Multimodal Fusion of Echocardiography and Electronic Health Records for the Detection of Cardiac Amyloidosis
arxiv(2024)
摘要
Cardiac amyloidosis, a rare and highly morbid condition, presents significant
challenges for detection through echocardiography. Recently, there has been a
surge in proposing machine-learning algorithms to identify cardiac amyloidosis,
with the majority being imaging-based deep-learning approaches that require
extensive data. In this study, we introduce a novel transformer-based
multimodal fusion algorithm that leverages information from both imaging and
electronic health records. Specifically, our approach utilizes echocardiography
videos from both the parasternal long-axis (PLAX) view and the apical 4-chamber
(A4C) view along with patients' demographic data, laboratory tests, and cardiac
metrics to predict the probability of cardiac amyloidosis. We evaluated our
method using 5-fold cross-validation on a dataset comprising 41 patients and
achieved an Area Under the Receiver Operating Characteristic curve (AUROC) of
0.94. The experimental results demonstrate that our approach can achieve
competitive results with a significantly smaller dataset compared to prior
imaging-based methods that required data from thousands of patients. This
underscores the potential of leveraging multimodal data to enhance diagnostic
accuracy in the identification of complex cardiac conditions such as cardiac
amyloidosis.
更多查看译文
AI 理解论文
溯源树
样例
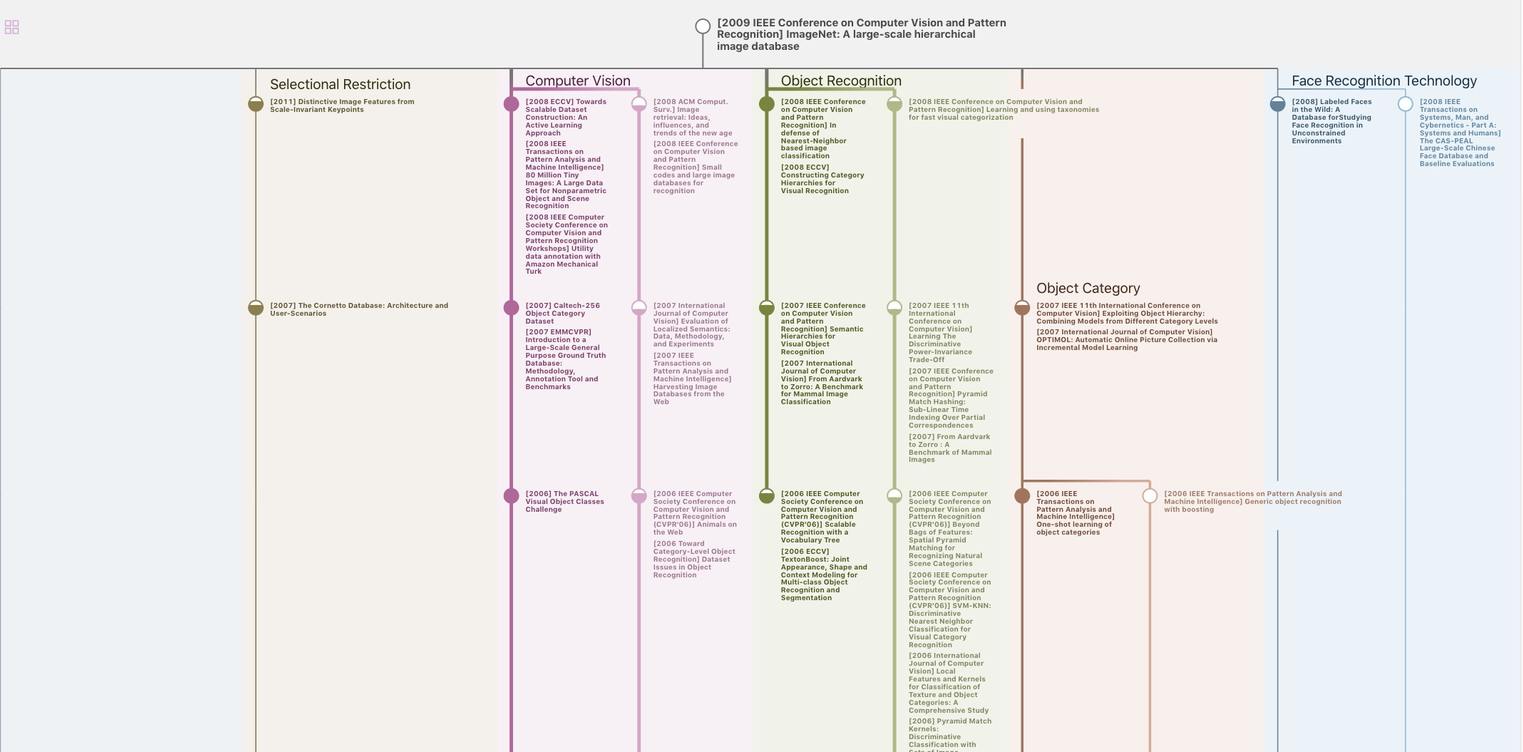
生成溯源树,研究论文发展脉络
Chat Paper
正在生成论文摘要