An Early Warning Approach for Pilots’ Cognitive Tipping Points Based Multi-Modal Signals
IEEE Transactions on Automation Science and Engineering(2024)
摘要
When executing complex missions in emergency scenarios, pilots’ cognitive state may deteriorate, posing significant challenges to flight safety and mission execution. This paper proposes a cross-subject and cross-session early warning approach based on small-sample and multi-modal signals for predicting cognitive collapse state. We designs an experimental paradigm that has been demonstrated to induce cognitive collapse in 87
$\%$
of trials by analyzing questionnaire scores, physiological signals, and task performance. The extracted multi-modal features are fused and selected to construct the optimal feature set. Further, a two-step early warning method is introduced to identify critical slowing down and to predict tipping points by detecting the high cognitive workload state and classifying the corresponding signals into early warning signal (EWS) and normal state. The early warning of pilot’s cognitive tipping point obtains the result that recall is 77.76
$\%$
, false alarm rate is 28.43
$\%$
, and the AUC of the warning method can reach 0.83. Our method shows better early warning performance compared with other classification models and has strong generalizability on other datasets.
Note to Practitioners
—The pilots’ cognitive state is crucial for mission completion and flight safety. Previous studies mainly focused on assessing current cognitive state, and there is little research on early warning of cognitive collapse that occurs in emergency scenarios (e.g., faults of system and the sudden increase in flight missions). In particular, identification is not effective for small-sample and instable data. Therefore, this paper presents an early warning method for cognitive tipping points, which can provide timely warning of pilots’ cognitive collapse and reduce human-caused risks in emergency scenarios.
更多查看译文
关键词
Early warning of tipping point,cognitive workload,transfer learning,multi-modal physiological signals,flight safety in emergency scenarios
AI 理解论文
溯源树
样例
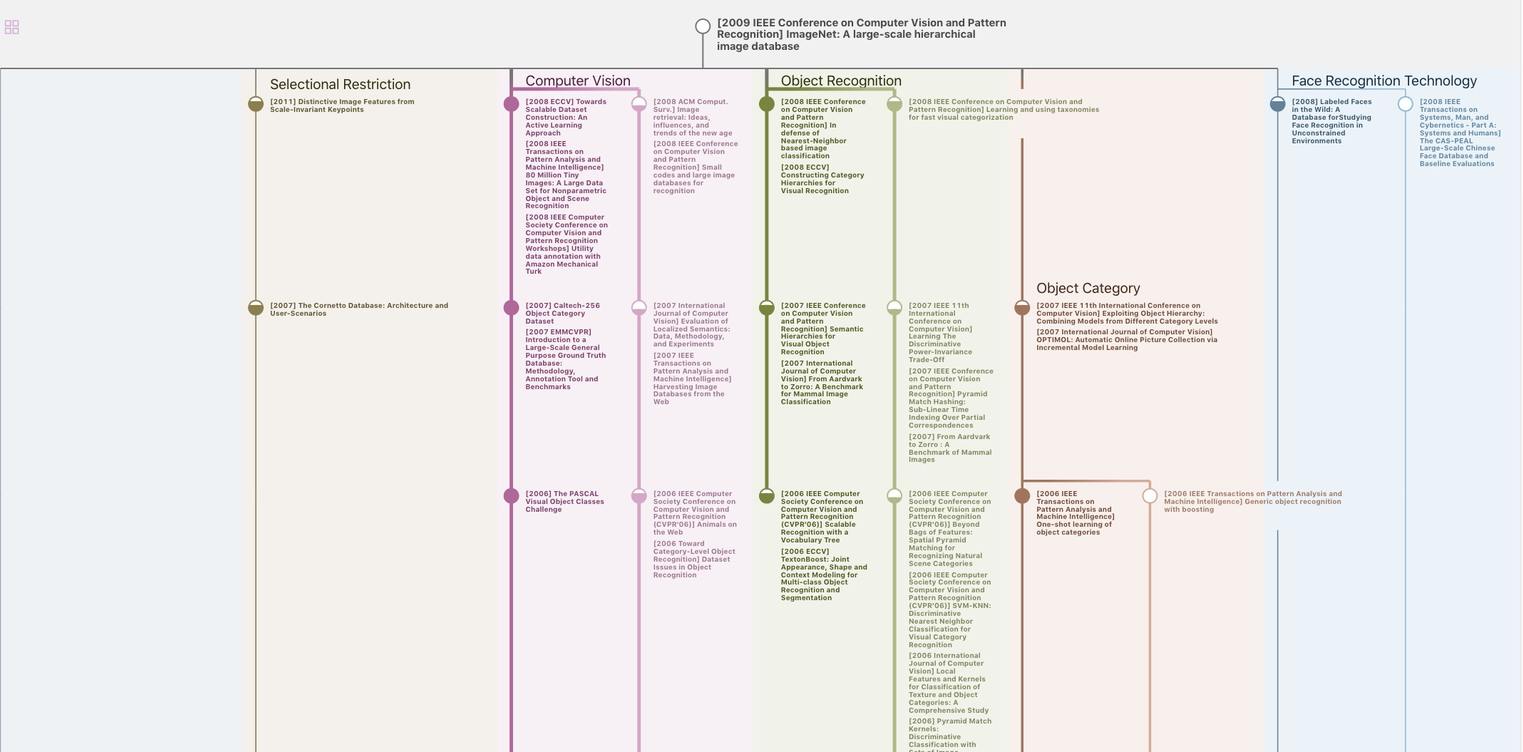
生成溯源树,研究论文发展脉络
Chat Paper
正在生成论文摘要