Optimizing anti-collision strategy for MASS: A safe reinforcement learning approach to improve maritime traffic safety
Ocean & Coastal Management(2024)
摘要
Maritime autonomous surface ships (MASS) promise enhanced efficiency, reduced human errors, and to improve maritime traffic safety. However, MASS navigation in complex maritime traffic presents challenges, especially in collision avoidance strategy optimization (CASO). This paper proposes a novel risk-based CASO approach based on safe reinforcement learning (SRL) with a reliability and risk hierarchical critic network (SRL-R2HCN) approach. Key steps in developing the approach start with the formulation of collision risk assessment. This is followed by the construction of a hierarchical network structure, supplemented by the supporting reward function, multi-objective function, and reliability measurement to realize the SRL-R2HCN. Finally, simulation experiments are conducted in mixed obstacle scenarios, and the results are compared with traditional algorithms to showcase the advancement and fidelity of the new SRL-R2HCN method. The results demonstrate that the proposed method can accurately assess collision risks in mixed obstacle scenarios and generate safe, efficient, and reliable collision avoidance strategies. The outcomes of this research provide a sound theoretical basis for the future development of MASS navigation safety and significant potential to improve the safe and efficient operations of MASS. Furthermore, the methodology could also benefit maritime transportation and shipping management.
更多查看译文
关键词
Collision avoidance decision-making,Safe reinforcement learning,Maritime traffic safety,Collision risk,Maritime autonomous surface ships (MASS)
AI 理解论文
溯源树
样例
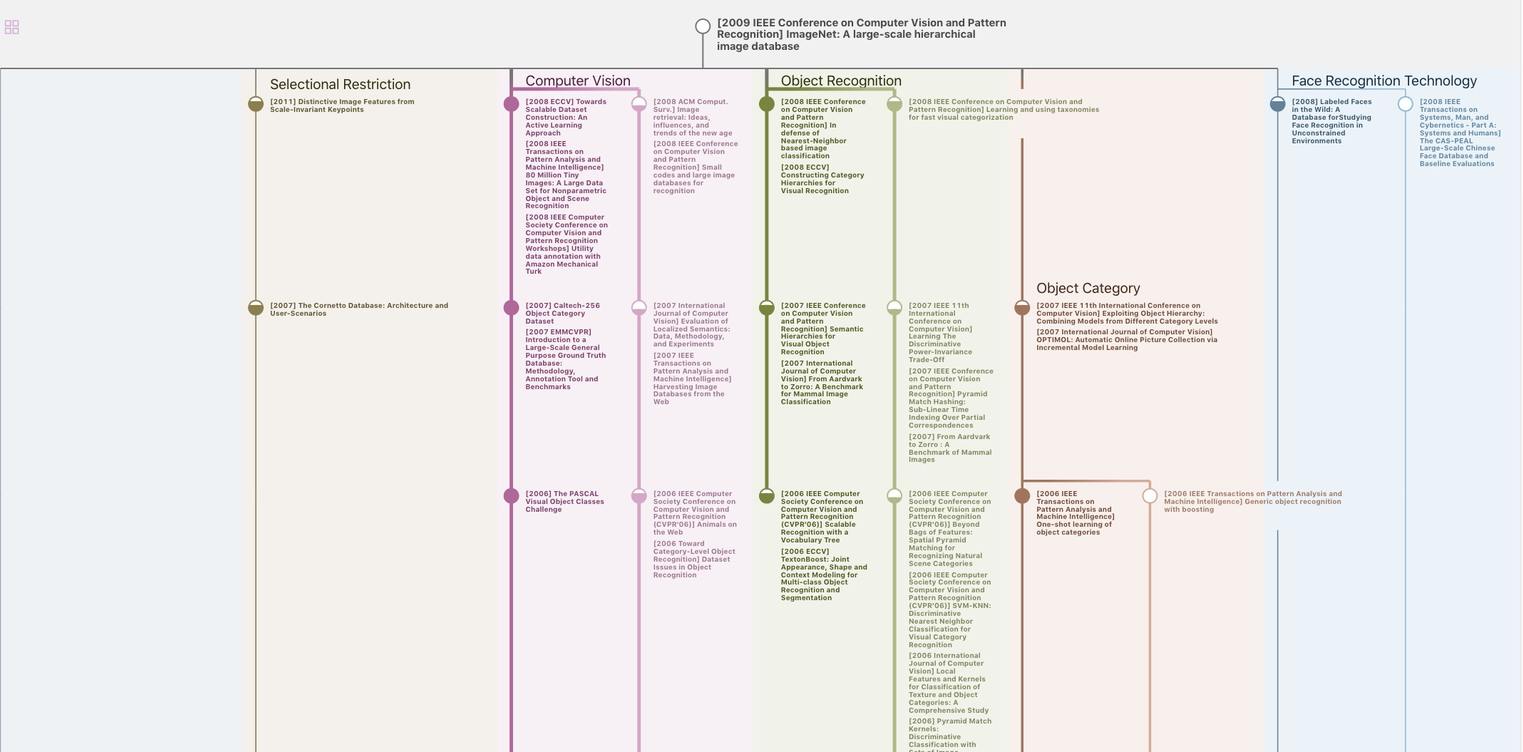
生成溯源树,研究论文发展脉络
Chat Paper
正在生成论文摘要