An interpretable model for sepsis prediction using multi-objective rule extraction
Journal of Intelligent Information Systems(2024)
摘要
Sepsis is a leading cause of death among intensive care unit patients. Early sepsis prediction, which primarily relies on advanced artificial intelligence technology, is an important phase in sepsis management. In previous sepsis prediction research, tremendous time and effort are spent in building high accurate tree ensemble models. However, due to the “black box” nature of tree ensemble models, clinicians typically reluctant to use them in clinical practice. To fill the gap, a novel multi-objective rule extraction method is proposed to trade off the predictive performance and the interpretability of tree ensemble model for sepsis prediction. The proposed method encompasses two phases: rule extraction utilizing a novel multi-objective binary differential evolution algorithm (MOBDE), and rule simplification employing a greedy heuristic method. In addition, rule extraction incorporates a Tchebycheff-based competitive learning strategy and a binary mutation operation aimed at enhancing the search ability of MOBDE. Rule simplification leveraging the proposed greedy heuristic method, streamlines the extracted rules from the previous phase by eliminating redundant constraints. The 2019 PhysioNet/CinC Challenge dataset, a well-known dataset in sepsis prediction, is used to evaluate the effectiveness of the proposed method. The experimental results show that the proposed method is more interpretable and has higher accuracy than some of commonly used rule-based models for sepsis prediction. The proposed method can be popularized to practical sepsis treatment, and assist doctors in sepsis diagnosis with the comprehensible decision-making support.
更多查看译文
关键词
Decision-making,Interpretable model,Rule extraction,Multi-objective optimization,Sepsis prediction
AI 理解论文
溯源树
样例
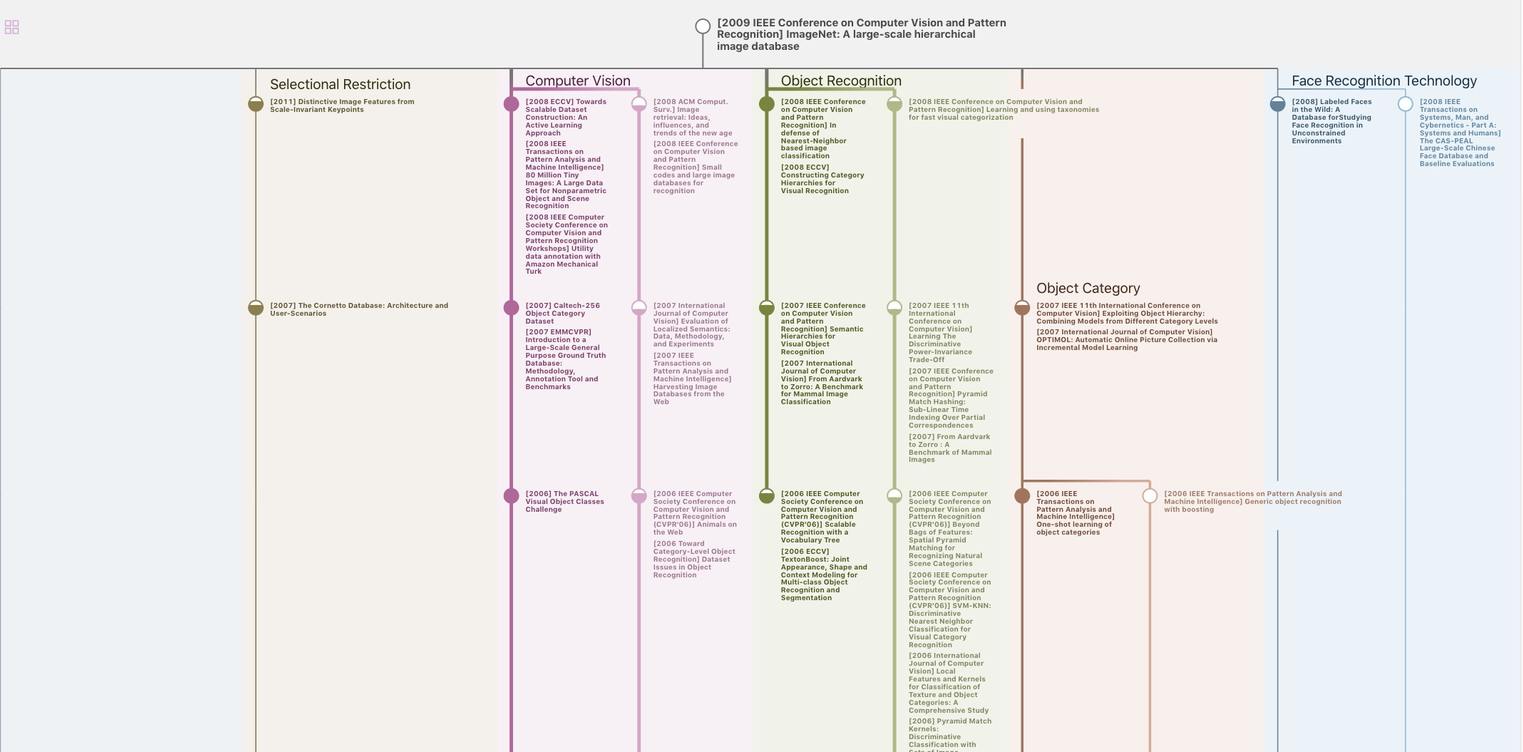
生成溯源树,研究论文发展脉络
Chat Paper
正在生成论文摘要