Cancerous and Non-Cancerous MRI Classification Using Dual DCNN Approach
Bioengineering(2024)
摘要
Brain cancer is a life-threatening disease requiring close attention. Early and accurate diagnosis using non-invasive medical imaging is critical for successful treatment and patient survival. However, manual diagnosis by radiologist experts is time-consuming and has limitations in processing large datasets efficiently. Therefore, efficient systems capable of analyzing vast amounts of medical data for early tumor detection are urgently needed. Deep learning (DL) with deep convolutional neural networks (DCNNs) emerges as a promising tool for understanding diseases like brain cancer through medical imaging modalities, especially MRI, which provides detailed soft tissue contrast for visualizing tumors and organs. DL techniques have become more and more popular in current research on brain tumor detection. Unlike traditional machine learning methods requiring manual feature extraction, DL models are adept at handling complex data like MRIs and excel in classification tasks, making them well-suited for medical image analysis applications. This study presents a novel Dual DCNN model that can accurately classify cancerous and non-cancerous MRI samples. Our Dual DCNN model uses two well-performed DL models, i.e., inceptionV3 and denseNet121. Features are extracted from these models by appending a global max pooling layer. The extracted features are then utilized to train the model with the addition of five fully connected layers and finally accurately classify MRI samples as cancerous or non-cancerous. The fully connected layers are retrained to learn the extracted features for better accuracy. The technique achieves 99%, 99%, 98%, and 99% of accuracy, precision, recall, and f1-scores, respectively. Furthermore, this study compares the Dual DCNN’s performance against various well-known DL models, including DenseNet121, InceptionV3, ResNet architectures, EfficientNetB2, SqueezeNet, VGG16, AlexNet, and LeNet-5, with different learning rates. This study indicates that our proposed approach outperforms these established models in terms of performance.
更多查看译文
关键词
deep learning,magnetic resonance imaging,classification,machine learning,deep convolutional neural networks,dual DCNN model
AI 理解论文
溯源树
样例
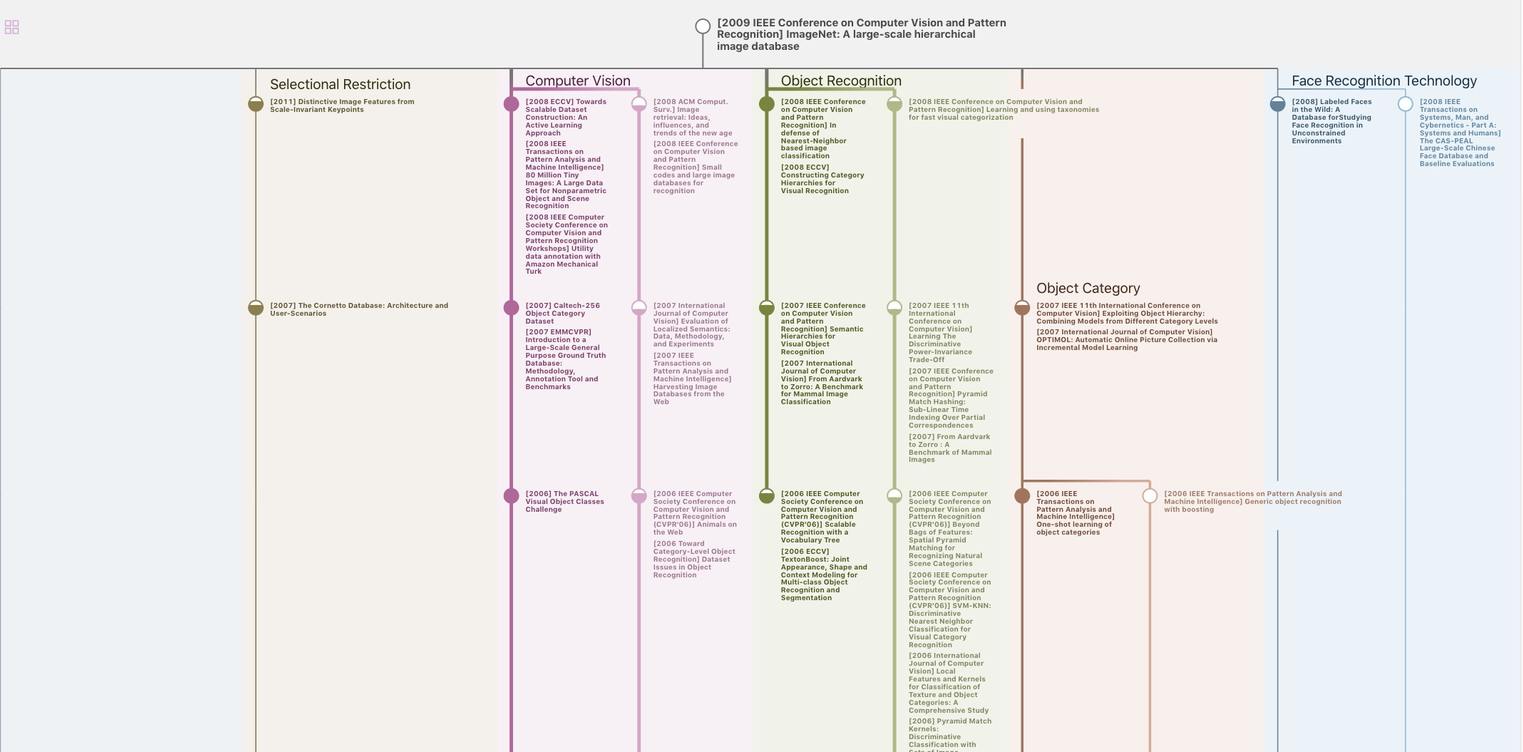
生成溯源树,研究论文发展脉络
Chat Paper
正在生成论文摘要