Searching for Functional Neuromarkers of Multiple Sclerosis: A Geodesic Distance-Based Framework on Riemannian Manifolds of Brain Connectivity
SoutheastCon 2024(2024)
摘要
Multiple Sclerosis (MS), a dynamic and dispersing disease that not only reflects the structural damages but also causes functional connectivity (FC) imbalance in and between brain networks of patients. Identifying novel neuromarkers for this disease remains a crucial challenge in brain connectivity analysis. To address this, we propose a two-stage mathematical framework capable of discriminating between healthy subjects and MS patients. Our proposed method leverages the properties of positive-definiteness of covariance-based connectivity matrices, which reside on a Riemannian manifold. It allows us to define and utilize a geodesic distance metric within this space. To construct a meaningful vector description for classification and neuromarker identification, we encode the data utilizing a set of reference networks established through geodesic clustering of FC matrices. These cluster centroids (reference networks) act as a dictionary, that enables us to encode each subject's connectivity matrix as a vector of geodesic distance. Finally, we employ a logistic regression classifier to differentiate between healthy and MS groups. A sensitivity analysis of trained classifier weights allowed the identification of the best discriminating feature and therefore led us to the identification of novel neuromarkers that best discriminate between the two groups. We demonstrate that our approach can successfully, discriminate between two groups and also identify neurophysiological markers of disease severity.
更多查看译文
关键词
connectomes,functional connectivity,dominant-set,geodesic clustering,Riemannian manifold
AI 理解论文
溯源树
样例
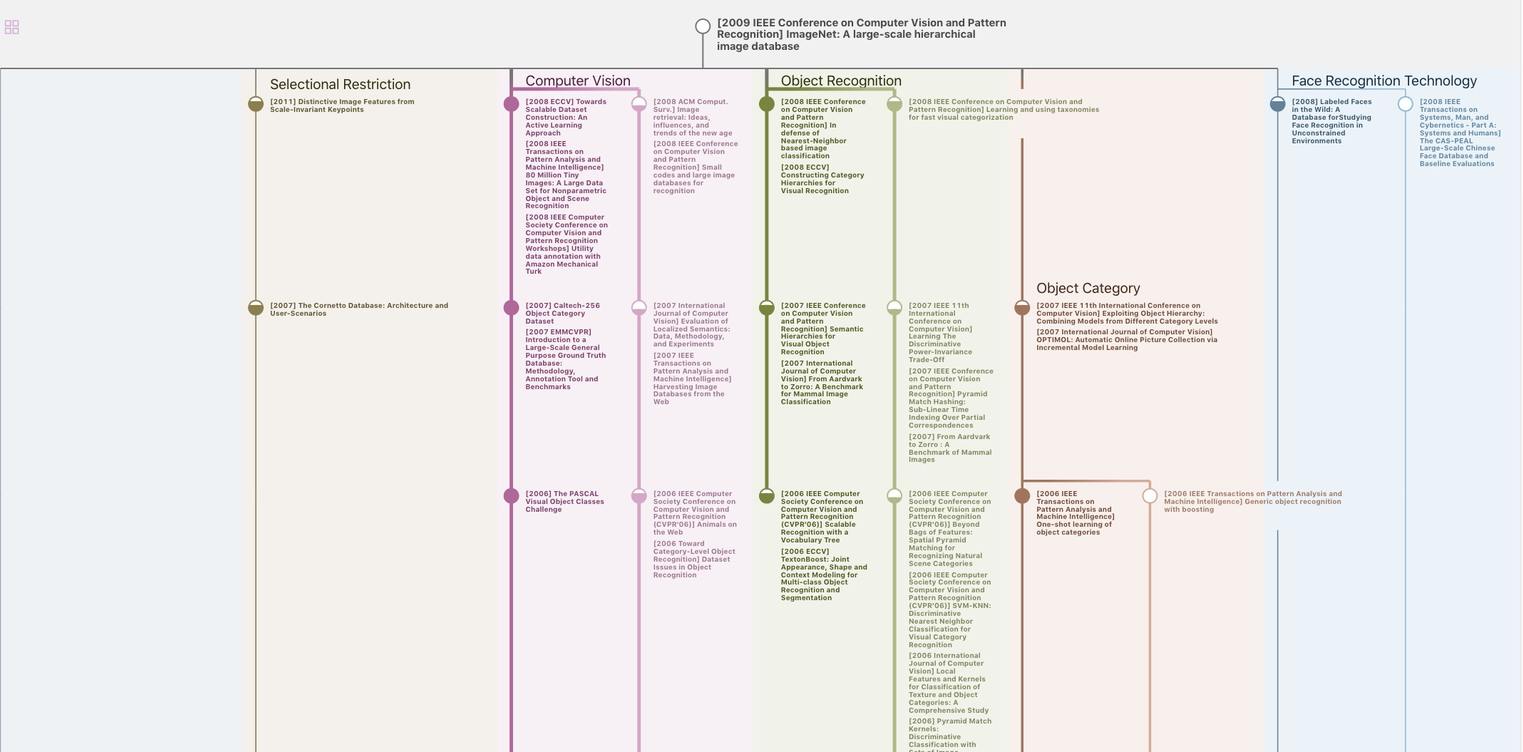
生成溯源树,研究论文发展脉络
Chat Paper
正在生成论文摘要