tinyRadar for Gesture Recognition: A Low-power System for Edge Computing.
Asia Pacific Conference on Circuits and Systems(2023)
摘要
Hand gesture recognition (HGR) plays a pivotal role in improving human-machine interaction across domains like smart homes/vehicles and wearable devices. While vision-based HGR systems encounter challenges with lighting, complex backgrounds, and occlusion, radar-based systems overcome these limitations by harnessing electromagnetic principles. This paper presents tinyRadar, a real-time, low-power, single-chip radar solution for HGR. By leveraging miniaturized mmWave radar hardware, tinyRadar offers a compact and cost-effective HGR solution. The Texas Instruments IWRL6432 radar is utilized, achieving a total power consumption of less than 80mW and a memory footprint of ~ 11 KB for the quantized inference model and < 256 KB for the entire system. The solution utilizes quantized depthwise separable convolutions and integrates a hardware accelerator and Cortex®-M4 microcontroller for real-time inference. With its small form factor and low power requirements, tinyRadar facilitates on-edge implementation, delivering 95% real-time inference accuracy for six gestures. This paper contributes to developing wearable gadgets and IoT devices that seamlessly incorporate HGR technology.
更多查看译文
关键词
hand gesture recognition,IWRL6432 single-chip mmWave radar,depthwise separable convolution,edge computing,low power,velocity-time map,angle-time map
AI 理解论文
溯源树
样例
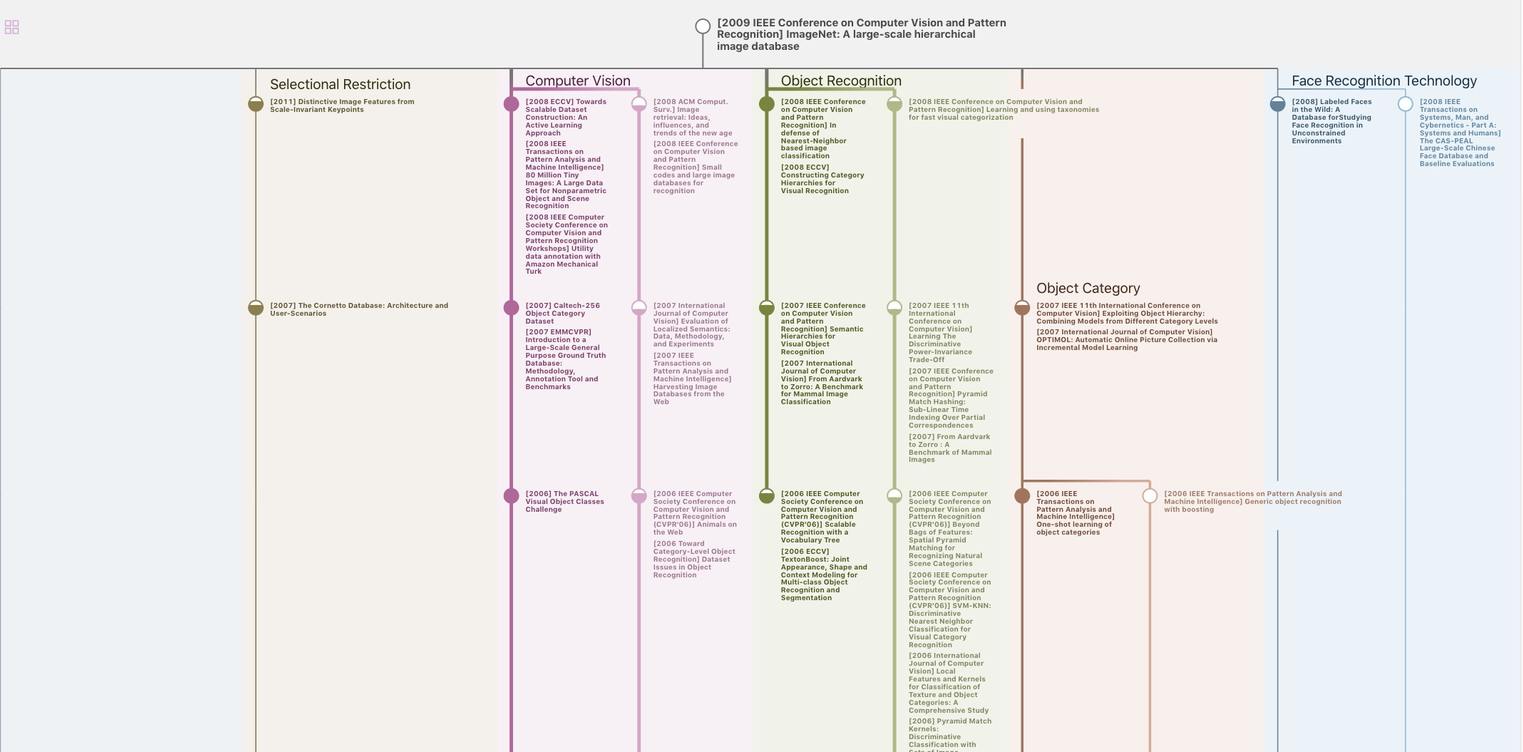
生成溯源树,研究论文发展脉络
Chat Paper
正在生成论文摘要