A Knowledge Graph Completion Algorithm Based on the Fusion of Neighborhood Features and vBiLSTM Encoding for Network Security
Electronics(2024)
摘要
Knowledge graphs in the field of network security can integrate diverse, heterogeneous, and fragmented network security data, further explore the relationships between data, and provide support for deep analysis. Currently, there is sparse security information in the field of network security knowledge graphs. The limited information provided by traditional text encoding models leads to insufficient reasoning ability, greatly restricting the development of this field. Starting from text encoding, this paper first addresses the issue of the inadequate capabilities of traditional models using a deep learning model for assistance. It designs a vBiLSTM model based on a word2vec and BiLSTM combination to process network security texts. By utilizing word vector models to retain semantic information in entities and extract key features to input processed data into BiLSTM networks for extracting higher-level features that better capture and express their deeper meanings, this design significantly enhances understanding and expression capabilities toward complex semantics in long sentences before inputting final feature vectors into the KGC-N model. The KGC-N model uses feature vectors combined with graph structure information to fuse forward and reverse domain features and then utilizes a Transformer decoder to decode predictions and complete missing information within the network security knowledge map. Compared with other models using evaluation metrics such as MR, MRR demonstrates that employing our proposed method effectively improves performance on completion tasks and increases comprehension abilities toward complex relations, thereby enhancing accuracy and efficiency when completing knowledge graphs.
更多查看译文
关键词
cybersecurity,knowledge graph completion,text encoding,vBiLSTM,KGC-N
AI 理解论文
溯源树
样例
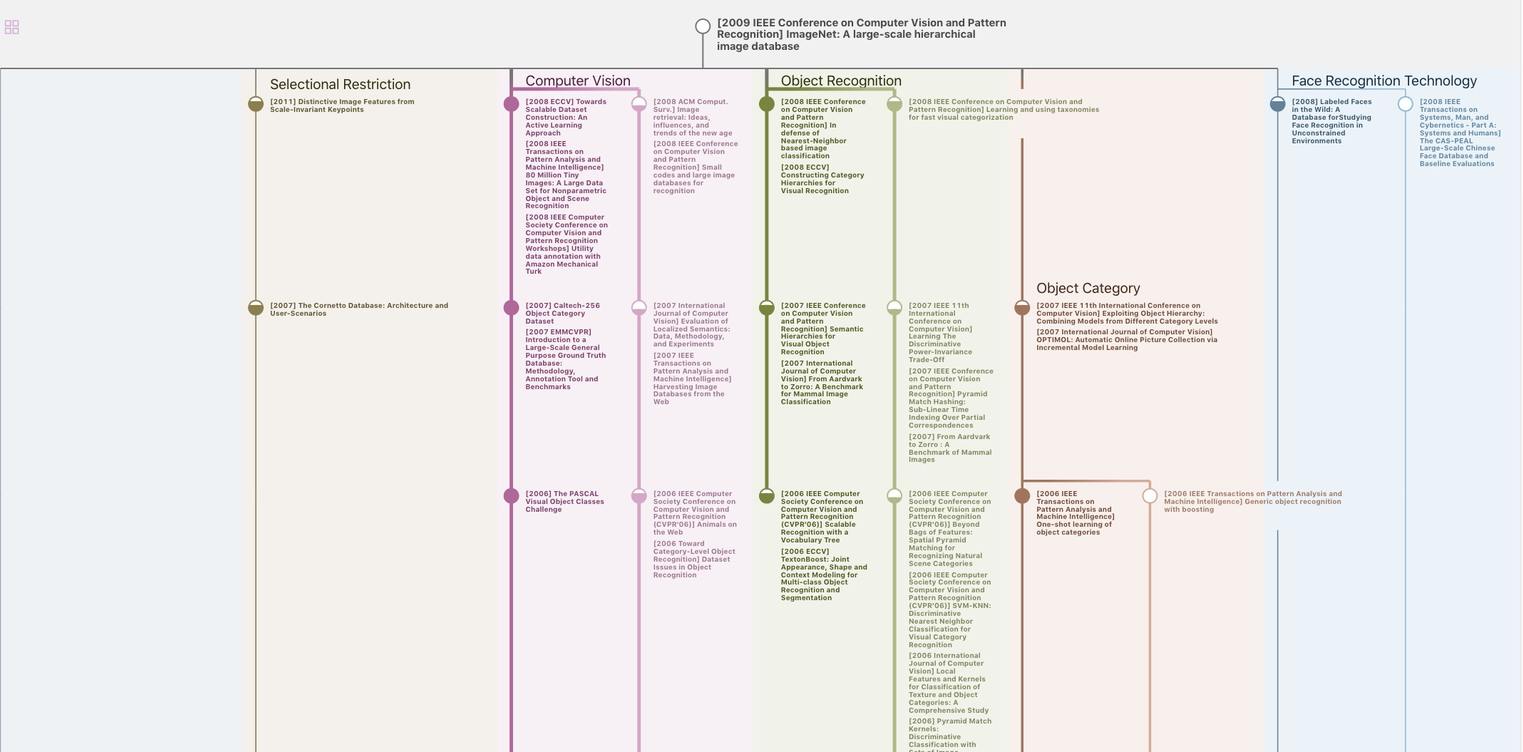
生成溯源树,研究论文发展脉络
Chat Paper
正在生成论文摘要