Conjoint Cross-attention Modeling and Joint Feature Calibrating for Remote Sensing Image Change Detection via a Triple-Double Network
IEEE Transactions on Geoscience and Remote Sensing(2024)
摘要
Remote sensing (RS) image change detection (CD) based on deep learning (DL), has received increasing attention recently. However, the general independent learning of bi-temporal images ignores the relationship between them, falling short in learning of the change information. In this paper, a Triple-Double (TD) framework with ability of conjoint cross-attention modeling and joint feature calibrating is proposed for CD. Specifically, the TD framework composed of Triple-branch encoder and Double-branch decoder is constructed to extract diverse features and acquire changed maps with the guidance of original edge cues. To enhance the perception of the connection between the bi-temporal features, the multi-scale difference guidance (MDG) module and conjoint cross-attention (CCA) module are designed for the dual-branch encoder, wherein the CCA introduces a novel and efficient rule for modeling the affinity in spatial and channel dimension simultaneously. Furthermore, a joint feature calibration (JFC) module is introduced to enhance the expression of feature diversity in the joint features within the single-branch encoder. Experimental results on three public datasets demonstrate the superiority of the proposed method compared to the state-of-the-art (SOTA) methods.
更多查看译文
关键词
Change detection,remote sensing,triple-double framework,conjoint cross-attention module,joint feature calibration
AI 理解论文
溯源树
样例
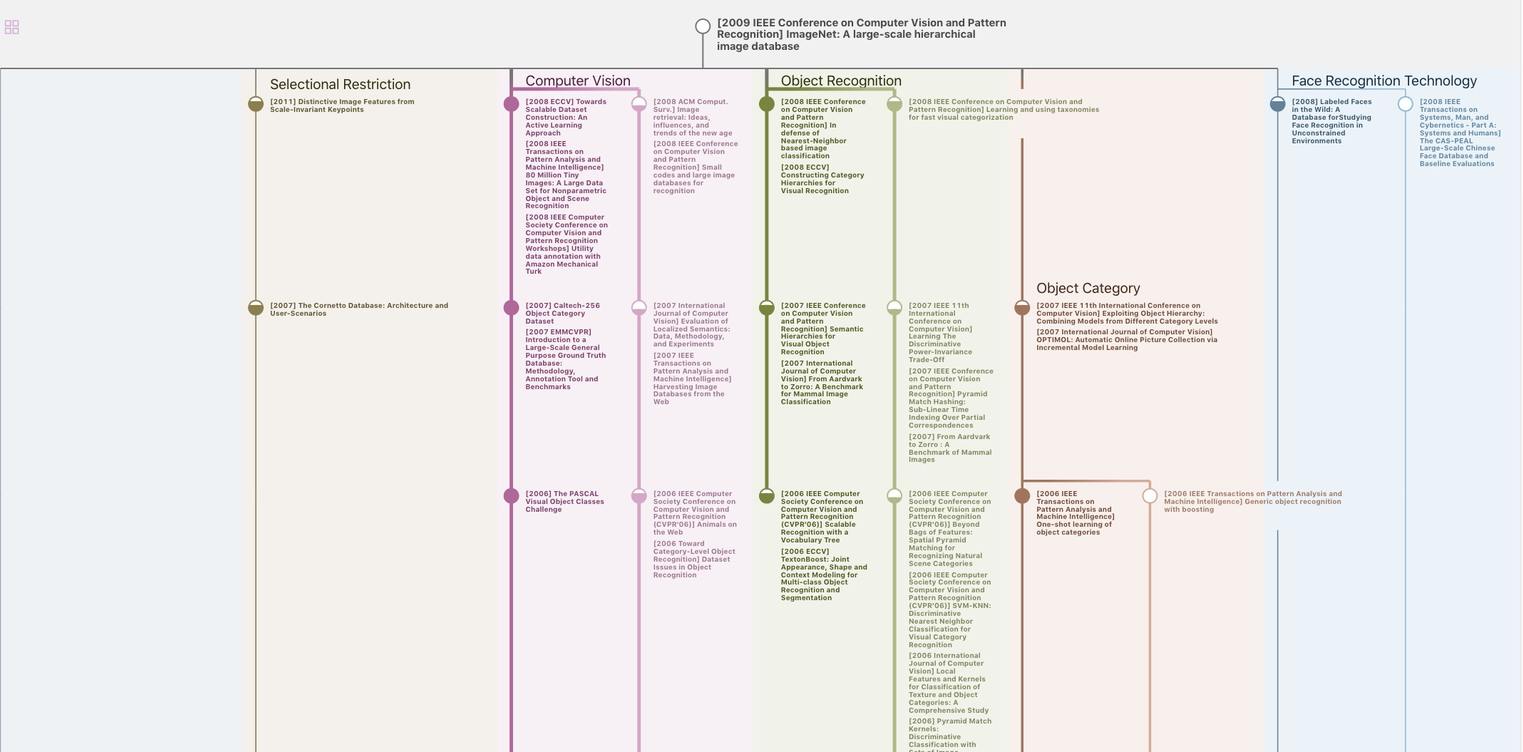
生成溯源树,研究论文发展脉络
Chat Paper
正在生成论文摘要