Deep-Learning Optimized Reconfigurable Metasurface for Magnetic Resonance Imaging
2024 18th European Conference on Antennas and Propagation (EuCAP)(2024)
摘要
Reconfigurable metamaterials (MTMs) allow for manifold applications in Magnetic Resonance Imaging (MRI). Most importantly, the full control in the spatio-temporal domain enables the realization of arbitrary sensitivity patterns during the transmit (Tx) as well as the receive (Rx) phase of an MRI scan via (re-)shaping the magnetic field distribution. The optimization of linking the reconfigurable
$N$
degrees of freedom to a desired field profile., however., is a non-trivial problem. In this work., a one-dimensional prototype
$(N=14)$
is presented that is digitally controlled in combination with deep learning (DL)-driven optimization. Both., the forward and inverse problem are addressed. The forward problem., involving the mapping of capacitance values to a desired magnetic field distribution., is well-posed and can be effectively solved through a variety of methods. The inverse problem., i.e., deriving capacitance values from observed magnetic fields, is tackled by deep learning, specifically through a Multi-Layer Perceptrons (MLPs) approach. The feasibility of training a neural network with simulation data, achieving sufficient agreement between numerical and experimental results is demonstrated. Deep learning-driven optimization of MTMs in MRI applications holds a huge potential for many applications in the future and it represents a significant step towards bridging the gap between simulated and experimental results.
更多查看译文
AI 理解论文
溯源树
样例
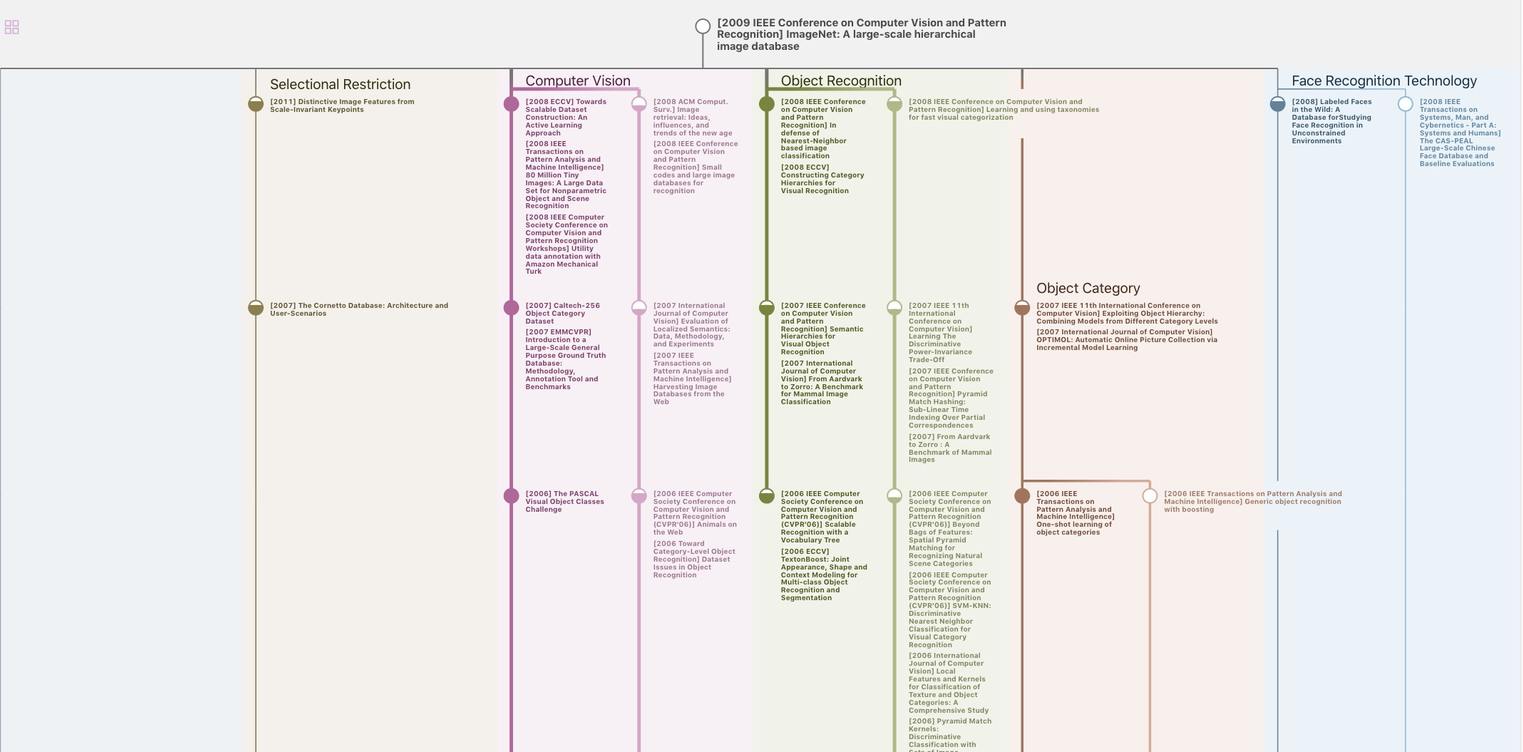
生成溯源树,研究论文发展脉络
Chat Paper
正在生成论文摘要