Gaze-infused BERT: Do human gaze signals help pre-trained language models?
Neural Computing and Applications(2024)
摘要
This research delves into the intricate connection between self-attention mechanisms in large-scale pre-trained language models, like BERT, and human gaze patterns, with the aim of harnessing gaze information to enhance the performance of natural language processing (NLP) models. We analyze the correlation between BERT attention and five distinct gaze signals based on the Spearman correlation, discovering that neither all attention layers nor all gaze signals accurately capture word importance. Building on this insight, we propose gaze-infused BERT, a novel model that integrates gaze signals into BERT for performance enhancement. Specifically, we first utilize a gaze prediction model based on RoBERTa to estimate five gaze signals, our lightweight model utilizes the entropy weight method (EWM) to generate a comprehensive gaze representation by combining five diverse gaze signals. This representation is then embedded into the transformer encoder while performing the self-attention between the input sequence, enriching contextual information and boosting performance. Extensive evaluations on the 15 datasets demonstrate that gaze-infused BERT consistently outperforms baseline models across various NLP tasks, highlighting the potential of integrating human gaze signals into pre-trained language models.
更多查看译文
关键词
Pre-trained language model,Gaze signals,Self-attention,Entropy weight method
AI 理解论文
溯源树
样例
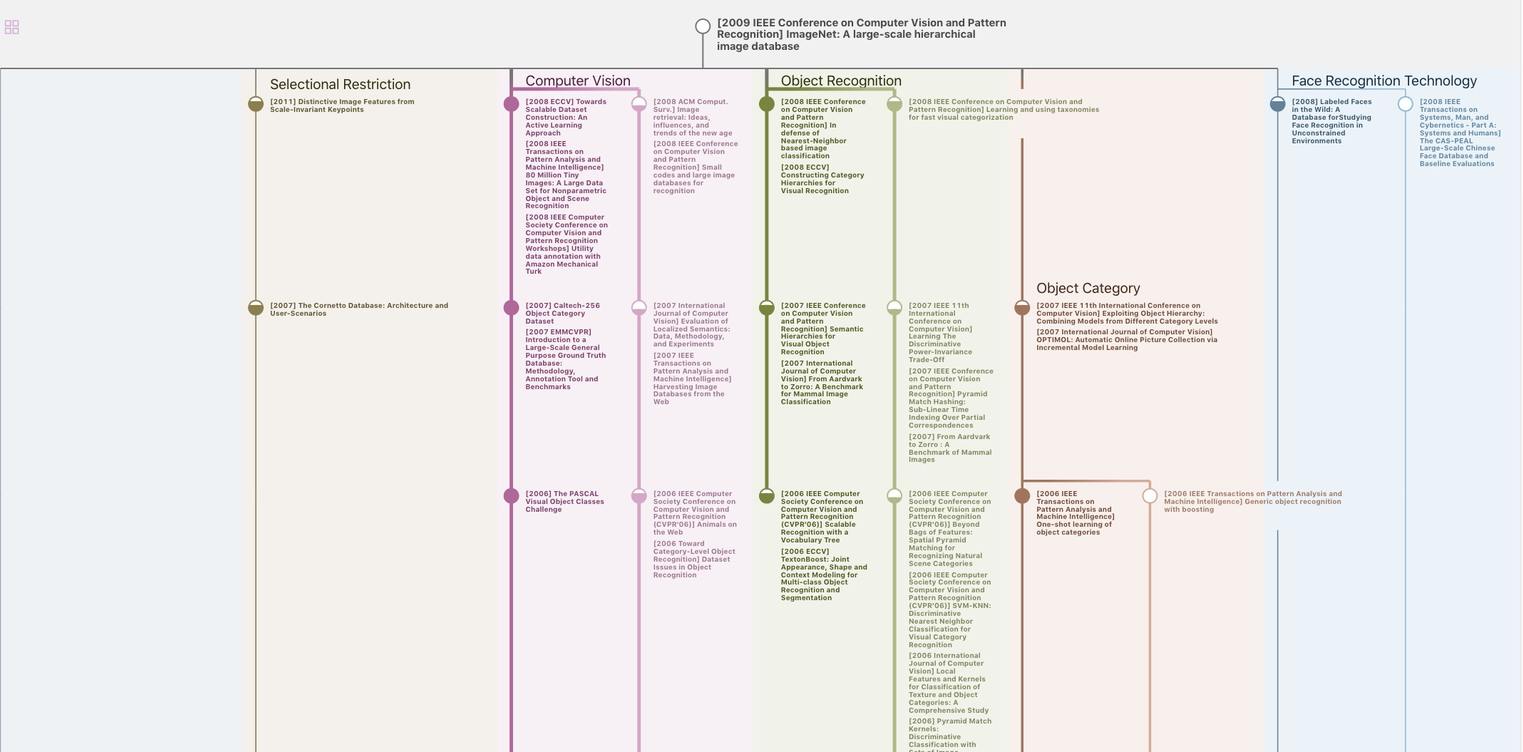
生成溯源树,研究论文发展脉络
Chat Paper
正在生成论文摘要