Detecting Spectral Breaks in Spiked Covariance Models
arxiv(2024)
摘要
In this paper, the key objects of interest are the sequential covariance
matrices 𝐒_n,t and their largest eigenvalues. Here, the matrix
𝐒_n,t is computed as the empirical covariance associated with
observations {𝐱_1,…,𝐱_⌊ nt ⌋}, for
t∈ [0,1]. The observations 𝐱_1,…,𝐱_n are assumed
to be i.i.d. p-dimensional vectors with zero mean, and a covariance matrix
that is a fixed-rank perturbation of the identity matrix. Treating {𝐒_n,t}_t ∈ [0,1] as a matrix-valued stochastic process indexed
by t, we study the behavior of the largest eigenvalues of 𝐒_n,t,
as t varies, with n and p increasing simultaneously, so that p/n → y
∈ (0,1). As a key contribution of this work, we establish the weak
convergence of the stochastic process corresponding to the sample spiked
eigenvalues, if their population counterparts exceed the critical
phase-transition threshold. Our analysis of the limiting process is fully
comprehensive revealing, in general, non-Gaussian limiting processes.
As an application, we consider a class of change-point problems, where the
interest is in detecting structural breaks in the covariance caused by a change
in magnitude of the spiked eigenvalues. For this purpose, we propose two
different maximal statistics corresponding to centered spiked eigenvalues of
the sequential covariances. We show the existence of limiting null
distributions for these statistics, and prove consistency of the test under
fixed alternatives. Moreover, we compare the behavior of the proposed tests
through a simulation study.
更多查看译文
AI 理解论文
溯源树
样例
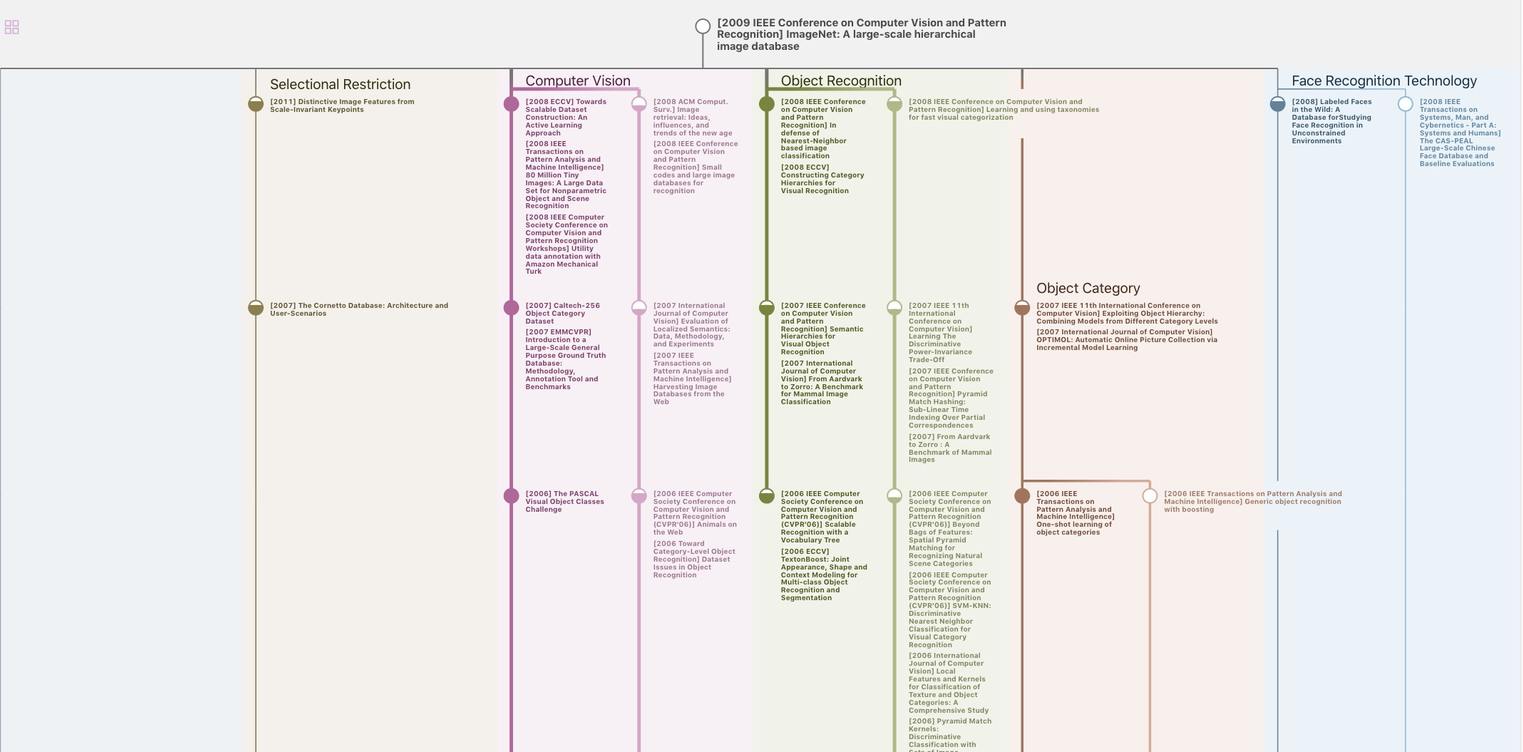
生成溯源树,研究论文发展脉络
Chat Paper
正在生成论文摘要