SSA-Deep Learning Forecasting Methodology with SMA and KF Filters and Residual Analysis
Mathematical and Computational Applications(2024)
摘要
Accurate forecasting remains a challenge, even with advanced techniques like deep learning (DL), ARIMA, and Holt–Winters (H&W), particularly for chaotic phenomena such as those observed in several areas, such as COVID-19, energy, and financial time series. Addressing this, we introduce a Forecasting Method with Filters and Residual Analysis (FMFRA), a hybrid methodology specifically applied to datasets of COVID-19 time series, which we selected for their complexity and exemplification of current forecasting challenges. FMFFRA consists of the following two approaches: FMFRA-DL, employing deep learning, and FMFRA-SSA, using singular spectrum analysis. This proposed method applies the following three phases: filtering, forecasting, and residual analysis. Initially, each time series is split into filtered and residual components. The second phase involves a simple fine-tuning for the filtered time series, while the third phase refines the forecasts and mitigates noise. FMFRA-DL is adept at forecasting complex series by distinguishing primary trends from insufficient relevant information. FMFRA-SSA is effective in data-scarce scenarios, enhancing forecasts through automated parameter search and residual analysis. Chosen for their geographical and substantial populations and chaotic dynamics, time series for Mexico, the United States, Colombia, and Brazil permitted a comparative perspective. FMFRA demonstrates its efficacy by improving the common forecasting performance measures of MAPE by 22.91%, DA by 13.19%, and RMSE by 25.24% compared to the second-best method, showcasing its potential for providing essential insights into various rapidly evolving domains.
更多查看译文
关键词
filtering time series,residual analysis time series,singular spectrum analysis,LSTM,convolutional neural networks,COVID-19
AI 理解论文
溯源树
样例
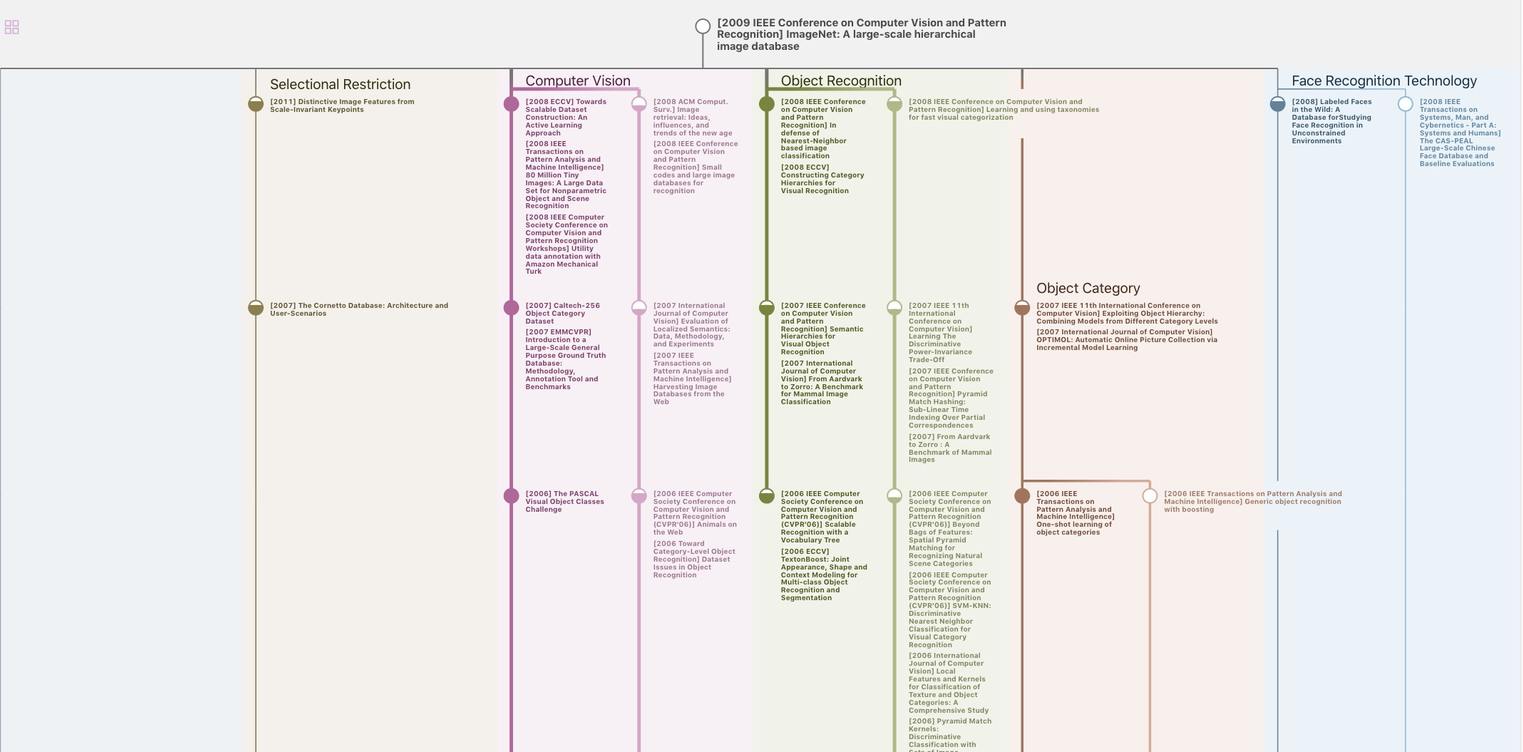
生成溯源树,研究论文发展脉络
Chat Paper
正在生成论文摘要