Volume-Wise Decoding of Human Brain's Task fMRI via Deep Learning
2024 4th International Conference on Neural Networks, Information and Communication (NNICE)(2024)
摘要
In recent years, significant progress has been made in decoding human cognitive tasks from functional magnetic resonance imaging (fMRI) images using deep learning methods. However, existing approaches predominantly rely on the assumption of temporal stationarity of neural activity, decoding static brain networks from task-based fMRI data at the block-wise. The study of dynamic brain networks poses challenges in terms of limited accuracy and complexity of research methods. This limitation hampers the development of neuroscience in achieving a broader and finer-grained understanding of human brain function across a wider range of temporal scales. To address this issue, we propose an volume-wise decoder for task fMRI, adopts end-to-end deep learning method. Compared with traditional machine learning methods, the decoding accuracy and efficiency are significantly improved. This method not only offers a more accurate and efficient decoding approach for a finer-grained comprehension of cognitive processes, but also offers new perspectives and insights into human cognition.
更多查看译文
AI 理解论文
溯源树
样例
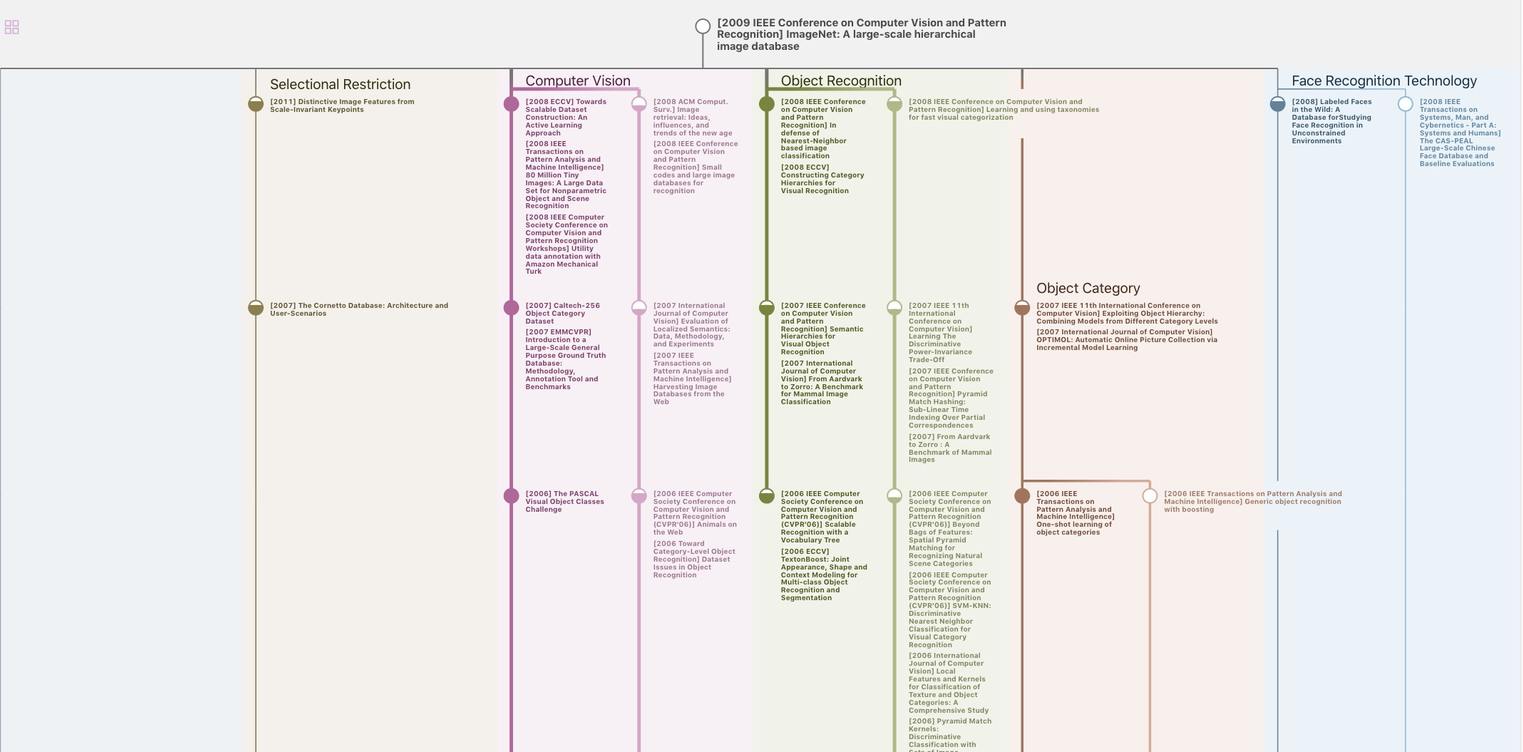
生成溯源树,研究论文发展脉络
Chat Paper
正在生成论文摘要