Catenary Insulator Defects Detection: A Dataset and an Unsupervised Baseline
IEEE Transactions on Instrumentation and Measurement(2024)
摘要
The high-speed railway, powered by the catenary, serves as a high-capacity and high-frequency transportation means due to its convenience. Insulators are integral components of the catenary. Once defects appear in insulators, they pose a risk of serious traffic accidents. Automatic detection of insulator defects proves to be an effective way to avoid further breakdowns and ensure the safety of the high-speed railway. To this end, many deep learning-based approaches have been developed. Though high performances have been achieved, they heavily rely on a large amount of high-quality annotated samples. Nevertheless, in practical scenarios, defect samples are difficult to collect. Moreover, annotating these samples is both labor-intensive and expert-requiring. Additionally, to the best of our knowledge, there is currently no publicly available dataset specifically dedicated to insulator defect detection. To address the issue of data scarcity, in this work, we first collect catenary insulator images captured by the high-speed rail inspection device on real railway lines and construct the Catenary Insulator Defect (CID) dataset. To break the dilemma of the lack of defect data and high-quality annotations, we further propose a simple but effective insulator defect detection framework in an unsupervised image reconstruction manner. Extensive experiments demonstrate that our proposed method demonstrates high accuracy in detecting various insulator defects without the need for manual annotations. The CID dataset and source codes have been made publicly available at https://github.com/LightZH/Insulator-Defect-Detection.
更多查看译文
关键词
Catenary Insulator,Defect Detection,Anomaly Detection,Image Reconstruction,Unsupervised Learning
AI 理解论文
溯源树
样例
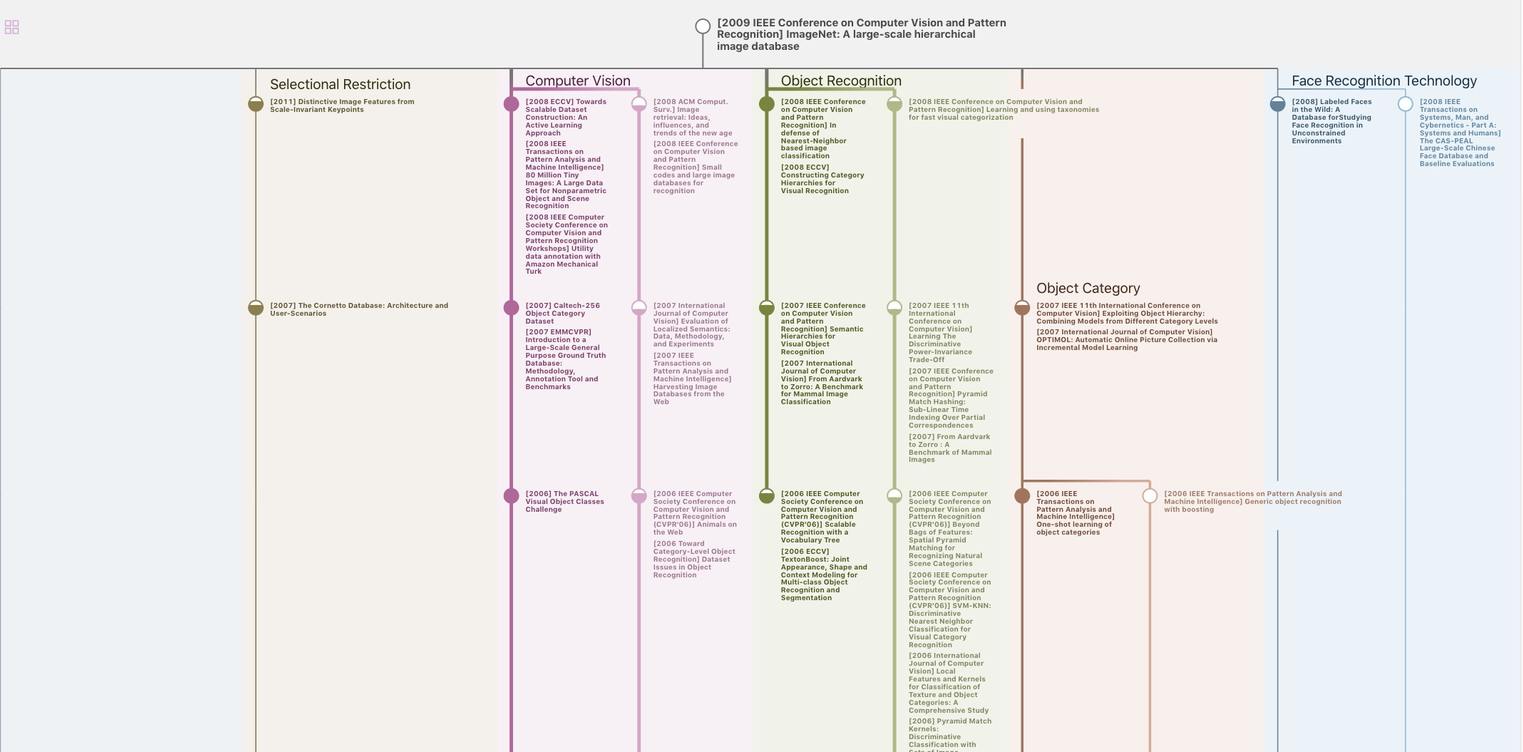
生成溯源树,研究论文发展脉络
Chat Paper
正在生成论文摘要