Memristor-based hardware accelerators for artificial intelligence
Nature Reviews Electrical Engineering(2024)
摘要
Satisfying the rapid evolution of artificial intelligence (AI) algorithms requires exponential growth in computing resources, which, in turn, presents huge challenges for deploying AI models on hardware. Memristor-based hardware accelerators provide a promising solution to the energy efficiency and latency issues in large AI model deployments. The non-volatility of memristive devices facilitates in-memory computing, in which computing occurs within memory cells where data are stored. This approach eliminates the constant data shuttling between the processing and memory units found in the von Neumann architecture, resulting in substantial time and energy savings. The recent surge of research and development in this field indicates a pivotal transition of memristor technology from proof-of-concept demonstrations to commercial products that accelerate AI models across various applications. In this Review, we survey the latest progress in memristive crossbar arrays, peripheral circuits, architectures, hardware–software co-designs and system implementations for memristor-based hardware accelerators. We discuss how these research efforts bridge the gap between memristive devices and energy-efficient accelerators for AI. Finally, we summarize the key remaining issues and propose potential pathways to future hardware accelerators with low latency and high energy efficiency, emphasizing the technology scale-up and commercialization for large-scale AI applications. This Review summarizes latest advancements in memristor-based hardware accelerators, an energy-efficient solution for computing-intensive artificial intelligence algorithms, covering crossbar arrays, peripheral circuits, architectures and software–hardware co-designs. It analyses challenges and pathways for the transition of memristor technology to commercial products.
更多查看译文
AI 理解论文
溯源树
样例
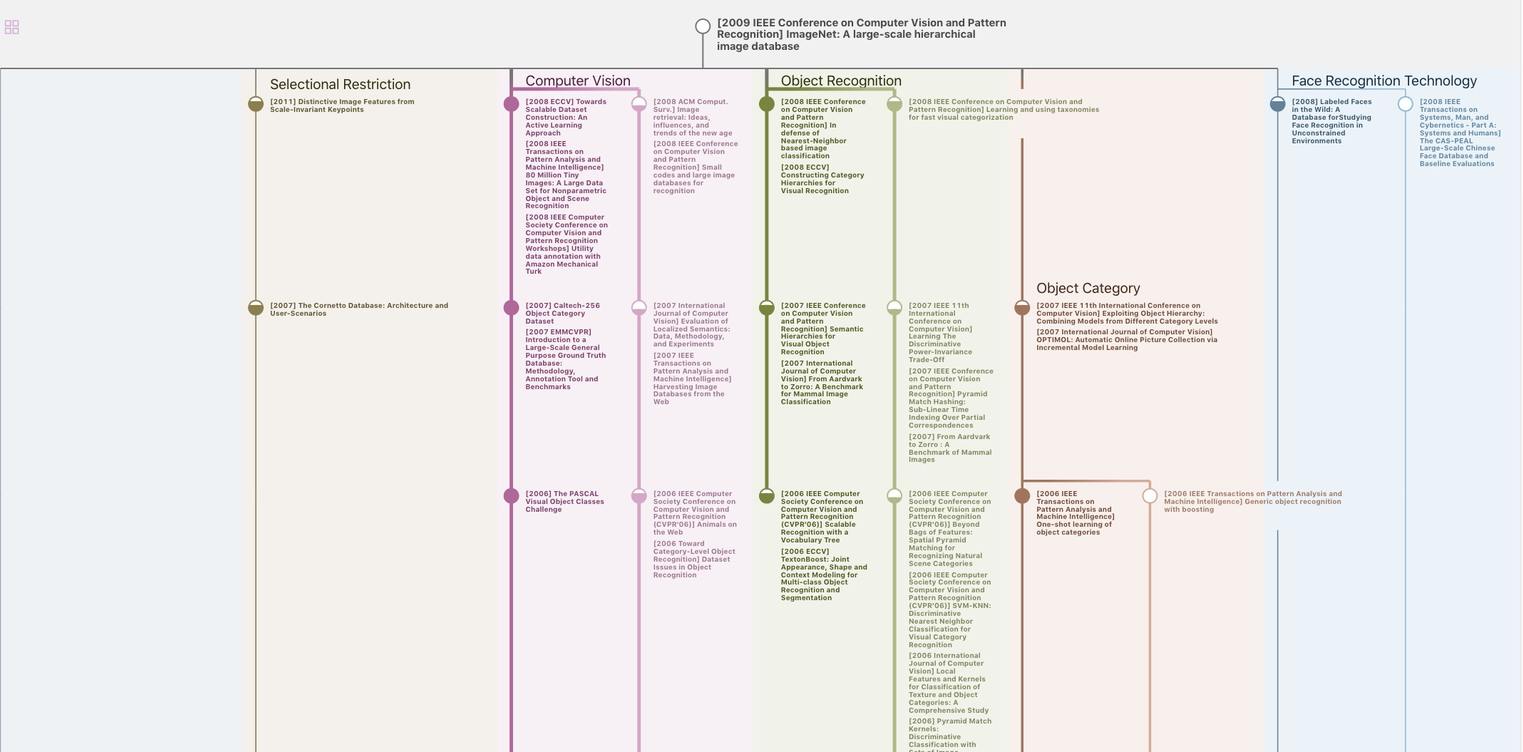
生成溯源树,研究论文发展脉络
Chat Paper
正在生成论文摘要