Signal Processing and its Applications (DSPA)(2024)
摘要
In the evolving landscape of industrial processes, maintaining the accuracy of machine learning (ML) models is a formidable challenge due to the phenomenon of data drift. This paper introduces an innovative reinforcement learning (RL) framework designed to monitor and adaptively retrain ML models in response to data drift, ensuring sustained model performance in industrial settings. We apply our methodology to a real-world scenario within the cement milling industry, demonstrating that our RL agent can effectively identify optimal retraining intervals and maintain high model accuracy over time. Our results indicate that ML models managed by the RL agent surpass the performance of static models, highlighting the potential of RL in continuous model management. The task-agnostic nature of our approach also suggests its applicability across various domains, paving the way for future research in adaptive systems and realtime data processing.
更多查看译文
关键词
Reinforcement Learning,Machine Learning,Model Retraining,Industrial Mill,Data Drift Detection,Adaptive Systems,Real-Time Data Processing,Statistical Data Analysis,Model Adaptation Techniques
AI 理解论文
溯源树
样例
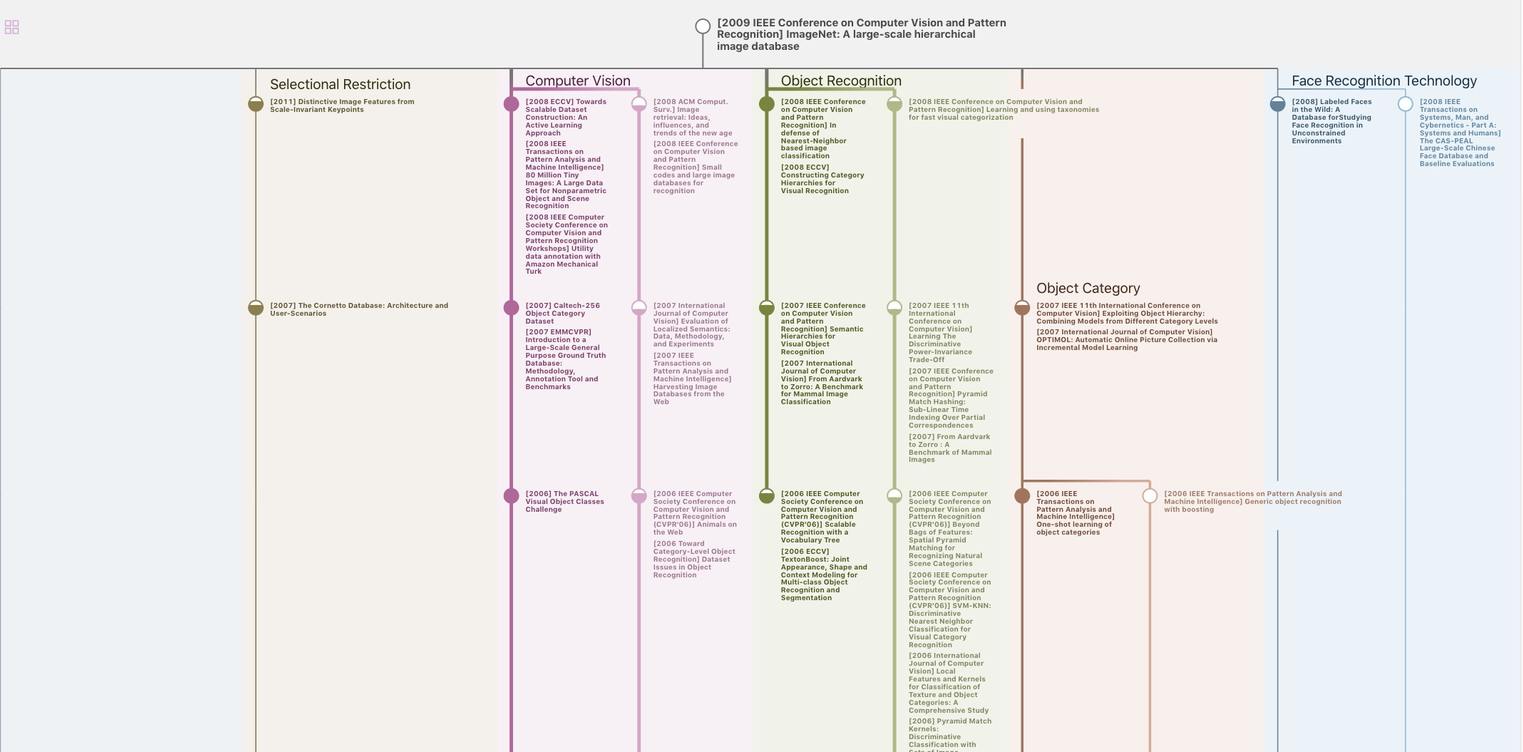
生成溯源树,研究论文发展脉络
Chat Paper
正在生成论文摘要