Dynamic Memristor based Cyclic Echo State Network for Time Series Prediction
2024 4th International Conference on Neural Networks, Information and Communication (NNICE)(2024)
摘要
Compared with traditional recurrent neural networks, the training cost of echo-state network computing is lower because the neurons in the reservoir are randomly connected. We only need to train the weight of the connection between the reservoir and the output layer. Due to its high accuracy and low training cost for time series forecasting tasks, it is widely used as a time series forecasting model in many fields. It is very important to provide rich reservoir state for echo state network system, which is the key to hardware implementation. In this paper, we propose a cyclic echo state network (DC-ESN) based on dynamic memristors, which generates rich reservoir states through cyclic structure, and can predict complex time series well even with a single memristor. Compared with the traditional echo state network, the size of the reservoir is reduced and the prediction accuracy is greatly improved. Our work can provide an idea for more efficient memristor based echo state network systems in the future to cope with complex time series prediction tasks.
更多查看译文
关键词
echo state network,memristor,time series prediction
AI 理解论文
溯源树
样例
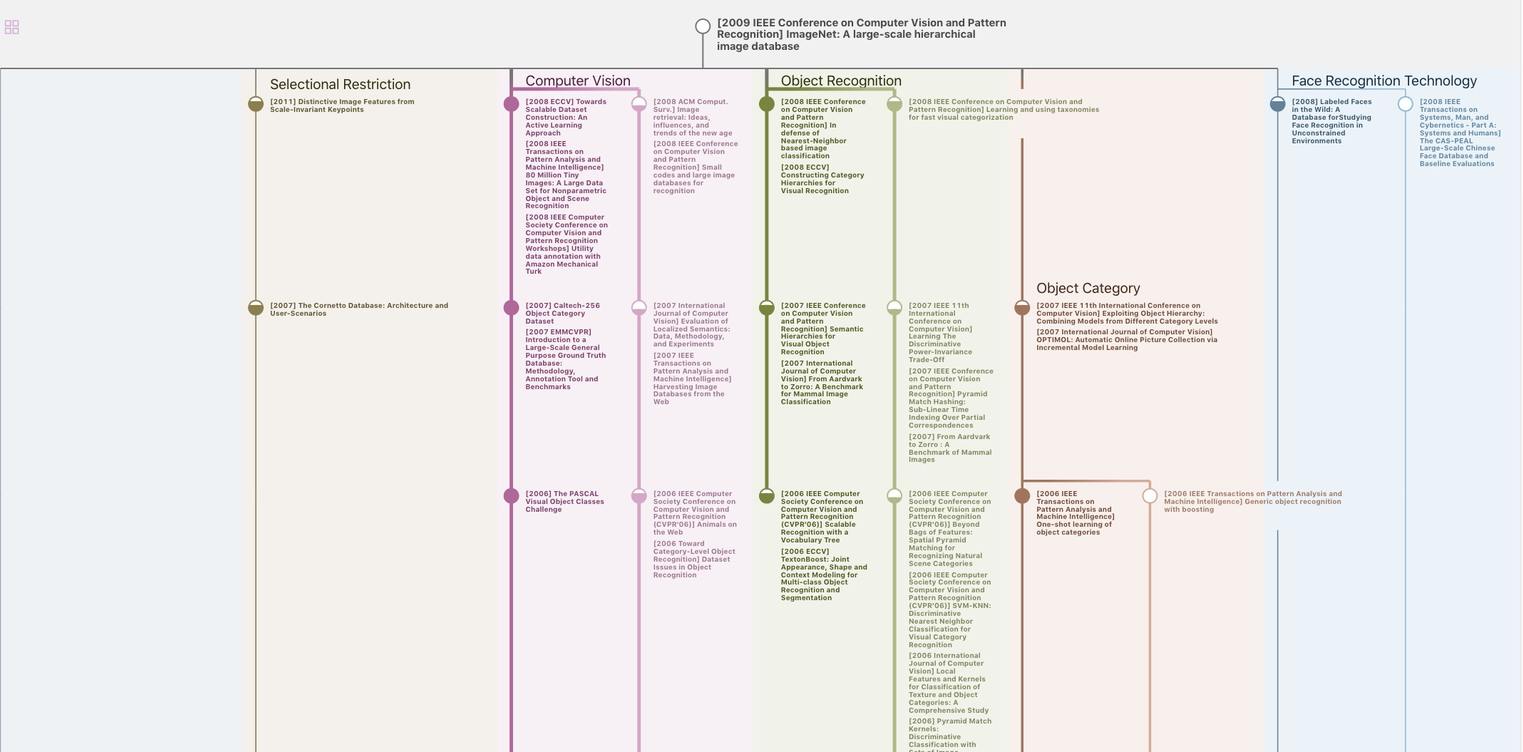
生成溯源树,研究论文发展脉络
Chat Paper
正在生成论文摘要