Knowledge Distillation Based Lightweight Deep Neural Network for Automatic Modulation Classification
2023 9th International Conference on Computer and Communications (ICCC)(2023)
摘要
Deep learning is widely used in the field of automatic modulation classification. Although the present state-of-art deep learning models for Automatic Modulation Classification (AMC) have high accuracy, their computational complexities are large, which is not friendly for practical considerations. To address this issue, a novel lightweight student network named GSCNET is proposed, based on the Ghost module and depthwise separable convolution. Furthermore, three different loss functions—cross-entropy loss, Kullback-Leibler (KL) divergence loss, and soft label-based cross-entropy loss are used to supervise the training of GSCNET. Specifically, the cross-entropy loss is used to minimize the distance between predictions and true label. Regarding the last two losses, they are employed to minimize the discrepancy between predictions made by a student model and those from a teacher model (where the teacher model has better classification performance than GSCNET), which are derived from the methodology of knowledge distillation. As a result, the proposed algorithm can benefit from the knowledge of a teacher model, which is more discriminative than relying solely on the cross-entropy loss. Finally, the proposed GSCNET exhibits high classification performance with low computational complexity.
更多查看译文
关键词
Automatic modulation classification,knowledge distillation,deep learning,convolutional neural network
AI 理解论文
溯源树
样例
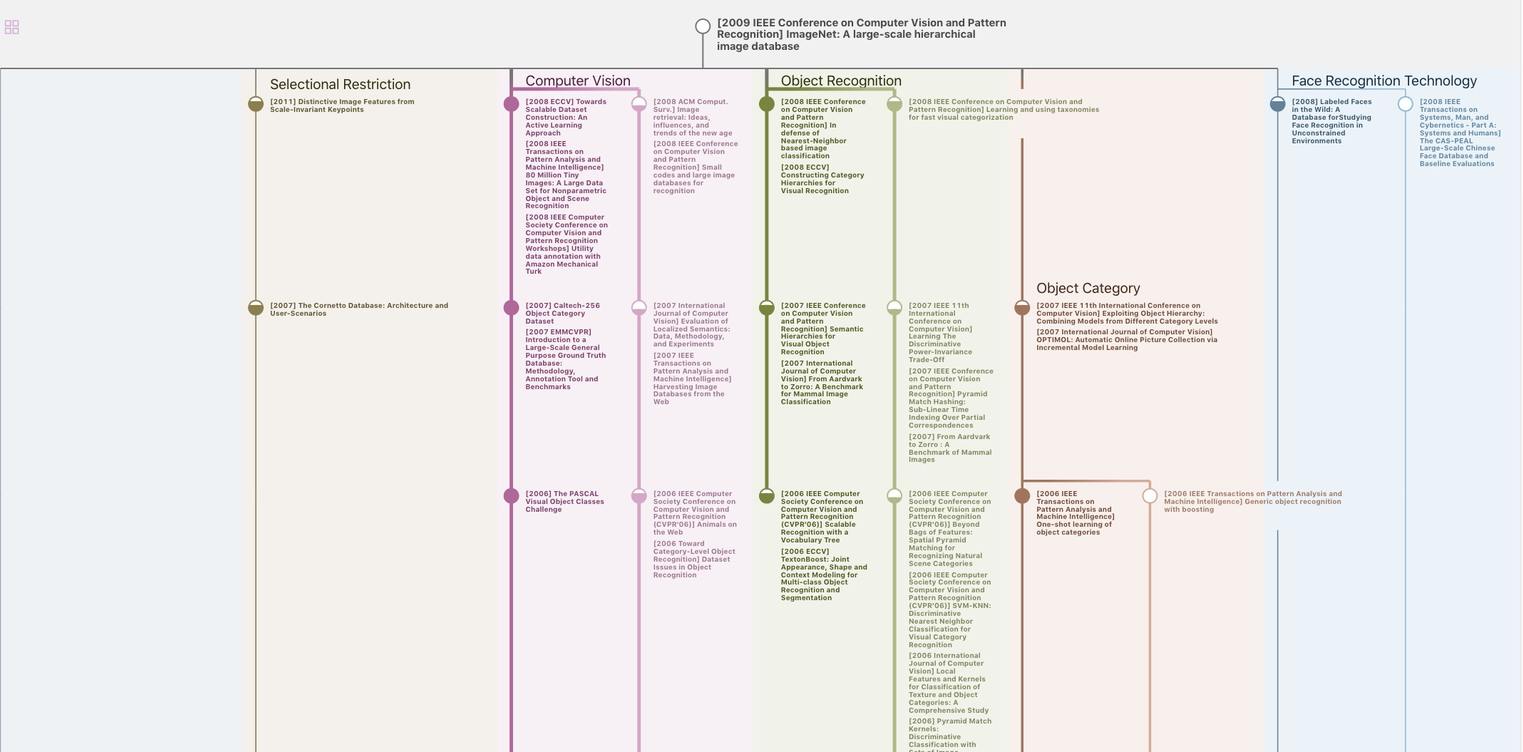
生成溯源树,研究论文发展脉络
Chat Paper
正在生成论文摘要