A Weighted Co-Training Framework for Emotion Recognition Based on EEG Data Generation Using Frequency-Spatial Diffusion Transformer
IEEE Transactions on Affective Computing(2024)
摘要
Emotion recognition based on EEG signals has been a challenging task. The acquisition of EEG signals is complex, time-consuming, and has a high overhead. Artificial Intelligence Generated Content technology has been developing rapidly in image and sound generation, but effective generative models for EEG signal generation have rarely appeared. To address the problem, we propose a weighted co-training framework for emotion recognition using a frequency-spatial diffusion transformer. We propose the EEG signal generation model by utilizing frequency-spatial correlation. In the first step, we use forward diffusion to add noise to the real samples and then use the proposed model to denoise and restore the real EEG signals. It ensures that the trained generative model can generate real EEG signals. Then, we use the denoising process of the proposed model to generate a large amount of data and then use the pseudo-data weighting module to evaluate the generated samples further. Finally, the actual samples and weighted pseudo-data are used to train the classifier for better generalization jointly. We conducted comprehensive experiments on three EEG emotion recognition benchmark datasets. The experimental results show that our method improves by 0.96%-1.91% compared to state-of-the-art methods. In addition, quantitative and qualitative analysis proves the effectiveness of our proposed method.
更多查看译文
关键词
EEG data generation,emotion recognition,frequency-spatial diffusion transformer,weighted co-training framework
AI 理解论文
溯源树
样例
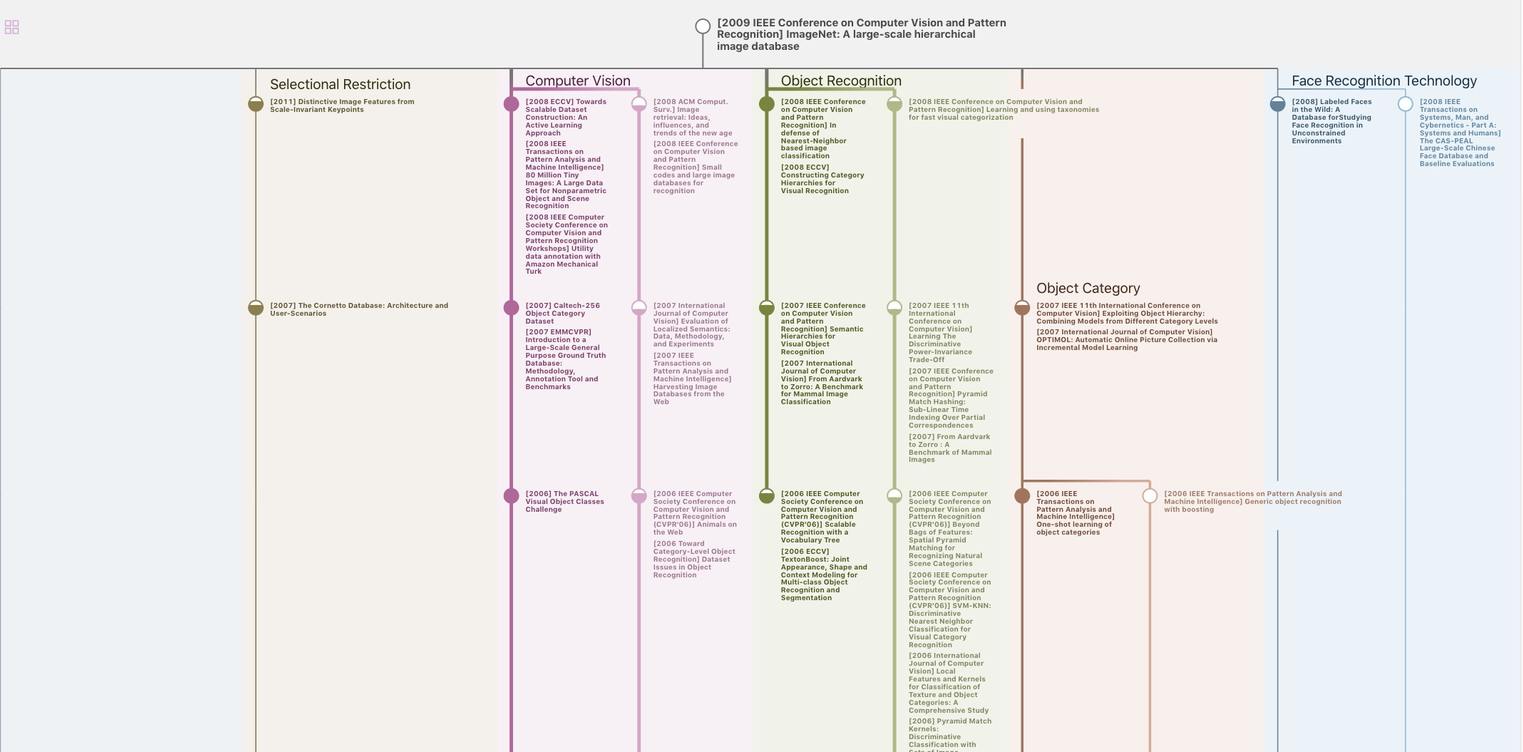
生成溯源树,研究论文发展脉络
Chat Paper
正在生成论文摘要