Sub-region aware retrieval-based network with multimodal prior knowledge guidance for microvascular invasion prediction in PET/CT imaging
Neural Computing and Applications(2024)
摘要
Accurate preoperative microvascular invasion (MVI) prediction using PET/CT imaging is important for guiding surgical plans and treatment strategies for hepatocellular carcinoma (HCC) patients. Deep learning (DL)-based approaches have shown effectiveness in medical imaging-based diagnostics. However, most DL-based methods for automatic MVI diagnosis depend on detailed annotations of HCC regions by clinicians, then perform feature extraction and directly output MVI risk scores, which can increase application complexity and lack transparency in decision-making. Therefore, we propose the sub-region aware retrieval-based network (SRANet), utilizing the prior knowledge of modality complementarity and localization of HCC in PET/CT images for tumor area location and feature extraction. Specifically, in the feature extraction stage, to better highlight lesion areas, we designed the prior knowledge guided fusion (PKGF) block. PKGF integrates knowledge from PET and CT through attention mechanism-based intermodal interaction and implicitly weights features to calibrate different modalities. Furthermore, we developed a sub-region aware module (SRAM) that employs class activation schemes and peak selection to identify and highlight sparse, salient sub-regions in tumor areas, relying solely on coarse-grained supervision. Additionally, we design a new loopback Siamese training strategy, guiding the model to focus on lesion areas through the semantic invariance of lesion features. Finally, we employ a retrieval strategy to present similar slices, providing clinicians with transparent references and enhancing the understandability of MVI predictions beyond simple risk scores. Experimental results show SRANet outperforms leading methods, achieving a slice-level AUC of 0.855 and an individual-level AUC of 0.796, highlighting its potential for clinical application.
更多查看译文
关键词
Computer-aided cancer diagnosis,Medical image retrieval,Multimodal
AI 理解论文
溯源树
样例
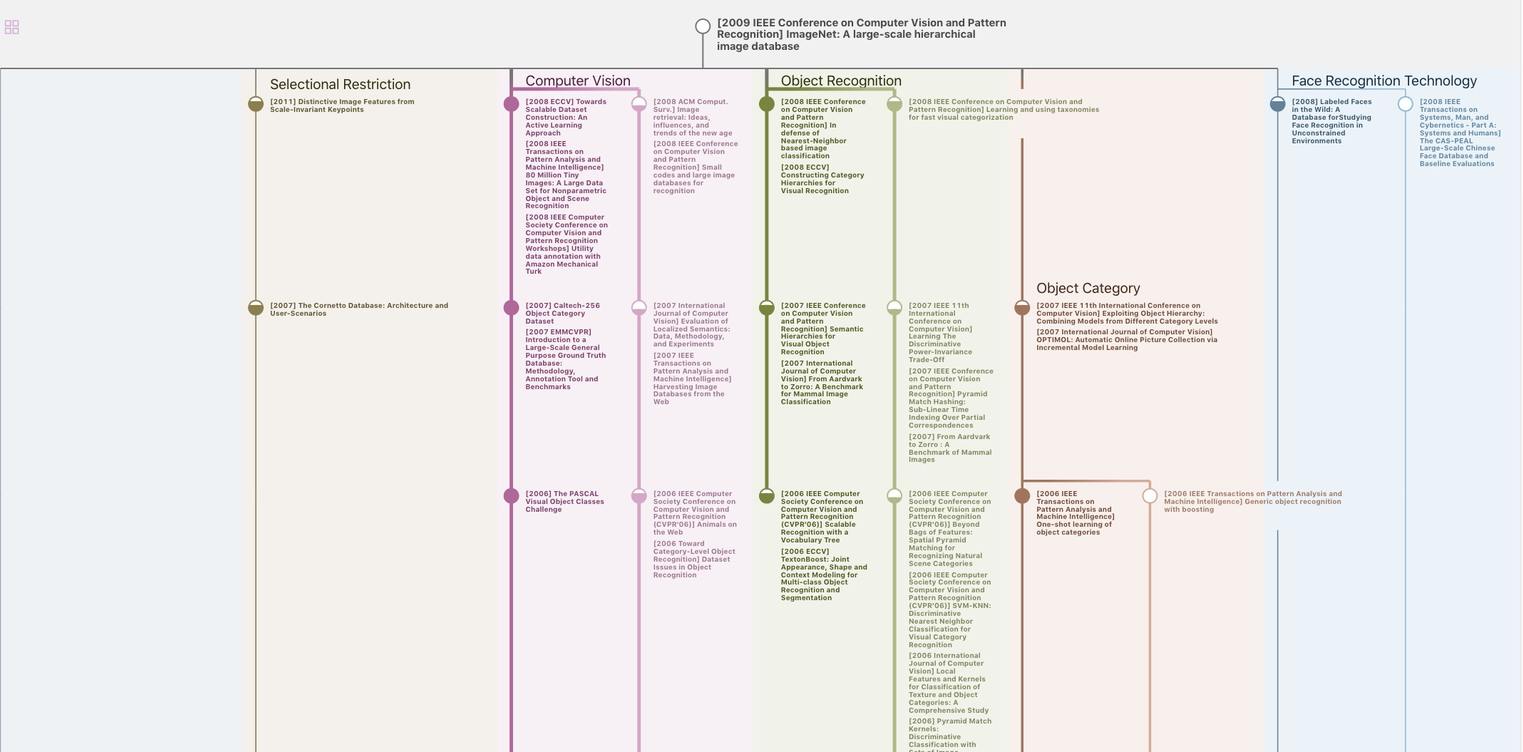
生成溯源树,研究论文发展脉络
Chat Paper
正在生成论文摘要