Estimating and explaining regional land value distribution using attention-enhanced deep generative models
Computers in Industry(2024)
摘要
Accurate land valuation is crucial in sustainable urban development, influencing pivotal decisions on resource allocation and land-use strategies. Most existing studies, primarily using point-based modeling approaches, face challenges on granularity, generalizability, and spatial effect capturing, limiting their effectiveness in regional land valuation with high granularity. This study therefore proposes the LVGAN (i.e., land value generative adversarial networks) framework for regional land value estimation. The LVGAN model redefines land valuation as an image generation task, employing deep generative techniques combined with attention mechanisms to forecast high-resolution relative value distributions for informed decision-making. Applied to a case study of New York City (NYC), the LVGAN model outperforms typical deep generative methods, with MAE (Mean Absolute Error) and MSE (Mean Squared Error) averagely reduced by 36.58 % and 59.28 %, respectively. The model exhibits varied performance across five NYC boroughs and diverse urban contexts, excelling in Manhattan with limited value variability, and in areas characterized by residential zoning and high density. It identifies influential factors such as road network, built density, and land use in determining NYC land valuation. By enhancing data-driven decision-making at early design stages, the LVGAN model can promote stakeholder engagement and strategic planning for sustainable and well-structured urban environments.
更多查看译文
关键词
Land price estimation,Generative adversarial networks (GAN),Generative artificial intelligence (generative AI),Deep learning,Attention mechanism,Deep generative models
AI 理解论文
溯源树
样例
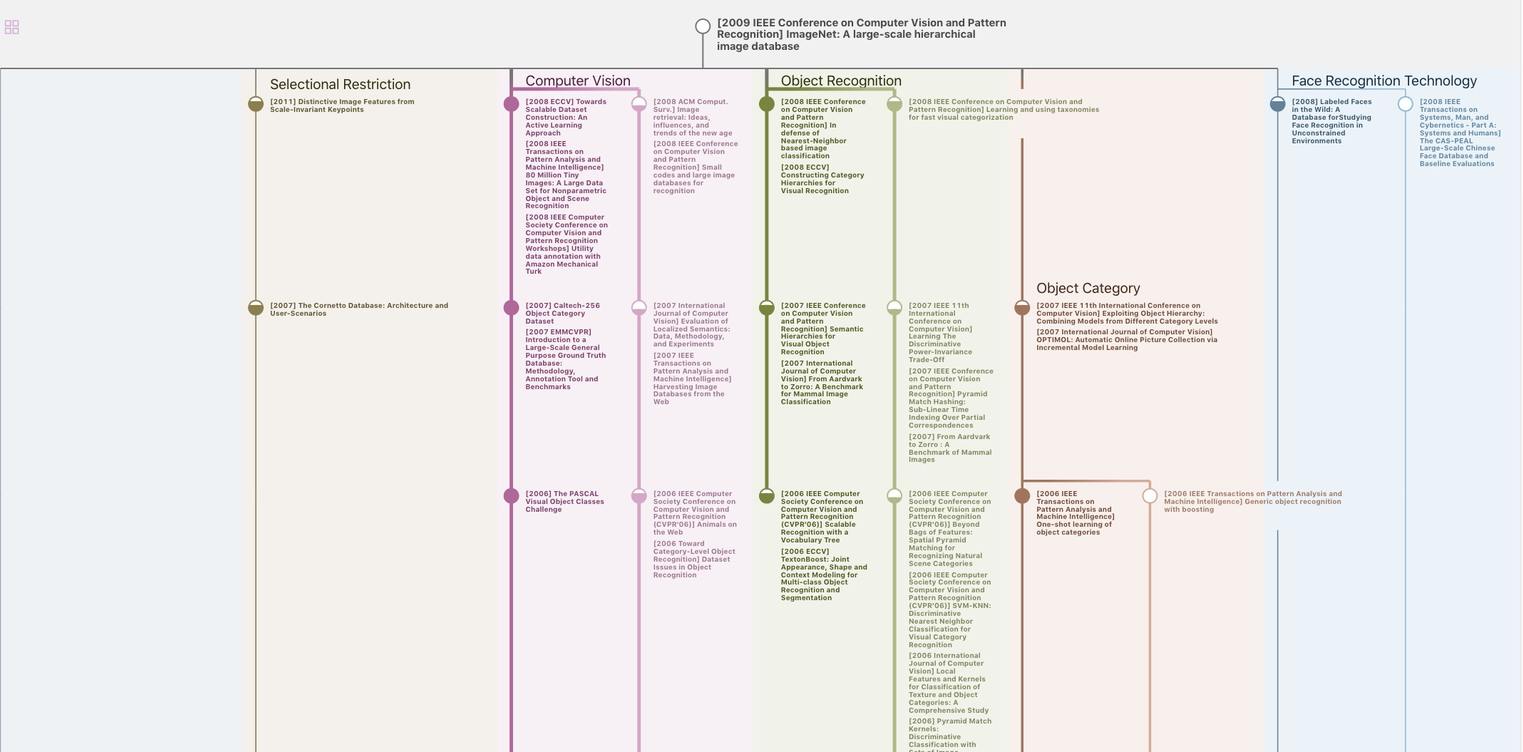
生成溯源树,研究论文发展脉络
Chat Paper
正在生成论文摘要