An Artificial Intelligence-Driven Approach for Automatic Evaluation of Right-to-Left Shunt Grades in Saline-Contrasted Transthoracic Echocardiography
Ultrasound in Medicine & Biology(2024)
摘要
Background
Intracardiac or pulmonary right-to-left shunt (RLS) is a common cardiac anomaly associated with an increased risk of neurological disorders, specifically cryptogenic stroke. Saline-contrasted transthoracic echocardiography (scTTE) is often used for RLS diagnosis. However, the identification of saline microbubbles in the left heart can be challenging for novice residents, potentially leading to a delay in diagnosis and treatment. In this study, we proposed an artificial intelligence (AI)-based algorithm designed to automatically detect microbubbles in scTTE images and evaluate right-to-left shunt grades. This tool aims to support residency training and decrease the workload of cardiologists.
Methods
A dataset of 23,665 scTTE images obtained from 174 individuals was included in this study. This dataset was partitioned into a training set (n = 20,475) and an internal validation set (n = 3,190) on a patient-level basis. An additional cohort of 33 patients diagnosed with cryptogenic ischemic stroke was enrolled as an external validation set. The proposed algorithm for right-to-left shunt degree classification employed the EfficientNet-b4 model, and the model's performance was evaluated using the area under the receiver operating characteristic curve (AUC), sensitivity, and specificity, and compared to the performance of residents.
Results
Our AI model demonstrated robust performance with an accuracy of 0.926, sensitivity of 0.827, and specificity of 0.951 on the internal testing dataset. In the external validation set, our AI model exhibited diagnostic accuracy, sensitivity, and specificity of 0.864, 0.818, and 0.909, respectively. In comparison, residents achieved values of 0.727, 0.636, and 0.818, respectively.
Conclusion
Our AI model provides a swift, precise, and easily deployable methodology for grading the degree of right-to-left shunt in scTTE, carrying substantial implications for routine clinical practice. Residents can benefit from our artificial intelligence-based algorithm, enhancing both the accuracy and efficiency of RLS diagnosis.
更多查看译文
关键词
Right-to-left shunt grade,Artificial intelligence,Saline-contrasted transthoracic echocardiography
AI 理解论文
溯源树
样例
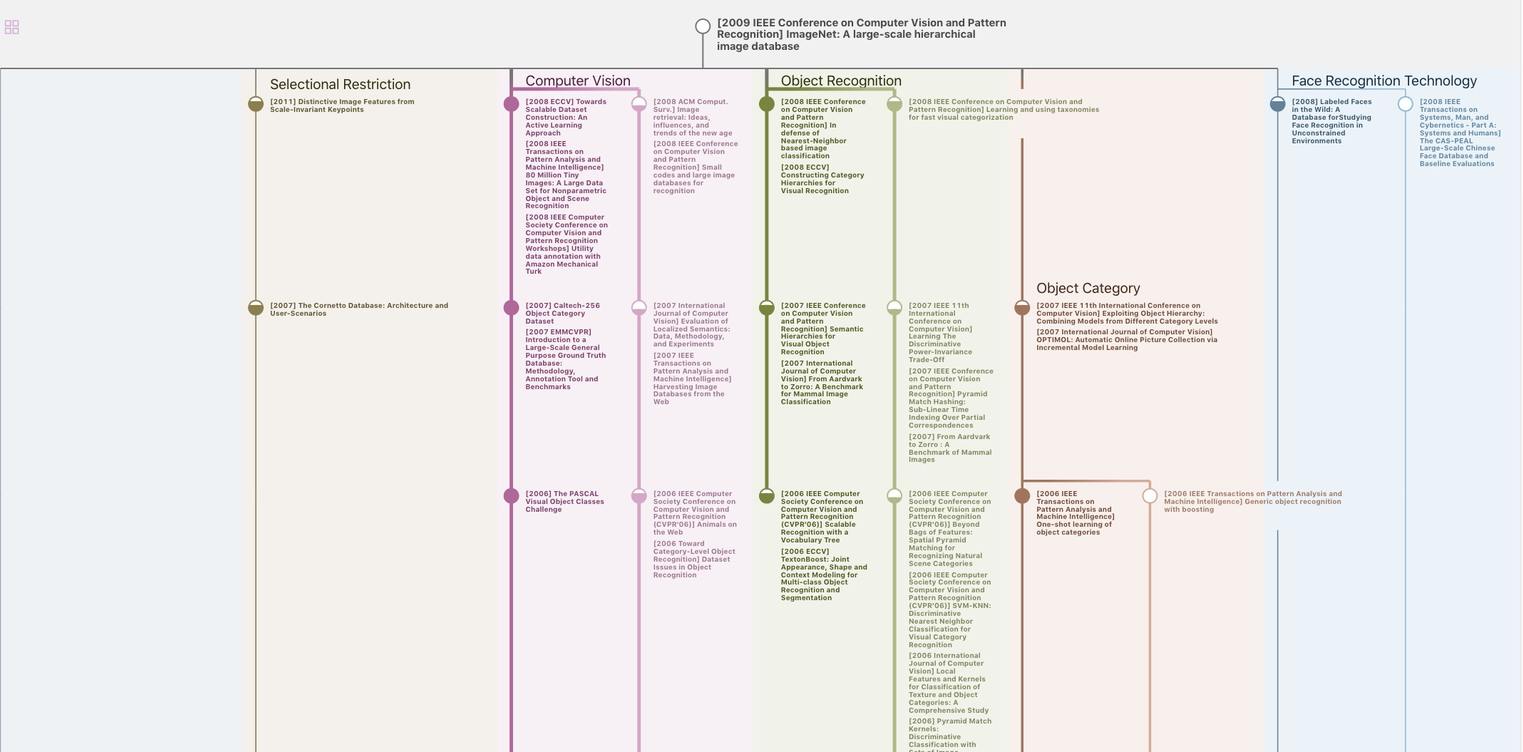
生成溯源树,研究论文发展脉络
Chat Paper
正在生成论文摘要