CNN and SVM-based Model for Effective Blackberry Leaf Disease Classification
2024 International Conference on Advancements in Smart, Secure and Intelligent Computing (ASSIC)(2024)
摘要
In this study, evaluated a classification model thoroughly intending to identify different plant leaf diseases. Anthracnose, Powdery Mildew, Rust, Septoria Plant Spot, Cane Spot, or Angular Leaf Spot are just a few of the six different forms of leaf diseases that the model was able to forecast using cutting-edge methods such as Convolutional Neural Networks (CNNs) or a Random Forest Classifier. Precision, Recall, & F1-Score were used as the primary performance indicators to evaluate the model's effectiveness. With the aid of these measurements, we were able to assess the model's precision, its capacity to recognize pertinent occurrences, and the harmony between these features. The analysis, which was based on the above table, produced interesting findings. For several diseases, the model displayed outstanding Precision values, including Anthracnose at 87.72%, Powdery Mildew at 82.10%, and Rust at 82.33%. These results demonstrate the model's accuracy in diagnosing particular diseases. Additionally, the model demonstrated impressive Recall values, including 73.97% for Downy Mildew, and 73.26% for Cercospora Green Spot, highlighting its success in accurately capturing actual disease cases. Unfortunately, the table does not contain these specific values. In conclusion, the study highlights the potential for the CNN on Random Forest technique to classify leaf diseases with a high degree of accuracy and dependability. This model shows promise towards practical applications for farming and plant disease management, facilitating improvements in agricultural disease diagnosis and plant health management, with an average certainty of 72.42% (Weighted Overall F1-Score). With scores like 78.74% with Anthracnose, 76.43% in Powdery Mildew, and 74.58% in Septoria Leaf Spot, the F1-Score, which mixes Precision and Recall, revealed strong performance across diverse disease classes. These results highlight the model's general efficacy in disease classification.
更多查看译文
AI 理解论文
溯源树
样例
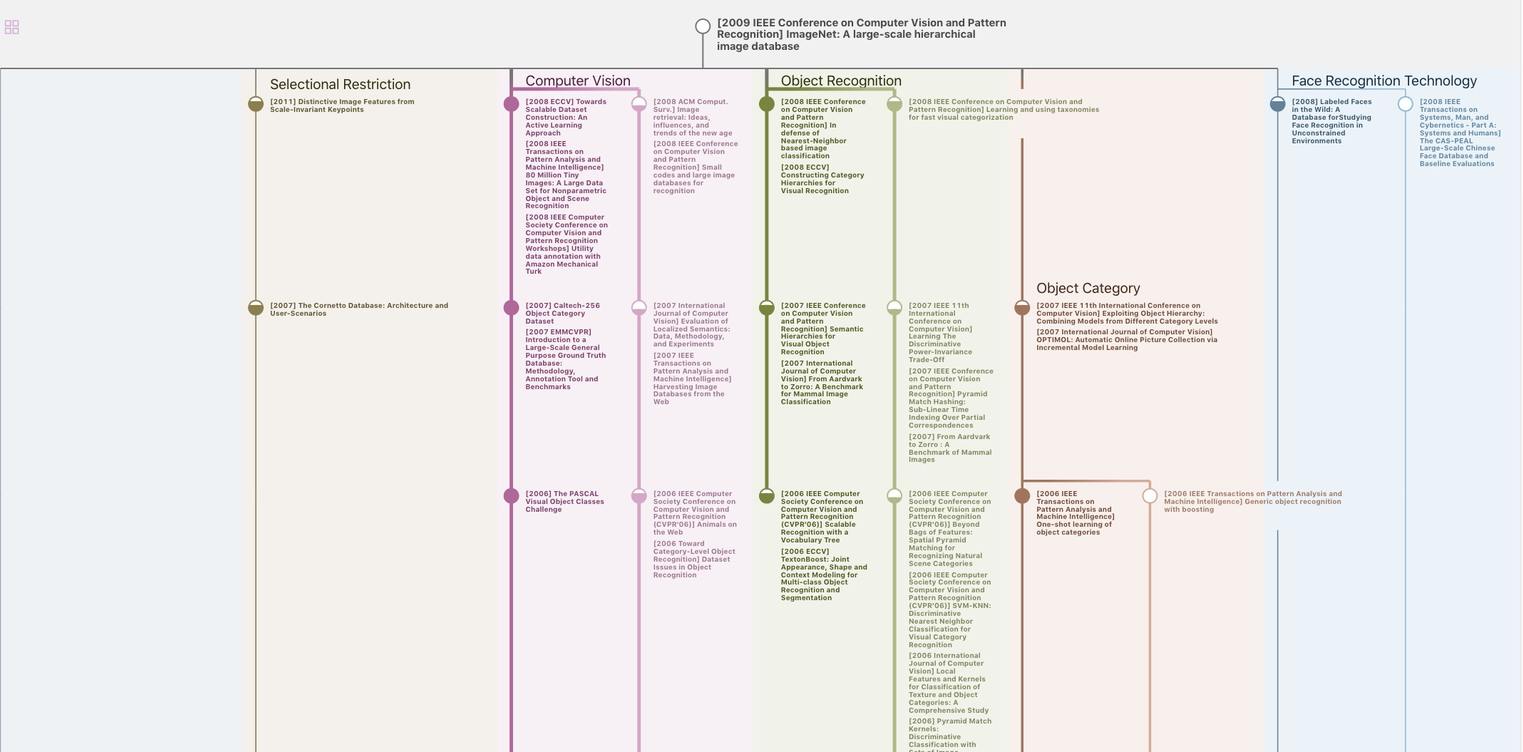
生成溯源树,研究论文发展脉络
Chat Paper
正在生成论文摘要