Cross-Dataset Continual Learning: Assessing Pre-Trained Models to Enhance Generalization in HAR.
Annual IEEE International Conference on Pervasive Computing and Communications(2024)
摘要
Pervasive computing has profoundly transformed the way in which companies provide and develop innovative services across various sectors. In the healthcare domain, for instance, smartphones equipped with sensors can be used to collect data to enhance health diagnostics and analysis. Using such data in conjunction with Machine Learning (ML) models for Human Activity Recognition (HAR) has gained significant attention, as it offers promising avenues for healthcare innovation and personalized services. However, traditional ML models often struggle to adapt to evolving data streams over time. To address this issue, the introduction of Continual Learning (CL) has become crucial, ensuring that models can accumulate knowledge over time and continually improve their performance in dynamic environments. This, however, raises several major issues related, for example, to catastrophic forgetting as well as to the size of the datasets. Here, the typical size of HAR datasets is relatively small, which can be an issue when conducting training in CL from scratch. To mitigate this challenge, starting the CL process with pre-trained models has emerged as a promising strategy. In this context, the purpose of this paper is twofold. First, we analyze the impact of conducting CL on a target dataset when starting with a pre-trained model initially built with limited data from a similar dataset. Furthermore, we investigate the effect of using a model pre-trained on a large dataset on the CL process conducted on a smaller target dataset. Our experiments on the UCI HAR and the USC HAD datasets showed that CL significantly improves model accuracy when starting with a pre-trained model with limited initial data. However, the choice of the pre-trained model and dataset for CL is crucial. Using a pre-trained model from more complex dataset can lead to better CL accuracy when moving to a simpler dataset.
更多查看译文
关键词
Incremental Learning,Human Activity Recognition,Accuracy Of Model,Machine Learning Models,Traditional Machine Learning,Similar Datasets,Target Dataset,Traditional Machine Learning Models,Simple Datasets,Catastrophic Forgetting,Continuous Learning Process,Training Data,Knowledge Base,Convolutional Neural Network,Convolutional Layers,Accelerometer,Generative Adversarial Networks,Convolutional Neural Network Model,Convolutional Neural Network Architecture,Task Accuracy,Small Amount Of Data,Pre-training Process,Previous Tasks,Current Task,Variational Autoencoder,Pre-training Phase,Domain Adaptation,Challenging Dataset
AI 理解论文
溯源树
样例
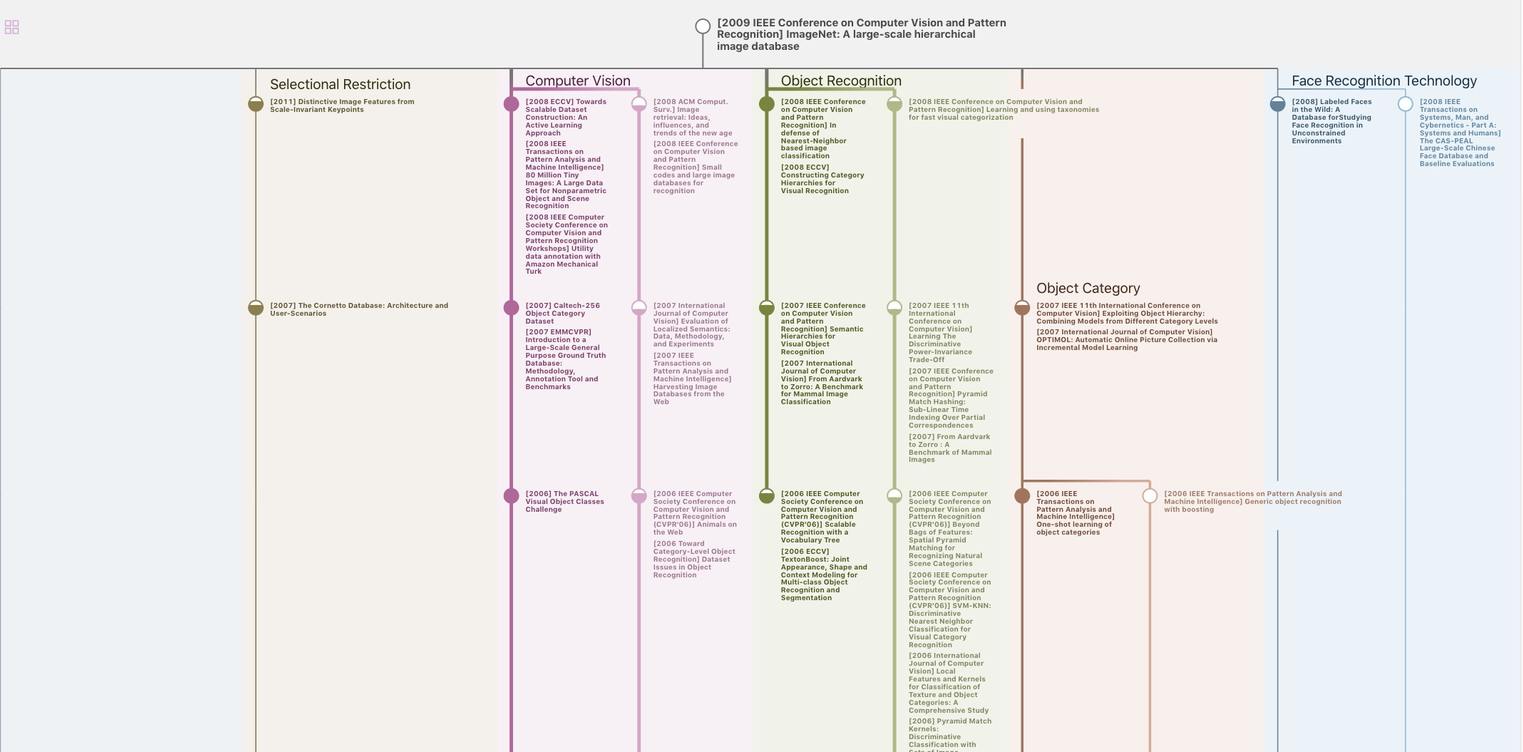
生成溯源树,研究论文发展脉络
Chat Paper
正在生成论文摘要