Optimization-Based Multi-Source Transfer Learning for Modeling of Nonlinear Processes
Chemical Engineering Science(2024)
摘要
This work develops an optimization-based multi-source transfer learning scheme for modeling of nonlinear chemical processes. Specifically, a transfer learning neural network model for a target process with limited data is developed using the pre-trained model obtained with multiple source processes. Since the performance of transfer learning models depends on the quality of the pre-trained models, we propose a novel Bayesian optimization problem to optimize the selection of multi-source data for the pre-trained models by first deriving a generalization error bound for multi-source domain adaptation using Y-discrepancy distance. Subsequently, the optimization problem is formulated using the theoretical error bound to select the optimal set of multiple sources, which can provide a good initial guess of the weight parameters for transfer learning model. Finally, a simulation study of a chemical reactor process in Aspen Plus Dynamics is conducted to illustrate the effectiveness of the optimization-based multi-source transfer learning scheme.
更多查看译文
关键词
Transfer learning,Nonlinear processes,Multi-source data,Bayesian optimization,Recurrent neural network,Process modeling
AI 理解论文
溯源树
样例
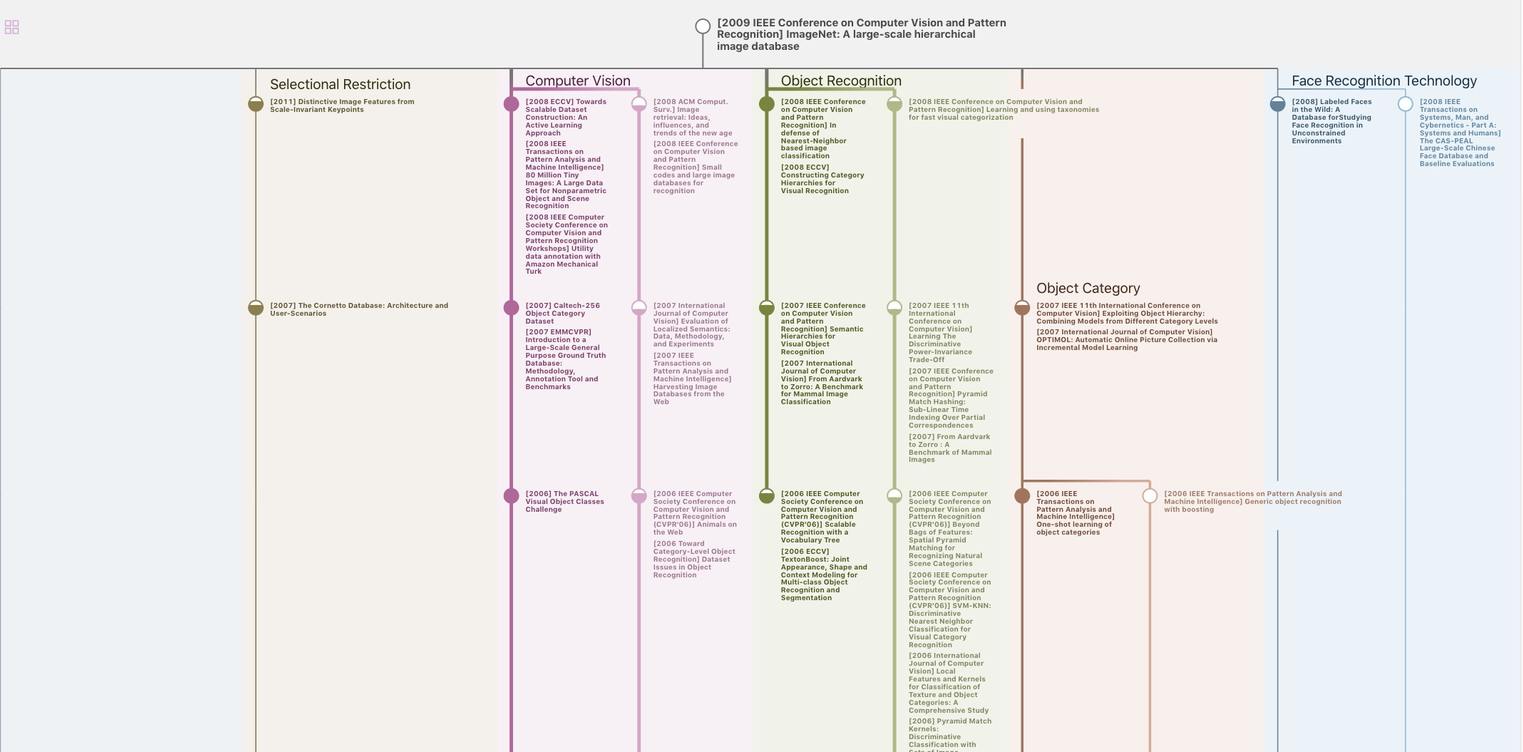
生成溯源树,研究论文发展脉络
Chat Paper
正在生成论文摘要