Parsing and encoding interactive phrase structure for implicit discourse relation recognition
Neural Computing and Applications(2024)
摘要
Implicit discourse relation recognition (IDRR) is to detect and classify relation sense between two text segments without an explicit connective. Existing neural network models learn a semantic representation for each argument from its compositional words and classify discourse relation by the interactive semantic representation of an argument pair. As the basic English unit, a word only carries a simple and limited meaning that may be incorrectly interpreted in an argument without considering its syntactic context. This motivates us to explore whether we can learn an argument’s semantic representation from another language unit phrase which usually contains full contextual meaning. We also argue that some semantic connection in between phrase pairs can be further exploited to infer the discourse relation between arguments. In this paper, we propose an Attentive Phrase Interaction Learning (APIL) model to parse and encode the interactive phrase structure for the IDRR task. In APIL, we propose a minimum subtree algorithm to obtain the phrase sequence of input arguments from its constituency syntax tree. We also design an Attentive Matching Network to learn the representation for each phrase from both arguments’ semantic context and words’ linguistic evidence. Furthermore, we propose a Phrase Inference Network to encode the linguistic relations of phrase pairs as semantic connections for relation inference. Experiments on the PDTB corpus show that our APIL model outperforms the state-of-the-art algorithms.
更多查看译文
关键词
Implicit discourse relation recognition,Phrase structure,Constituent syntactic parsing,Neural network
AI 理解论文
溯源树
样例
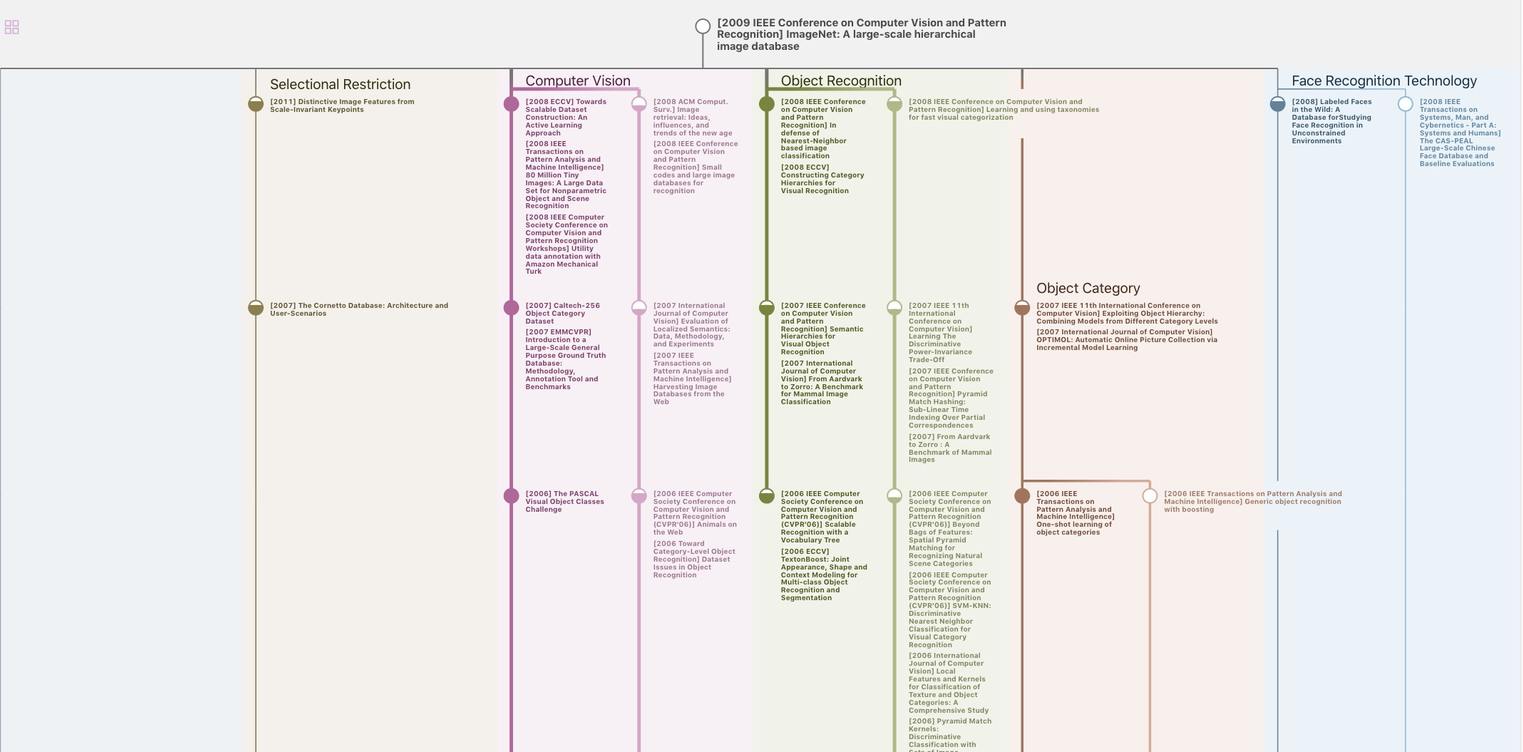
生成溯源树,研究论文发展脉络
Chat Paper
正在生成论文摘要