Exploring the Efficacy of Learning Techniques in Model Extraction Attacks on Image Classifiers: A Comparative Study
Applied Sciences(2024)
摘要
In the rapidly evolving landscape of cybersecurity, model extraction attacks pose a significant challenge, undermining the integrity of machine learning models by enabling adversaries to replicate proprietary algorithms without direct access. This paper presents a comprehensive study on model extraction attacks towards image classification models, focusing on the efficacy of various Deep Q-network (DQN) extensions for enhancing the performance of surrogate models. The goal is to identify the most efficient approaches for choosing images that optimize adversarial benefits. Additionally, we explore synthetic data generation techniques, including the Jacobian-based method, Linf-projected Gradient Descent (LinfPGD), and Fast Gradient Sign Method (FGSM) aiming to facilitate the training of adversary models with enhanced performance. Our investigation also extends to the realm of data-free model extraction attacks, examining their feasibility and performance under constrained query budgets. Our investigation extends to the comparison of these methods under constrained query budgets, where the Prioritized Experience Replay (PER) technique emerges as the most effective, outperforming other DQN extensions and synthetic data generation methods. Through rigorous experimentation, including multiple trials to ensure statistical significance, this work provides valuable insights into optimizing model extraction attacks.
更多查看译文
关键词
synthetic data,security,active learning,reinforcement learning,model extraction attack,image classification
AI 理解论文
溯源树
样例
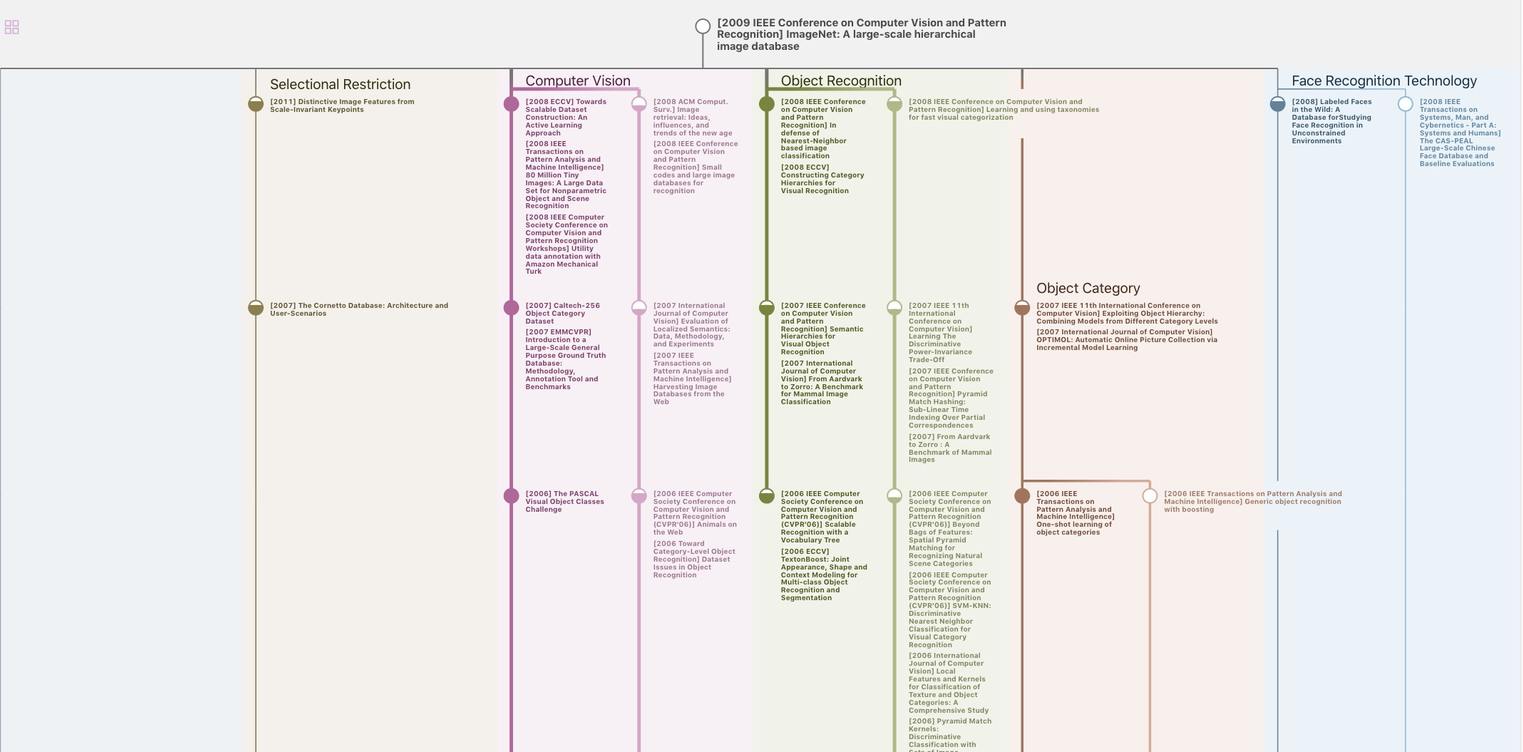
生成溯源树,研究论文发展脉络
Chat Paper
正在生成论文摘要