Assessing LLMs in Malicious Code Deobfuscation of Real-world Malware Campaigns
arxiv(2024)
摘要
The integration of large language models (LLMs) into various pipelines is
increasingly widespread, effectively automating many manual tasks and often
surpassing human capabilities. Cybersecurity researchers and practitioners have
recognised this potential. Thus, they are actively exploring its applications,
given the vast volume of heterogeneous data that requires processing to
identify anomalies, potential bypasses, attacks, and fraudulent incidents. On
top of this, LLMs' advanced capabilities in generating functional code,
comprehending code context, and summarising its operations can also be
leveraged for reverse engineering and malware deobfuscation. To this end, we
delve into the deobfuscation capabilities of state-of-the-art LLMs. Beyond
merely discussing a hypothetical scenario, we evaluate four LLMs with
real-world malicious scripts used in the notorious Emotet malware campaign. Our
results indicate that while not absolutely accurate yet, some LLMs can
efficiently deobfuscate such payloads. Thus, fine-tuning LLMs for this task can
be a viable potential for future AI-powered threat intelligence pipelines in
the fight against obfuscated malware.
更多查看译文
AI 理解论文
溯源树
样例
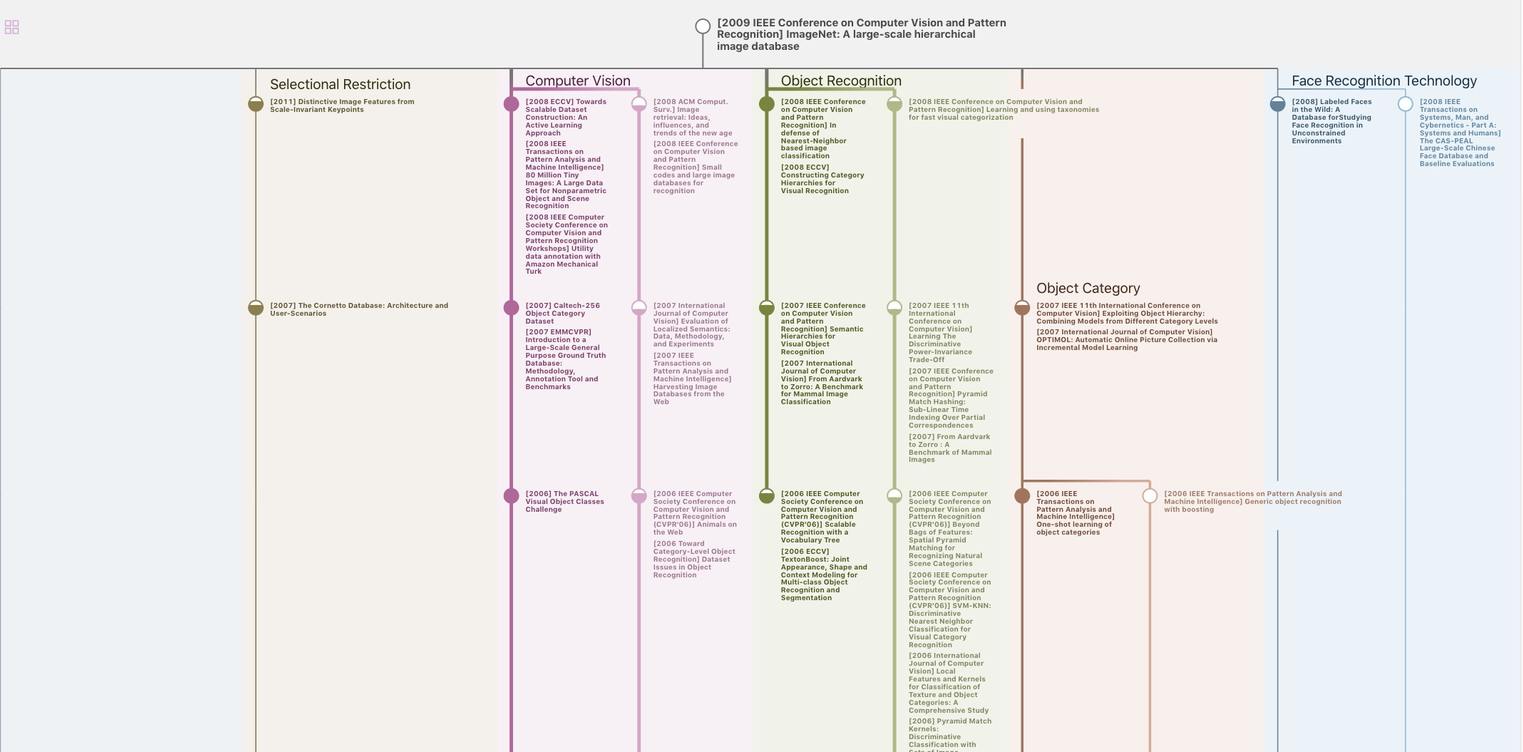
生成溯源树,研究论文发展脉络
Chat Paper
正在生成论文摘要