Meta-learning-based approach for tool condition monitoring in multi-condition small sample scenarios
Mechanical Systems and Signal Processing(2024)
摘要
Tool Condition Monitoring (TCM) technology in machining is crucial for maintaining safety and optimizing costs. However, its practical application faces two significant challenges: difficulties in data collection and a decline in generalization performance across different monitoring tasks. To this end, a hybrid feature boundary-enhanced meta-learning network with adaptive gradients (HFBEAML) is proposed. This method combines cutting process parameters with time-series signal features, employing a multimodal one-dimensional Convolutional Neural Network (1D-CNN) based on the DenseNet architecture and a multi-head self-attention mechanism (MHSA) to mine multi-dimensional features sensitive to tool wear. To further enhance the model’s feature discrimination capability, a multi-loss joint optimization strategy is introduced, combining task-level loss with an improved triplet loss. This approach incorporates strategies for adaptive boundaries and handling the hardest positive and negative sample sets, thereby enhancing the robustness of feature metrics. Additionally, this research innovatively proposes an adaptive meta-level gradient update mechanism, dynamically adjusting gradient weights according to the characteristics of multiple tasks, aiming to improve the model’s generalization ability in multi-task learning environments. The effectiveness of the proposed method is demonstrated through experiments in two different scenarios, comparing its results with five other models showcasing its significant advantages in multi-task, small-sample data monitoring environments.
更多查看译文
关键词
Tool condition monitoring,Small sample,Meta-learning,Multi-loss joint optimization,Adaptive gradient update
AI 理解论文
溯源树
样例
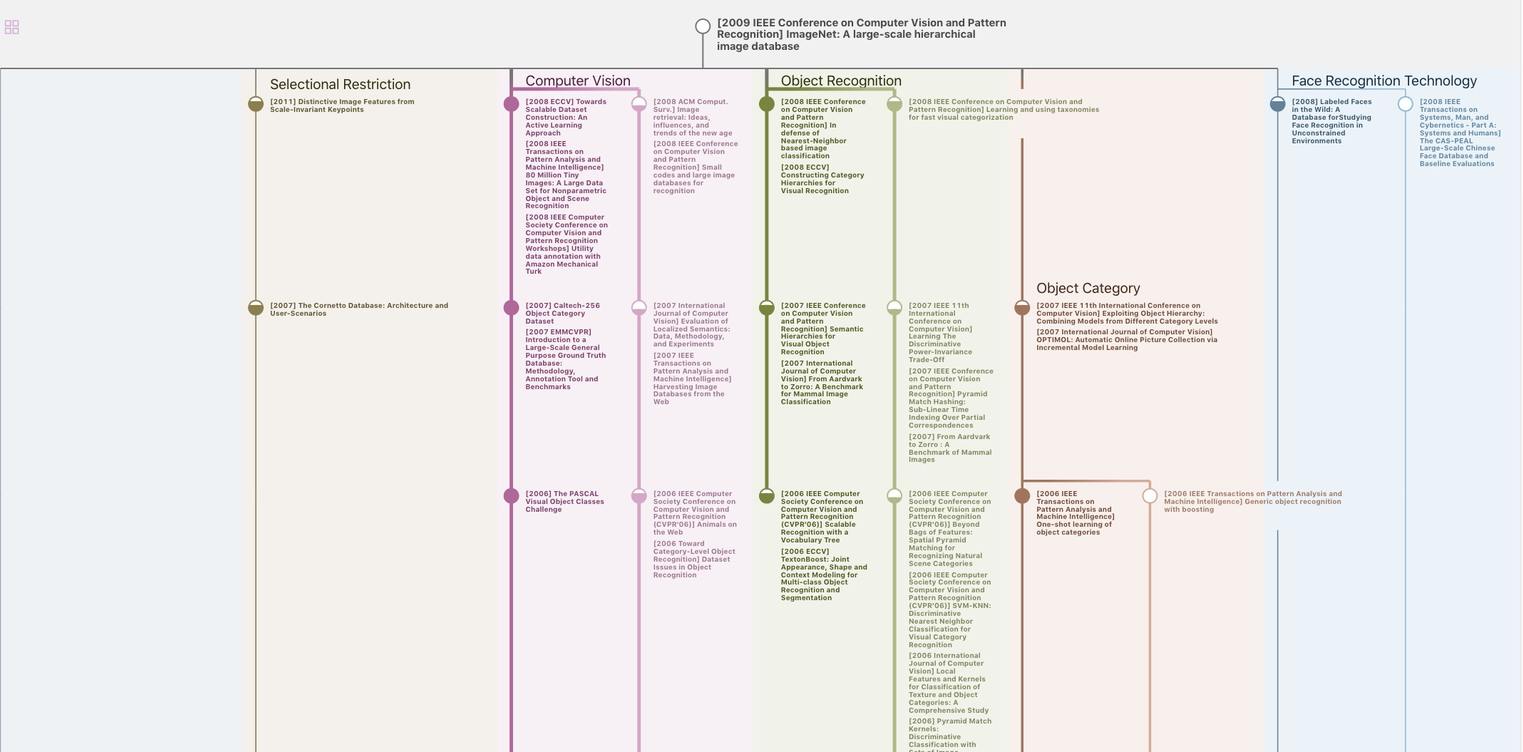
生成溯源树,研究论文发展脉络
Chat Paper
正在生成论文摘要