A review on nanofluid, phase change material and machine learning applications for thermal management of hydrogen storage in metal hydrides
International Journal of Hydrogen Energy(2024)
摘要
This review is generally about some significant applications related to thermal management of hydrogen storage in metal hydrides. First, the importance of hydrogen energy is given. Second, an introductive piece of information about metal hydrides is given and the significance of metal hydride usage in hydrogen storage is discussed. In the next chapter, the concept of nanofluid usage in regard to thermal management of hydrogen storage in metal hydrides is given. Later, one of the thermal management techniques of hydrogen storage in metal hydrides, PCM (phase change material) usage, is discussed with studies related to the subjects in literature. Finally, different applications of machine learning in thermal management of hydrogen storage in metal hydrides are considered. It has been shown that thermal control for hydrogen storage in metal hydrides may be effectively achieved by employing nanofluids based on nanoparticles that have been carefully chosen for the system. Generally, Al2O3, CuO, MgO, Fe2O3, GO, GO-SiO2, and GO-TiO2 nanoparticles are used.in metal hydride storage thermal management system. The nanoparticle type and its loading amount are among the most important parameters that affect the improved performance of the hydrogen storage in metal hydrides. The quantity, melting temperature, thickness, thermal conductivity, depth of usage, and volume dispersion of PCMs are the fundamental parameters that are investigated in the studies for optimal values, taking into account additional parameters related to the metal hydride reactor systems, such as supply pressure, inlet velocity, temperature, etc. In order to get a faster response and a more compact hydrogen system, it is necessary to identify the ideal PCM thickness. Up to a certain degree, the hydrogen storage system benefits from PCM's higher thermal conductivity. The majority of machine learning research have been conducted to evaluate various characteristics and assess the potential of appropriate materials, metal hydrides, metals, material combinations, etc. for hydrogen storage. The findings of the studies under discussion have confirmed that machine learning is an effective, realistic means of predicting the parameters influencing hydrogen storage processes without sacrificing any of the drawbacks of experimental methods, such as high costs and lengthy search times. Future research should take into account more experimental investigations as well as a cost analysis of employing nanofluids and PCMs.
更多查看译文
关键词
Hydrogen storage,Metal hydrides,Thermal management,Nanofluid,PCM,Machine learning
AI 理解论文
溯源树
样例
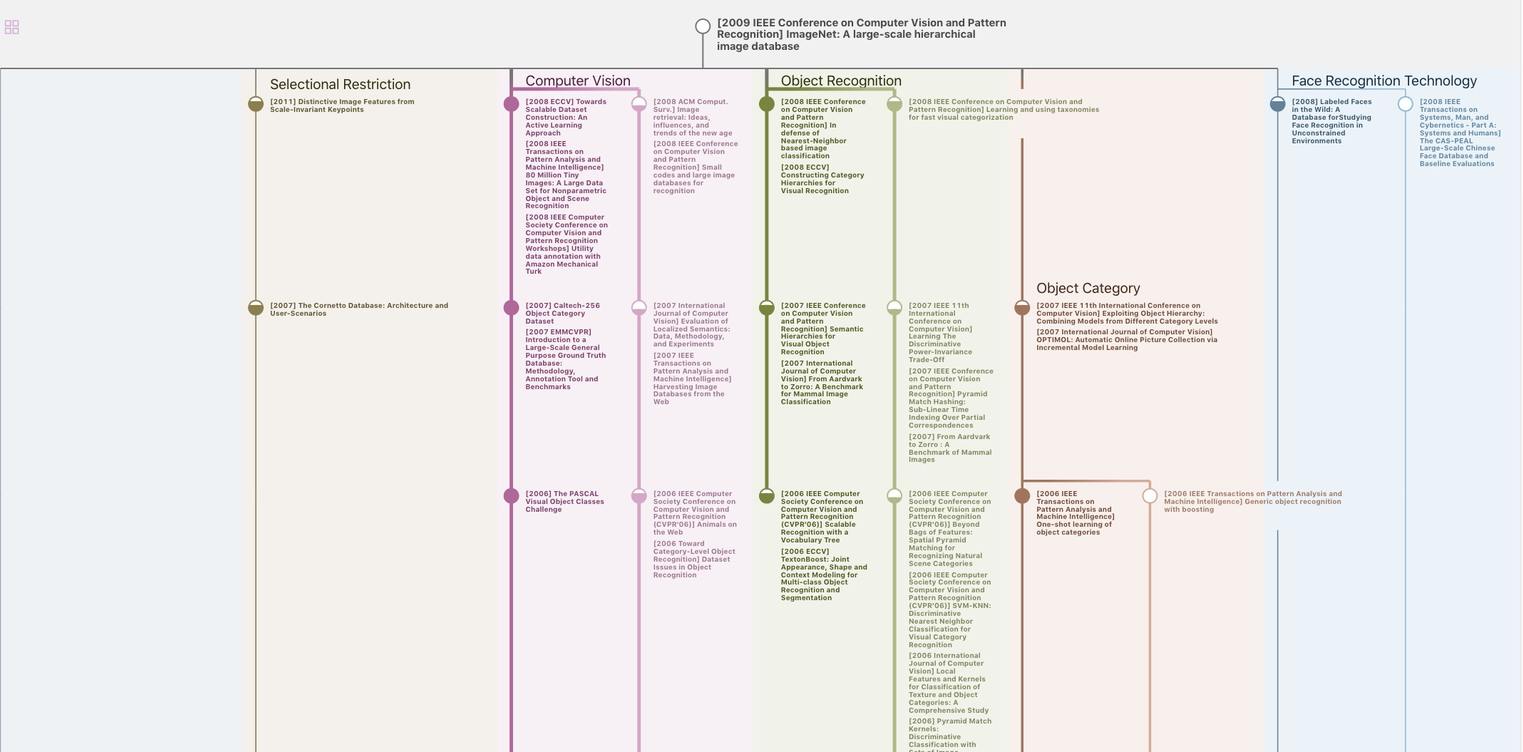
生成溯源树,研究论文发展脉络
Chat Paper
正在生成论文摘要