Class Incremental Learning With Deep Contrastive Learning and Attention Distillation
IEEE Signal Processing Letters(2024)
摘要
Class incremental learning can solve the issue of catastrophic forgetting when the trained model is used to learn a new task in which the model may forget part of previous knowledge learned. The key issue is to alleviate the stability-plasticity dilemma and maintain the balance between preventing old knowledge from being forgotten and learning new knowledge. In this paper, a new class incremental learning method with deep contrastive learning and attention distillation is proposed. The deep contrastive learning can optimize last layer of the model to learn strong-semantic information and the intermediate layers to learn weak-semantic information when new data is coming so that the plasticity can be improved. To ensure a balance between plasticity and stability, a new attention distillation approach is developed to learn and distill the latent attention information of the feature maps and the similarity between different samples. Our analysis and experimental results indicate that the proposed method can improve the learning and memorization ability and the average accuracy of the model compared with the previous methods, and obtain competitive results in class increment scenarios for image classification.
更多查看译文
关键词
Class Incremental Learning,Deep Contrastive Learning,Attention Information,Knowledge Distillation
AI 理解论文
溯源树
样例
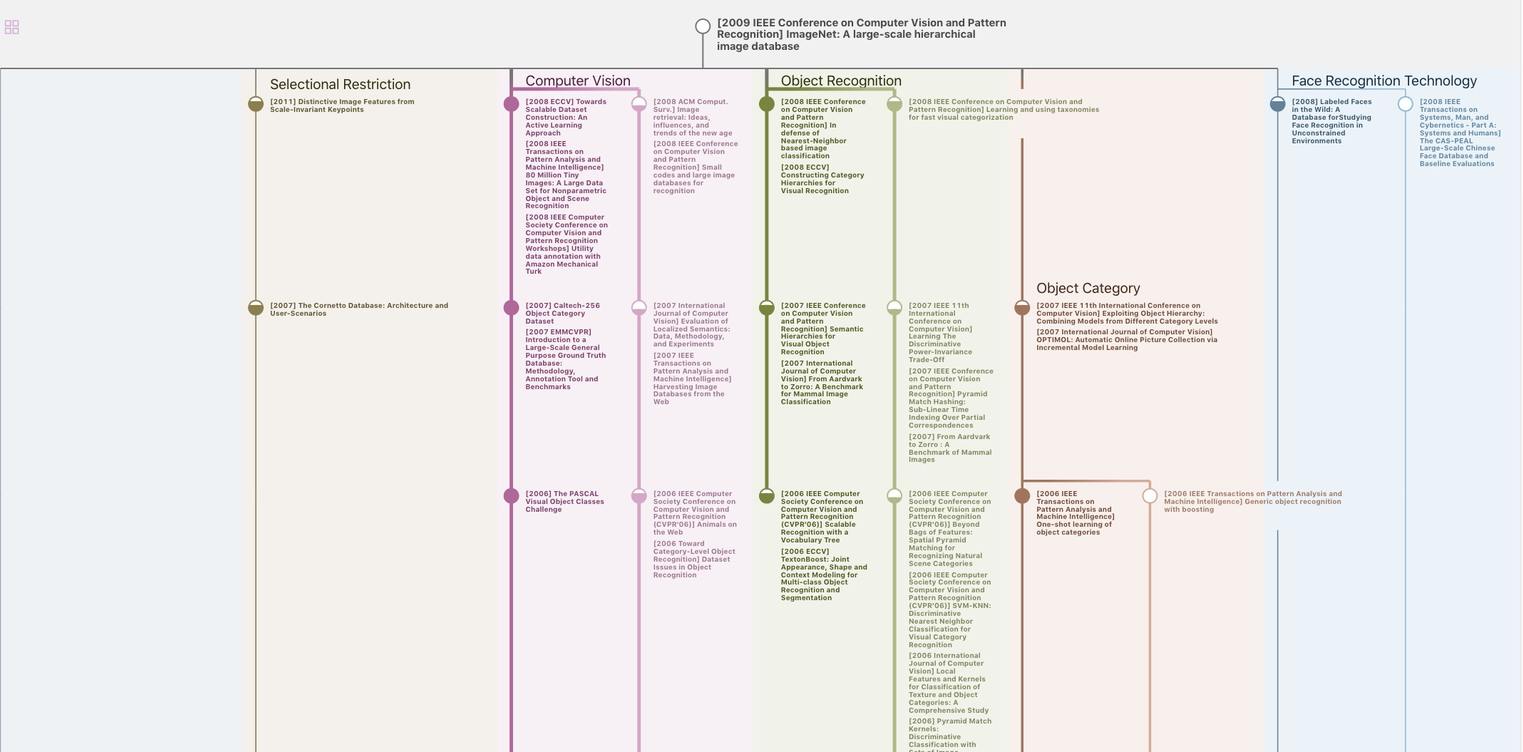
生成溯源树,研究论文发展脉络
Chat Paper
正在生成论文摘要