Differentially-Private Federated Learning with Non-IID Data for Surgical Risk Prediction
2024 IEEE First International Conference on Artificial Intelligence for Medicine, Health and Care (AIMHC)(2024)
摘要
Federated learning (FL) has emerged as a promising solution to deal with the privacy concerns which often limit access to data for training machine learning (ML) models. It encompasses the exchange of model parameters between data owners and a central model aggregation instance without the need to share sensitive data directly. Although FL does not require sharing sensitive information, it may not guarantee complete privacy, as model parameters can potentially reveal sensitive details about the underlying training data. To enhance privacy, FL approaches can additionally integrate differential privacy (DP), which entails the careful addition of noise to the model. In this paper, we investigate the relationship between FL, DP, and highly non-independent and identically distributed (non-IID) data. We present a specific real-life healthcare application of predicting patient mortality and revision surgery after visceral operations. Our contributions encompass assessing the performance of FL with and without DP in the presence of highly non-IID data, analysing the fairness for individual training participants and investigating the efficacy of personalisation to alleviate the privacy-utility trade-off introduced by DP. Our findings indicate that the addition of DP into FL given non-IID datasets disparately favours participants with larger datasets, while the model has limited utility for those with less data. The addition of a subsequent model fine-tuning step can remedy this disparity and enables all participants to reach a model performance comparable to the centralised training scenario.
更多查看译文
关键词
Federated learning,Differential privacy,Machine learning,Clinical risk prediction
AI 理解论文
溯源树
样例
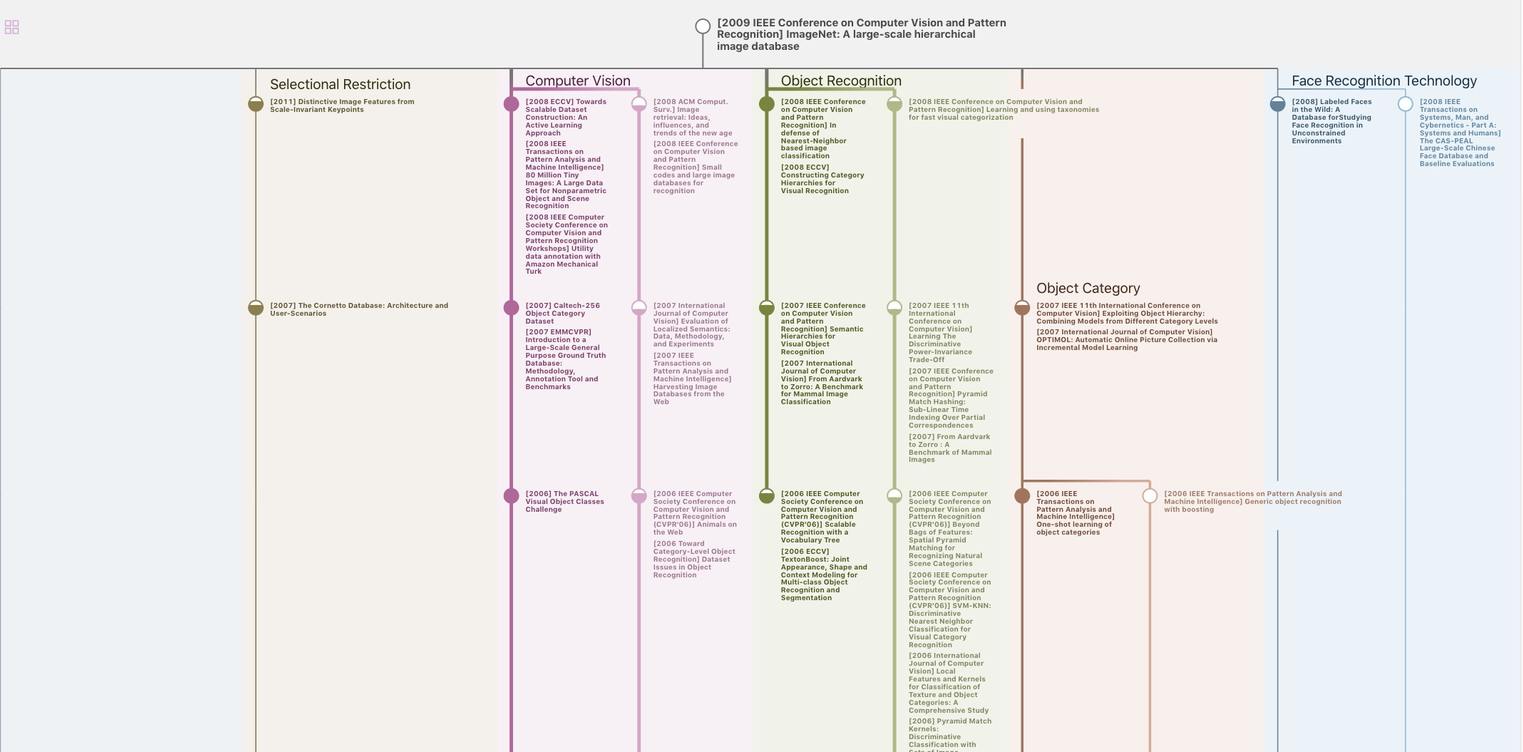
生成溯源树,研究论文发展脉络
Chat Paper
正在生成论文摘要