0297 Comparing Validation Metrics of Machine Learning Algorithms for Actigraphy Data in Children
SLEEP(2024)
摘要
Abstract Introduction Actigraphy methods are evolving to use machine learning algorithms for sleep health estimation, but most algorithms have been trained on adult data. It is not known if such algorithms can be used for sleep/wake estimation in children. We therefore leveraged machine learning models, trained with adult data for sleep prediction, to determine their validity in a sample of children. Methods We enrolled 30 children (14 female, 8-16y) referred for in-lab overnight polysomnography at Children’s Hospital of Philadelphia. Participants wore a GENEActiv device (a 3-axis accelerometer, set at 50 Hz sampling rate) on their non-dominant wrist while completing their overnight sleep test. Machine learning models trained using adult data by Walch et al. were applied to the accelerometer data, and aligned into 30-second epochs against the sleep stages from polysomnography scored by a sleep medicine physician. We used the F1 score, a harmonic average of sensitivity and precision, to rank the algorithms. Results Overall, 271.5 hours of polysomnography data were collected with 80% of the epochs scored as sleep. Sleep duration median was 7.0 hours (IQR = 2.0), WASO median was 39 minutes (IQR = 39), and sleep latency median was 48.5 minutes (IQR = 52.5). In rank order, the top average F1 scores were 0.91 (SD=0.04) for k-Nearest Neighbor (kNN), 0.86 (SD=0.05) for Neural Net, 0.83 (SD=0.05) for Logistic Regression, and 0.78 (SD=0.06) for Random Forest. Average sleep duration error was lowest for kNN (12.8 minutes [SD = 44.9]) and highest for Random Forest (-142.1 minutes [SD = 57.5]); the average WASO error was lowest for kNN (9.8 minutes [SD = 45.9]) and the highest for Random Forest (118.0 [SD = 58.9]); whereas the average sleep latency error was lowest for Random Forest (-3.4 minutes [SD = 27.6]) highest for kNN (-32.2 minutes [SD = 34. 8]). Conclusion Certain machine learning models trained with adult datasets performed well when applied to pediatric data, with kNN being the most optimal in accuracy. However, validity for sleep latency was the most optimal for Random Forest. Further development can potentially reduce the variation of these predictions with pediatric-data-based machine learning models. Support (if any)
更多查看译文
AI 理解论文
溯源树
样例
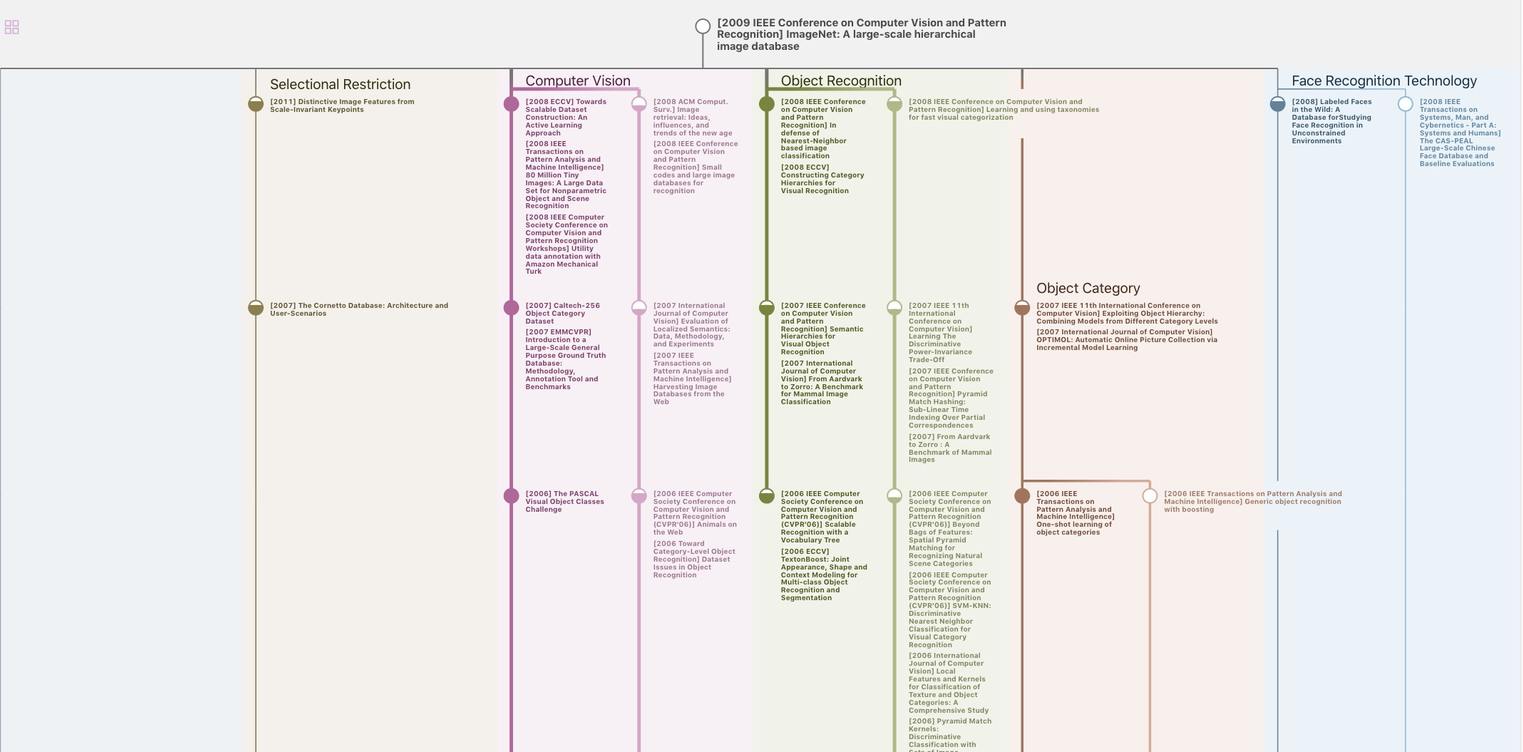
生成溯源树,研究论文发展脉络
Chat Paper
正在生成论文摘要