Field Robot Self-Exploration Based on Deep Reinforcement Learning and Safety Control Mechanism
crossref(2024)
摘要
Abstract This paper aims to address the issues that arise when using deep reinforcement learning algorithms for reactive robotic navigation in farmland environments. These challenges include complex network models, a large number of parameters, significant computational requirements, and the possibility of being vulnerable to local optimal solutions. Moreover, the complexity of the farmland environment poses a challenge to the existing indoor reactive navigation methods, which struggle to meet the requirements of tasks such as crop protection and human cooperation.To address these issues, the paper proposes the Field Robot Autonomous Navigation System (FRANS), which utilizes Deep Reinforcement Learning (DRL) to enable farmland robots to autonomously explore unknown environments. The system consists of two modules: Global Navigation (GN) and Local Navigation (LN). The GN module establishes goal points and implements a secure control mechanism to manage the robot's actions. This approach helps to address problems like locally optimal solutions. The LN module uses a lightweight DRL framework to develop a locally navigated motion strategy that guides the robot toward waypoints. FRANS does not require any prior knowledge and is capable of adjusting the navigation strategy in real time based on the current environment. The map is generated in real time as the robot moves through the environment. Experimental results demonstrate that FRANS outperforms similar approaches.
更多查看译文
AI 理解论文
溯源树
样例
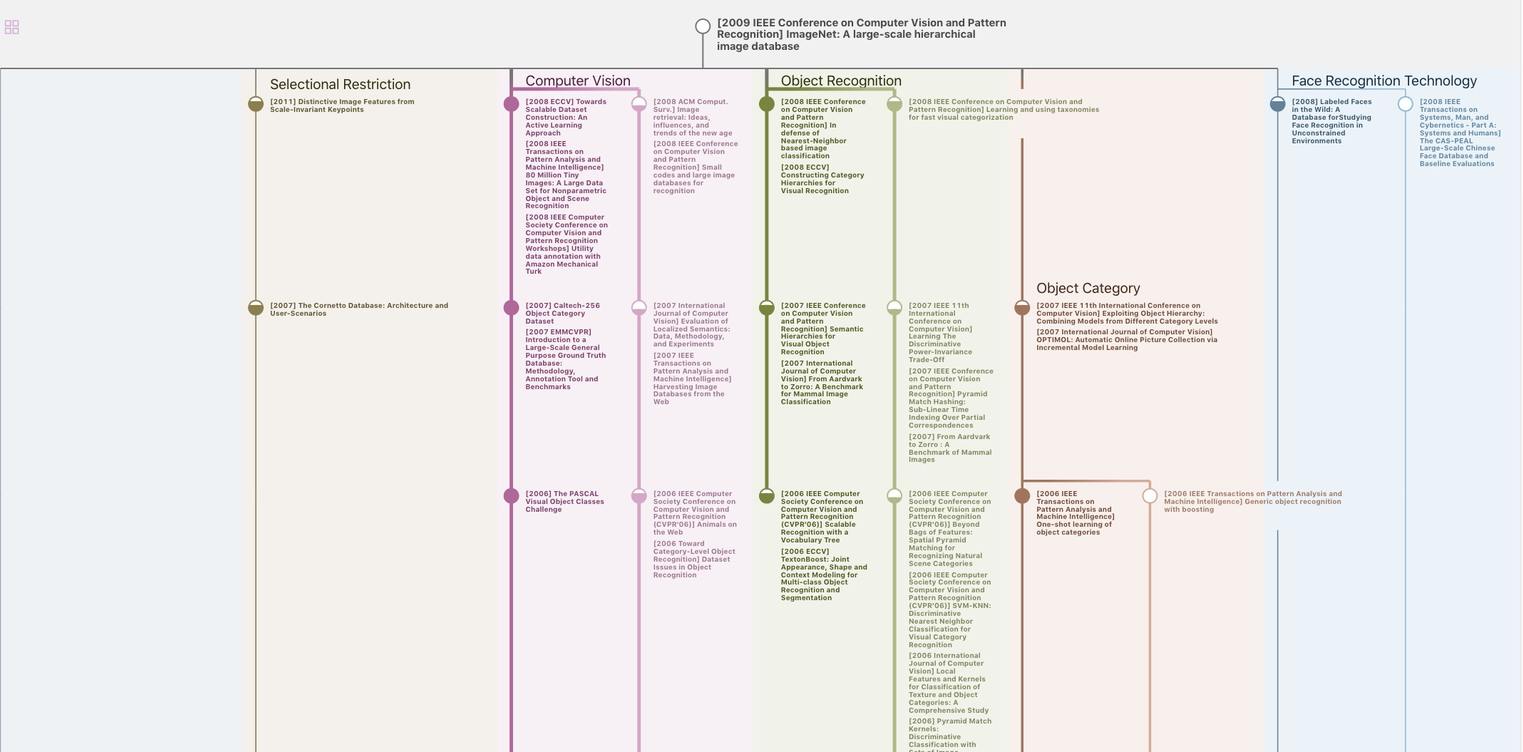
生成溯源树,研究论文发展脉络
Chat Paper
正在生成论文摘要