Automated assembly quality inspection by deep learning with 2D and 3D synthetic CAD data
Journal of Intelligent Manufacturing(2024)
摘要
In the manufacturing industry, automatic quality inspections can lead to improved product quality and productivity. Deep learning-based computer vision technologies, with their superior performance in many applications, can be a possible solution for automatic quality inspections. However, collecting a large amount of annotated training data for deep learning is expensive and time-consuming, especially for processes involving various products and human activities such as assembly. To address this challenge, we propose a method for automated assembly quality inspection using synthetic data generated from computer-aided design (CAD) models. The method involves two steps: automatic data generation and model implementation. In the first step, we generate synthetic data in two formats: two-dimensional (2D) images and three-dimensional (3D) point clouds. In the second step, we apply different state-of-the-art deep learning approaches to the data for quality inspection, including unsupervised domain adaptation, i.e., a method of adapting models across different data distributions, and transfer learning, which transfers knowledge between related tasks. We evaluate the methods in a case study of pedal car front-wheel assembly quality inspection to identify the possible optimal approach for assembly quality inspection. Our results show that the method using Transfer Learning on 2D synthetic images achieves superior performance compared with others. Specifically, it attained 95
更多查看译文
关键词
Assembly quality inspection,Synthetic data,Computer vision,Unsupervised domain adaptation,Transfer learning,Point cloud
AI 理解论文
溯源树
样例
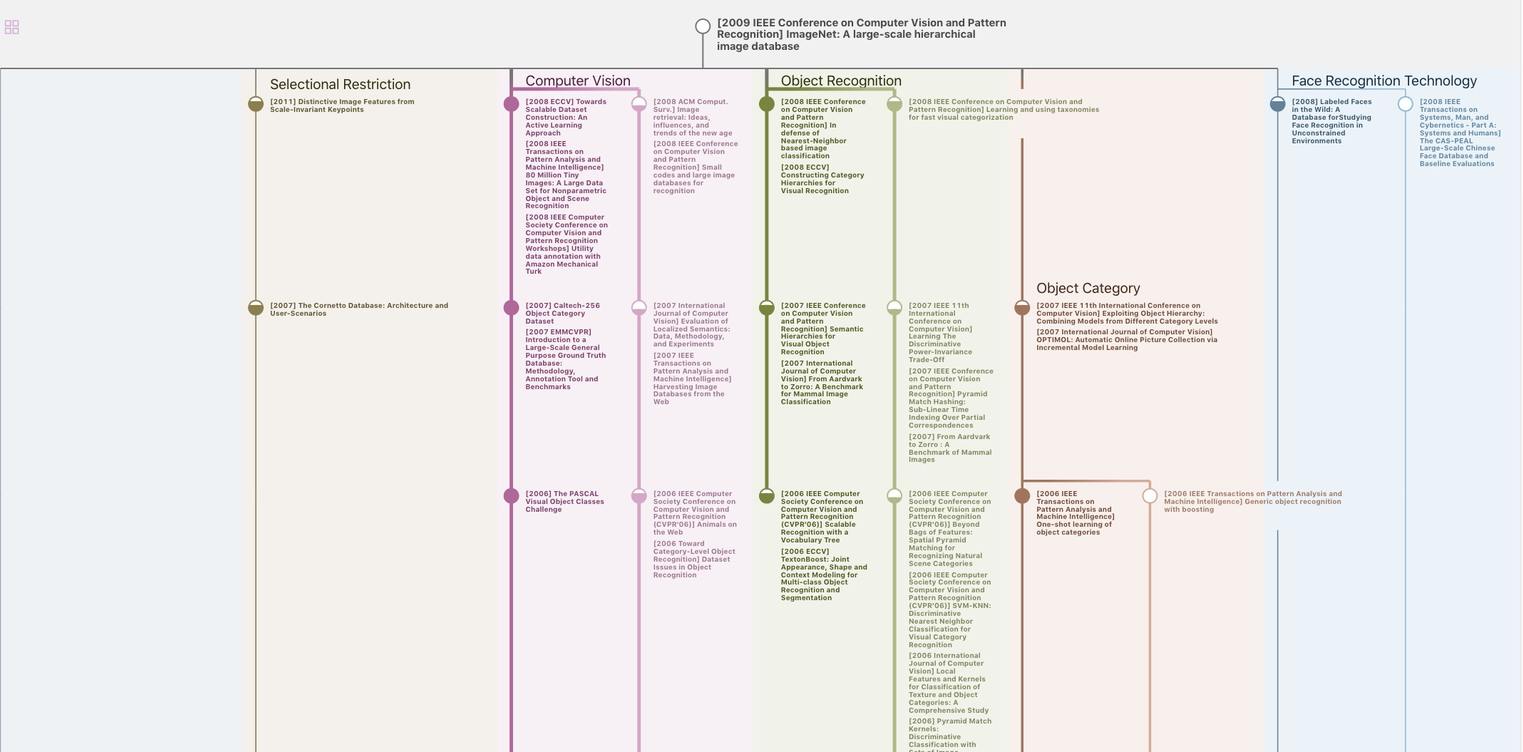
生成溯源树,研究论文发展脉络
Chat Paper
正在生成论文摘要