Performance evaluation and optimization of convolutional neural network architectures for Tomato plant disease eleven classes based on augmented leaf images dataset
Neural Computing and Applications(2024)
摘要
An efficient plant disease identification is prime important for Agricultural productivity. This paper proposes a method of automatic leaf extraction and classification with improved accuracy compared with existing techniques. Images acquired from natural fields are complex, with background information that needs to be accurately segmented to identify areas of interest. Grab cut is a semi-automatic approach to extract foreground objects, which might occasionally degrade an object’s properties. This paper suggests a better Grab cut for automatically extracting leaves from real-field images. The enhanced dataset of 23,617 images with eleven tomato leaf classes are created using the plant village dataset and actual field images. This paper addresses a wide range of issues related to convolutional neural network optimization, including how the number of layers affects the results of leaf disease detection and the creation of tiny models for portable devices. Five models, VGG 16, MobileNet, and custom architecture with input sizes of 98 × 98, 160 × 160, and 256 × 256, have been optimized and evaluated through several trials. Training and testing involve varying the input size, the number of layers, the optimizer, the dropout, the batch normalization, and the transfer learning. This paper presents and analyzes the findings of thirty experiments on various architecture. The proposed modified sequential eleven-layer architecture achieves accuracy compared with MobileNet and a reduced model size of 3.9 MB for input size 160 and 4.5 MB for input size 98.
更多查看译文
关键词
Agriculture,Plant diseases,Segmentation,Grab cut,Convolutional neural networks,Optimization
AI 理解论文
溯源树
样例
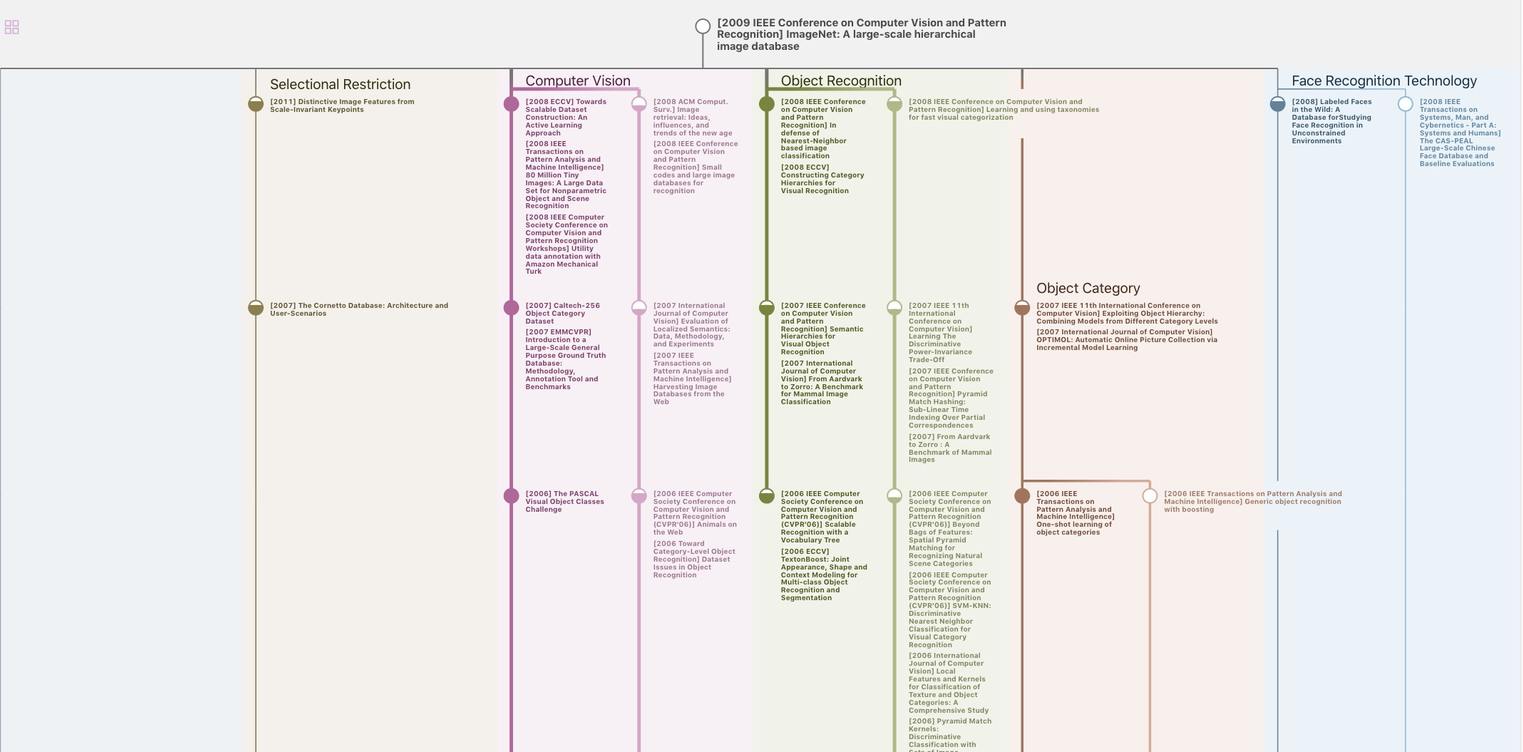
生成溯源树,研究论文发展脉络
Chat Paper
正在生成论文摘要