RS-BERT: Pre-training radical enhanced sense embedding for Chinese word sense disambiguation
Information Processing & Management(2024)
摘要
Word sense disambiguation is a crucial task to test whether a model can perform deep understanding. Nowadays, the rise of pre-trained language models facilitates substantial success in such tasks. However, most current pre-training tasks are taken in place of the token level while ignoring the linguistic sense of the tokens themselves. Thus it is questionable whether the token-predicting objectives are enough to learn the polysemy and disambiguate senses. To explore this question, we introduce RS-BERT, a radical enhanced sense embedding model with a novel pre-training objective, sense-aware language modeling, which introduces additional sense-level information to the model. For each training step, we first predict the senses and then update the model given the predicted senses. During training, we alternately perform the above two steps in an expectation–maximization manner. Besides, we also introduce radical information to RS-BERT at the beginning of pre-training. We conduct experiments on two Chinese word sense disambiguation datasets. Experimental results show that RS-BERT is competitive. When combined with other dedicated adaptions for specific datasets, RS-BERT shows impressive performance. Moreover, our analysis shows that RS-BERT successfully clusters Chinese characters into various senses. The experiment results demonstrate that the token-predicting objectives are not enough and the sense-level objective performs better for polysemy and sense disambiguation.
更多查看译文
关键词
Sense embeddings,Language models,Word sense disambiguation
AI 理解论文
溯源树
样例
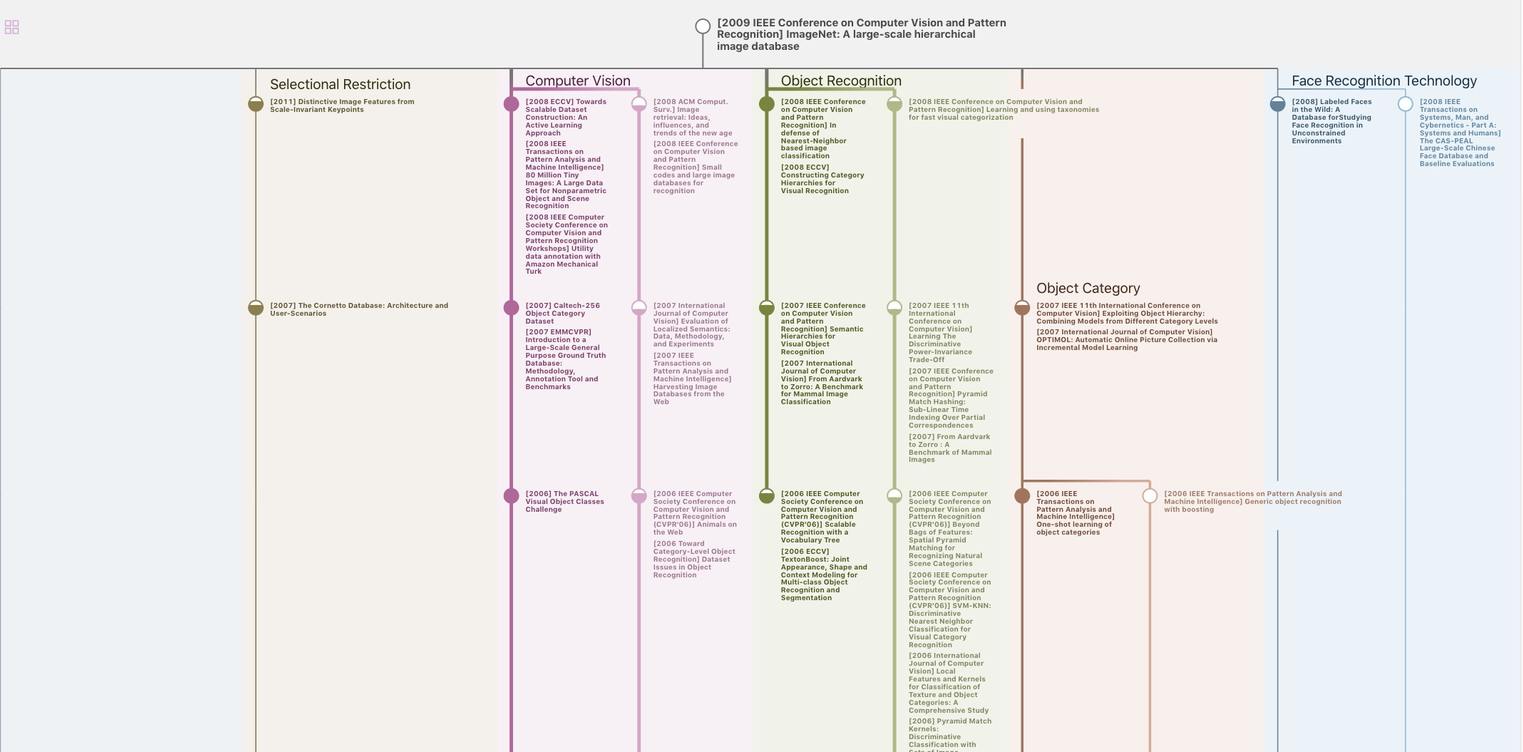
生成溯源树,研究论文发展脉络
Chat Paper
正在生成论文摘要