Enhancing Precision Medicine through Artificial Neural Networks for Phenotyping and Risk Prediction of Rare Genetic Disorders
2024 International Conference on Advancements in Smart, Secure and Intelligent Computing (ASSIC)(2024)
摘要
In this study, we present an innovative approach to enhance precision medicine for rare genetic disorders by leveraging a comprehensive Artificial Neural Network (ANN) architecture. This architecture amalgamates three pivotal algorithms: Convolutional Neural Network (CNN) for image analysis, Recurrent Neural Network (RNN) for sequential clinical data, and Dense Neural Network (DNN) for feature integration and risk prediction. CNNs are specialized for efficient grid-like data handling, making them ideal for image analysis and pattern recognition. RNNs, on the other hand, excel in processing sequential data due to their inherent ability to retain information from previous inputs. DNNs play a fundamental role in capturing intricate patterns and relationships in the data. Our approach strategically integrates these algorithms into a cohesive ANN framework, enabling a comprehensive analysis of genetic data, which is critical in the domain of rare genetic disorders. By leveraging the capabilities of CNN, RNN, and DNN, a comprehensive strategy emerges, enabling accurate risk prediction and phenotype analysis. This approach significantly contributes to the progression of precision medicine, specifically tailored for rare genetic disorders.
更多查看译文
AI 理解论文
溯源树
样例
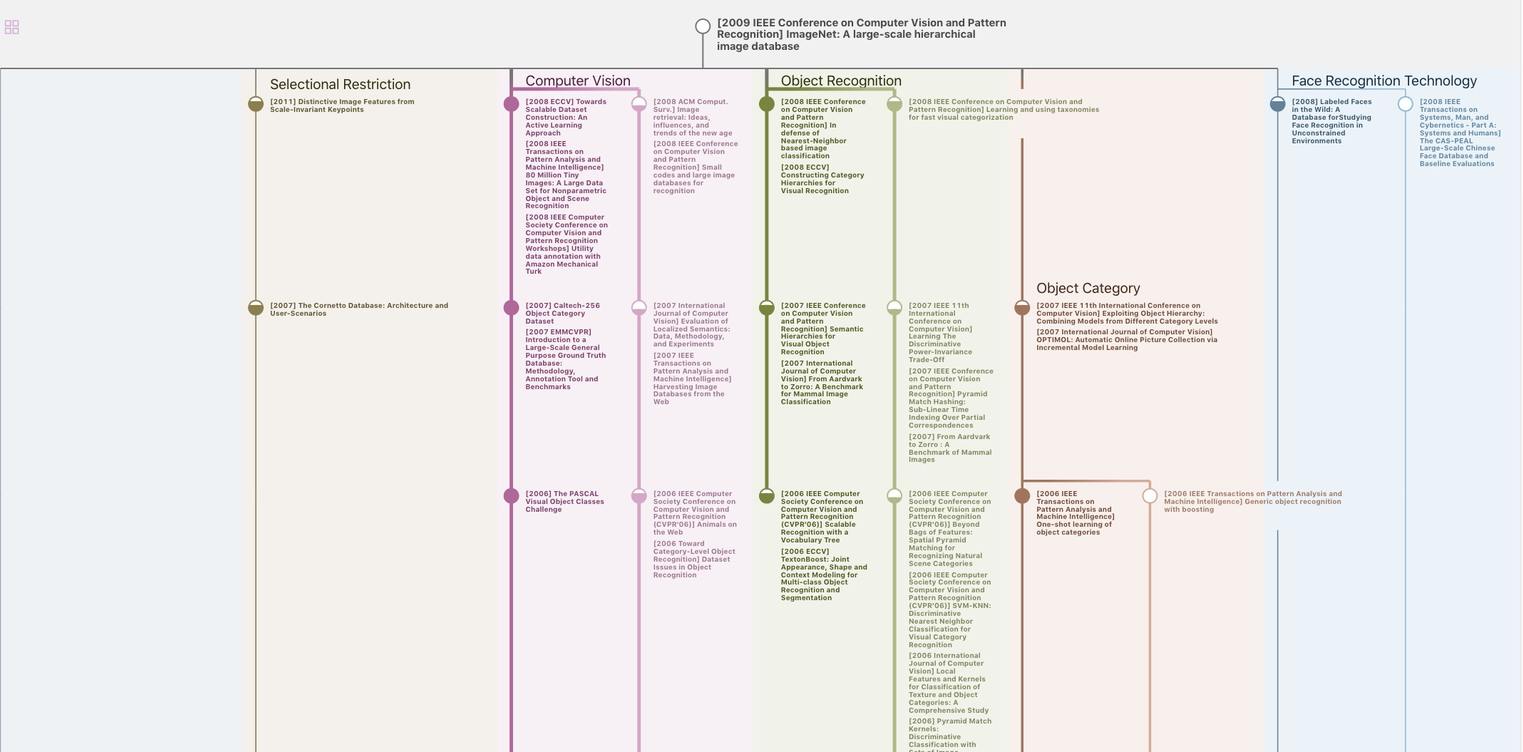
生成溯源树,研究论文发展脉络
Chat Paper
正在生成论文摘要