Multilevel Classification of Drowsiness States using ECG with Optimized Convolutional Neural Network.
IEEE/ACM International Conference on Green Computing and Communications(2023)
摘要
As drowsiness is recognized as one of the primary factors that threaten road safety, achieving a high-accuracy detection of drowsiness with finer granularity is crucial to ensuring safe driving. Current research has mainly focused on detecting drowsiness using EEG and ECG, typically classifying it into two states: alert and sleepy. However, given the multitude of possible parameters, the key factors that influence classification performance remain ambiguous. Furthermore, while a limited number of studies have gone beyond a binary classification of drowsiness, it is still unclear how well multilevel classification performs. To address these issues, we classified drowsiness states with different granularities using ECG signals from the DROZY dataset. We first performed a binary classification of drowsiness by comparing the combinations of parameters related to data preprocessing and model configuration. During binary classification, we achieved the state-of-the-art (SOTA) results in an accuracy of 99.65% by optimizing these parameters. These results also suggest that selecting a suitable window size along with the appropriate kernel size of the CNN model is essential for superior performance. We then performed a multilevel classification, categorizing drowsiness states into three, four, and eight levels, respectively. By adjusting the parameters, the multilevel classification reached accuracy levels comparable to binary classification: 98.72% for three-level classification, 98.54% for four-level classification and 98.33% for eight-level classification.
更多查看译文
关键词
deep learning,CNN model,drowsiness detection,driver state,physiological signal
AI 理解论文
溯源树
样例
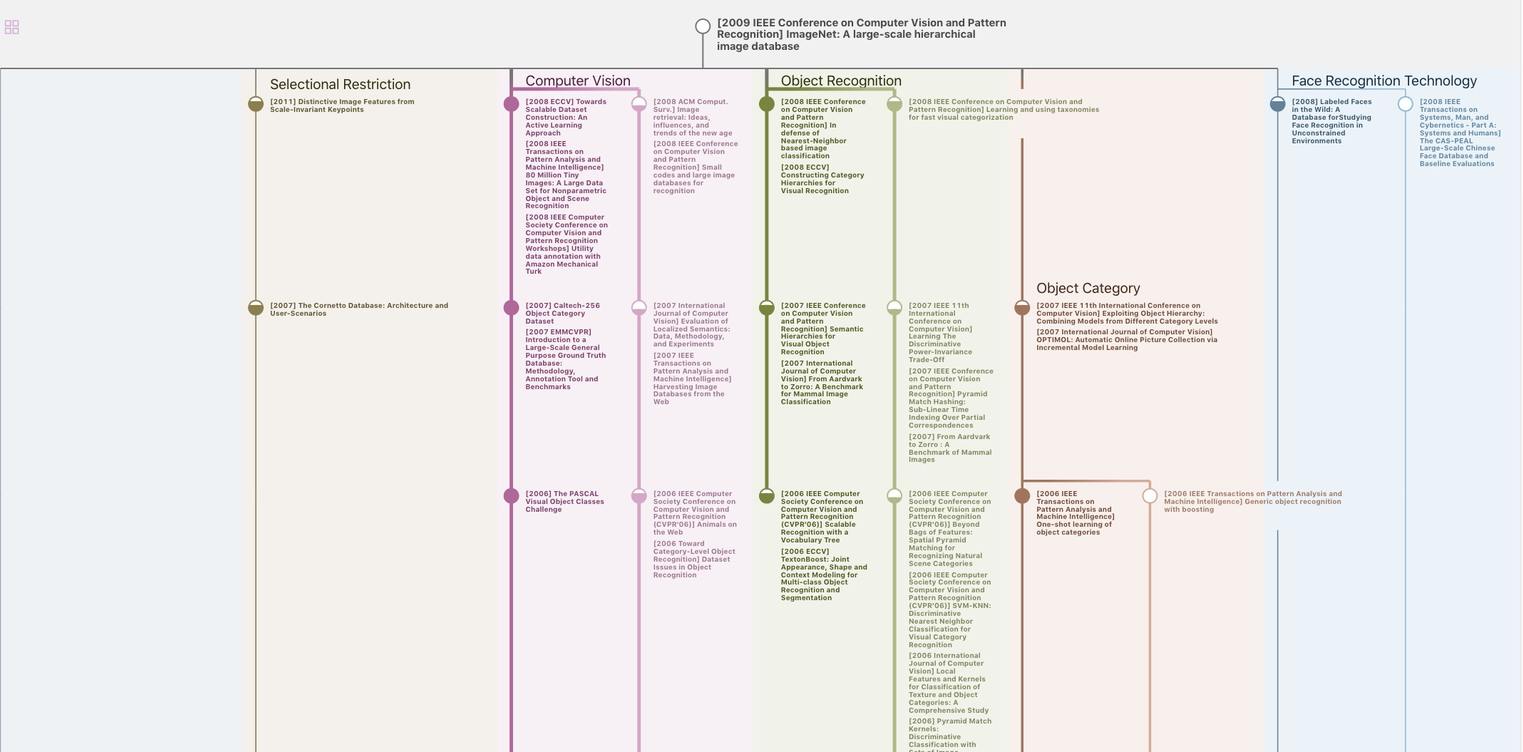
生成溯源树,研究论文发展脉络
Chat Paper
正在生成论文摘要