Dimensionality Reduction via Geometric Modeling of Topology-Optimized Devices for Machine Learning-based Inverse Design
2024 4th International Conference on Neural Networks, Information and Communication (NNICE)(2024)
摘要
Machine learning techniques have been increasingly applied in the inverse design of nanophotonics. A well-trained neural network can generate devices with diverse functions, obviating the requirement for repeated optimization processes for each type of devices. Topology optimization is a mainstream optimization strategy in inverse design, which excels in designing compact, high-performance devices, yet its high degree of freedom introduces challenges for the application of machine learning techniques. We present a novel scheme for adapting machine learning techniques into topology optimization of nanophotonic devices, breaking the convention where machine learning techniques were applied only in the design of devices with limited parameters. This scheme reduces the parameter dimension of topology-optimized devices from the order of 10
3
to 10
1
through a structure simplification process and B-spline geometric modeling, and subsequently utilizes a conditional generative network to generate devices with various functions in the new parameter space. As a demonstration, we present for the first time a set of T-junctions with continuous power splitting ratios ranging from 0 to 0.5, achieving transmissions exceeding 90% at 1550 nm across all ratios within a compact footprint of 2.8×2.8 μm
2
.
更多查看译文
关键词
dimensionality reduction,geometric modeling,invese design,machine learning,nanophotonics
AI 理解论文
溯源树
样例
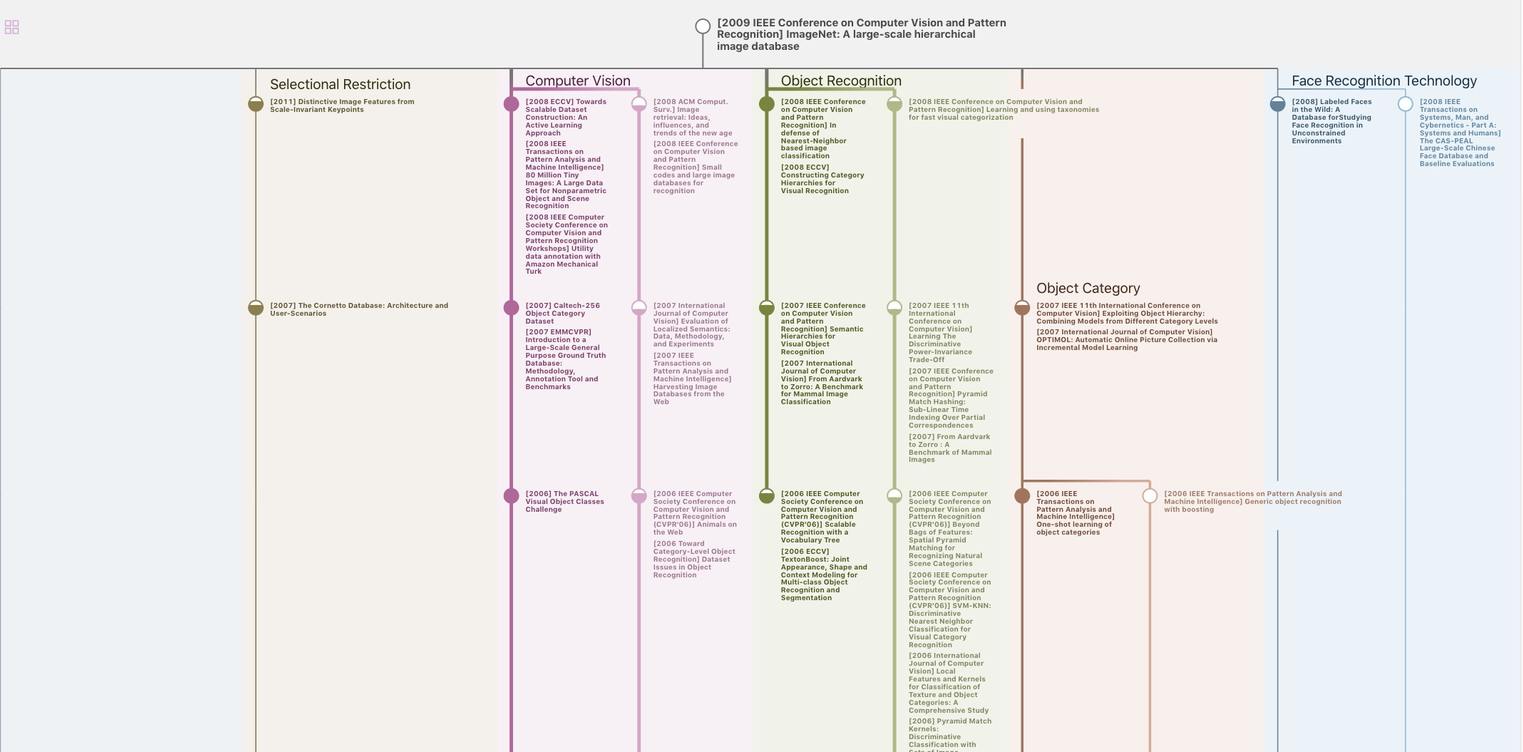
生成溯源树,研究论文发展脉络
Chat Paper
正在生成论文摘要