Neural Adaptive Video Streaming with OfflineReinforcement Learning
crossref(2024)
摘要
Abstract Learning adaptive bitrate (ABR) algorithms arecurrently an effective means for video players to optimize userquality of experience (QoE) under diverse network conditions.Nonetheless, reinforcement learning (RL) approaches demandextensive trial-and-error learning with Internet adaptive videostreaming, and the dynamic and heavy-tailed nature of networkcharacteristics poses a challenge. As a result, off-the-shelf RLtechniques face difficulties in efficient learning and fast adaptation to diverse network conditions. In this work, we propose Offline Meta-RL ABR (OMA) algorithm, which utilizes offline datasets to automatically generatehighly-efficient meta-ABR policies based on specific networkconditions. First, traditional learned ABR algorithm techniquesrequire lengthy online meta-training from video streaming sessions, which we replace with demonstration and and offline data,eliminating the need for expensive online learning and enablingsafer exploration. Second, meta-ABR inevitably fail to generalizeto unseen network conditions that differ significantly duringmeta-training. We address this issue by incorporating contextualmeta-learning for online fine-tuning. If the new network conditions are similar to the prior data, then the contextual meta-ABRlearner adapts immediately, and if it’s significantly different, itgradually adapts through fine-tuning. Comparing OMA under different network conditions, the experimental results demonstrate that it outperforms existing stateof-the-art ABR algorithms. OMA achieves up to 8× improvementduring training and effectively generalizes to unseen networkconditions and video streams.
更多查看译文
AI 理解论文
溯源树
样例
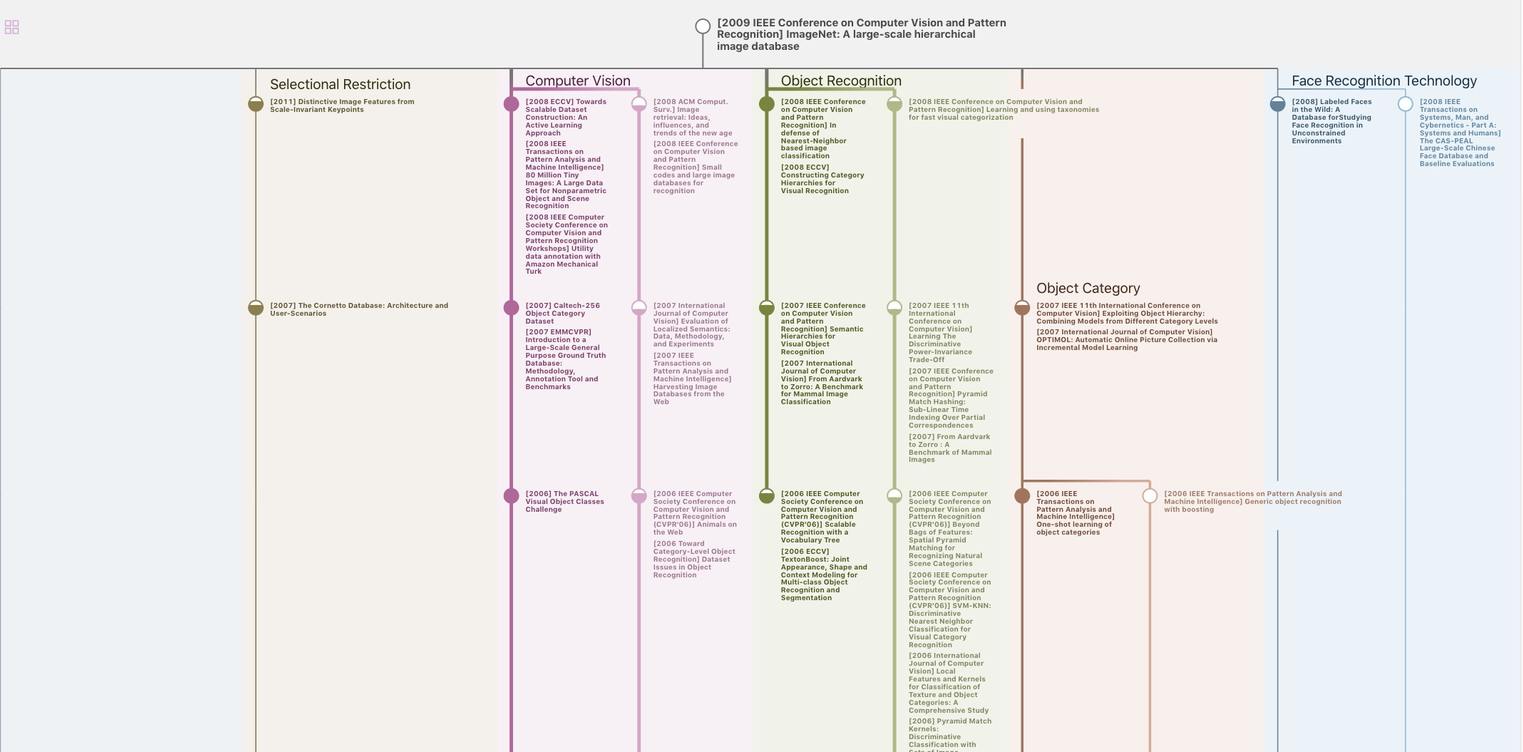
生成溯源树,研究论文发展脉络
Chat Paper
正在生成论文摘要