Multi-view Subspace Clustering Based on Weighted Tensor Schatten-P Norm
Journal of Physics: Conference Series(2024)
摘要
Abstract In the era of advancing technology and continuous societal evolution, an abundance of voluminous data from various domains has become prevalent in the public domain. Managing this wealth of data necessitates a series of preprocessing procedures, encompassing tasks such as data storage, computation, and analysis, with the aim of unveiling intrinsic features and patterns contained within. Among these procedures, clustering stands out as a crucial step, with the primary goal of unveiling the hidden structures within the data and organizing data samples into meaningful clusters based on specific criteria. Early clustering efforts were based on a singleview. Real world data, however, often contains interrelated information, leading to the emergence of multi-view clustering. Multiview subspace clustering (T-SVD) has been widely used in recent years. However, the equal treatment of each singular value in this approach lacks relevance when the data is noisy or contaminated. In order to improve the robustness and clustering performance, this paper presents a T-SVD weighted tensor norm. In order to improve the robustness, a Schatten-P norm was proposed. This paper explores multi-view subspace clustering is based on the Schatten-P norm weighted tensor. Experiments are carried out on 6 benchmark data sets, and the results are compared with 4 existing ones. Experiments show that the proposed approach is better than the most advanced multi-view subspace clustering methods.
更多查看译文
AI 理解论文
溯源树
样例
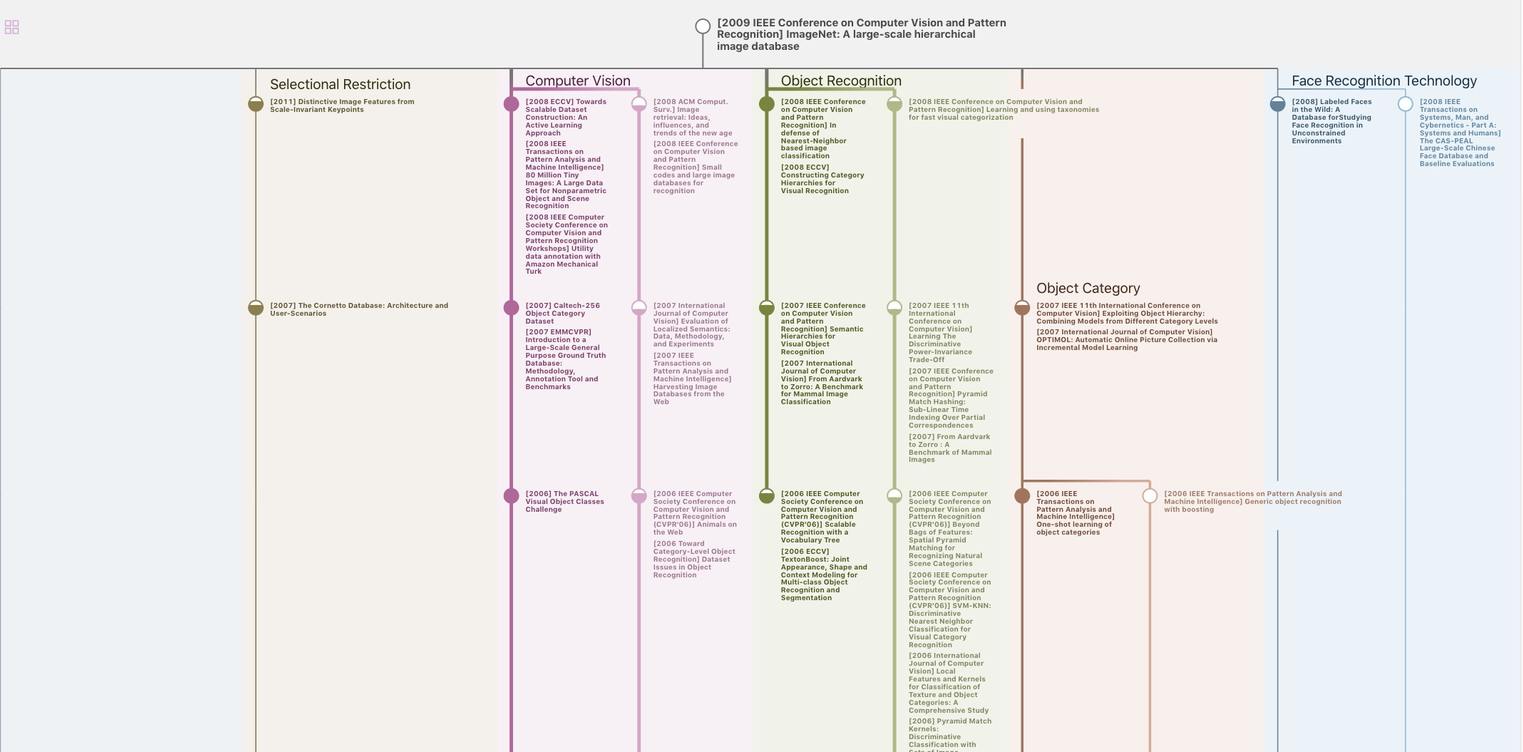
生成溯源树,研究论文发展脉络
Chat Paper
正在生成论文摘要