Sparsity-Constrained Vector Autoregressive Moving Average Models for Anomaly Detection of Complex Systems with Multisensory Signals
Mathematics(2024)
摘要
Detecting anomalies in large, complex systems is a critical and challenging task, and this is especially true for high-dimensional anomaly detection due to the underlying dependency structures among sensors. To incorporate the interrelationships among various sensors, a novel sparsity-constrained vector autoregressive moving average (scVARMA) model is proposed for anomaly detection in complex systems with multisensory signals. This model aims to leverage the inherent relationships and dynamics among various sensor readings, providing a more comprehensive and accurate analysis suitable for complex systems’ complex behavior. This research uses convex optimization to search for a parameterization that is sparse based on the principal of parsimony. This sparse model will not only contribute to meeting the real-time requirements of online monitoring strategies but also keeps the correlations among different sensory signals. The performance of the proposed scVARMA model is validated using real-world data from complex systems. The results affirm the superiority of the proposed scheme, demonstrating its enhanced performance and potential in practical applications.
更多查看译文
关键词
complex systems,sparsity-constrained,time series prediction,vector autoregressive moving average
AI 理解论文
溯源树
样例
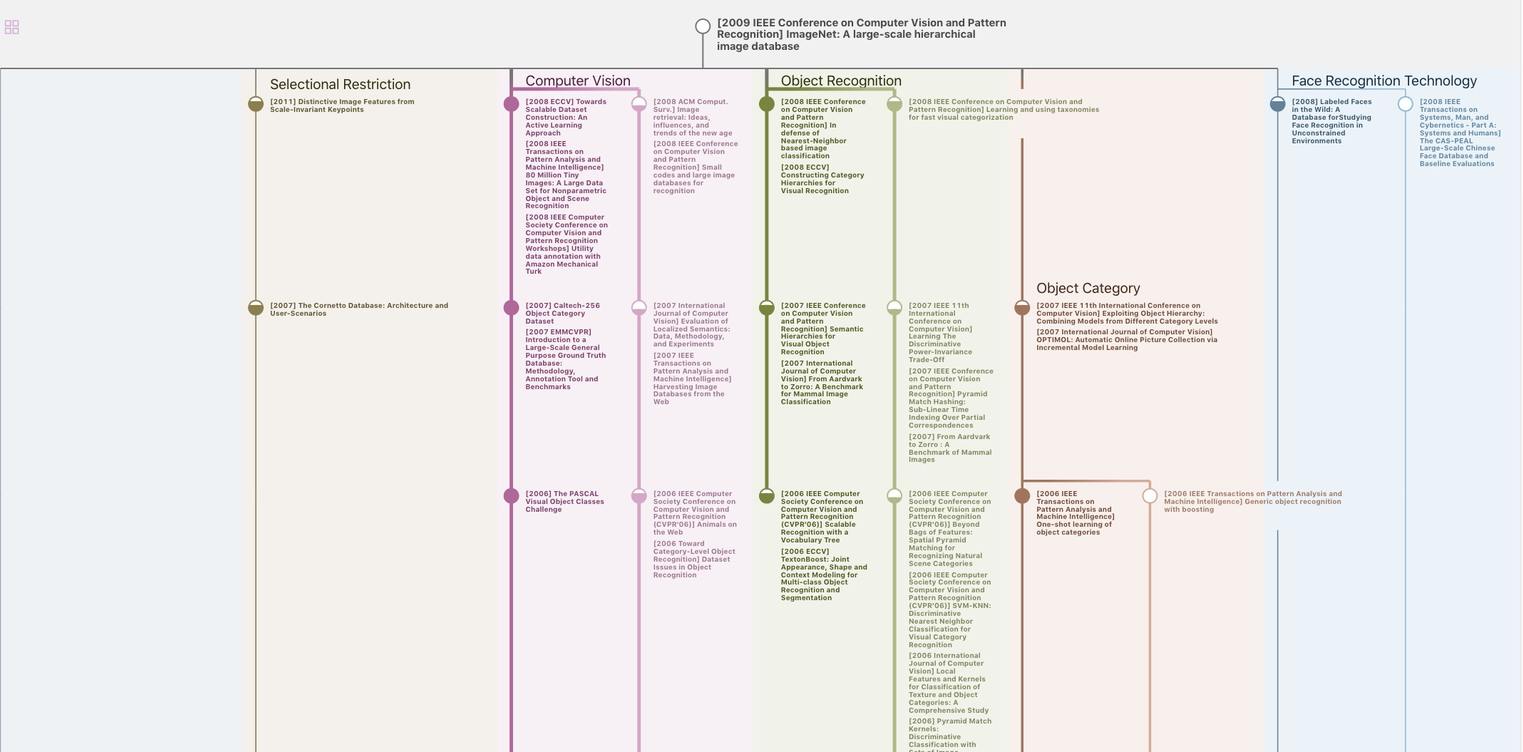
生成溯源树,研究论文发展脉络
Chat Paper
正在生成论文摘要